Attention-Enhanced Dual-Branch Residual Network with Adaptive L-Softmax Loss for Specific Emitter Identification under Low-Signal-to-Noise Ratio Conditions
Remote Sensing(2024)
摘要
To address the issue associated with poor accuracy rates for specific emitter identification (SEI) under low signal-to-noise ratio (SNR) conditions, where the single-dimension radar signal characteristics are severely affected by noise, we propose an attention-enhanced dual-branch residual network structure based on the adaptive large-margin Softmax (ALS). Initially, we designed a dual-branch network structure to extract features from one-dimensional intermediate frequency data and two-dimensional time–frequency images, respectively. By assigning different attention weights according to their importance, these features are fused into an enhanced joint feature for further training. This approach enables the model to extract distinctive features across multiple dimensions and achieve good recognition performance even when the signal is affected by noise. In addition, we have introduced L-Softmax to replace the original Softmax and propose the ALS. This approach adaptively calculates the classification margin decision parameter based on the angle between samples and the classification boundary and adjusts the margin values of the sample classification boundaries; it reduces the intra-class distance for the same class while increasing the inter-class distance between different classes without the need for cumbersome experiments to determine the optimal value of decision parameters. Our experimental findings revealed that, in comparison to alternative methods, our proposed approach markedly enhances the model’s capability to extract features from signals and classify them in low-SNR environments, thereby effectively diminishing the influence of noise. Notably, it achieves the highest recognition rate across a range of low-SNR conditions, registering an average increase in recognition rate of 4.8%.
更多查看译文
关键词
specific emitter identification,residual network,feature fusion,attention mechanism,adaptive large-margin Softmax
AI 理解论文
溯源树
样例
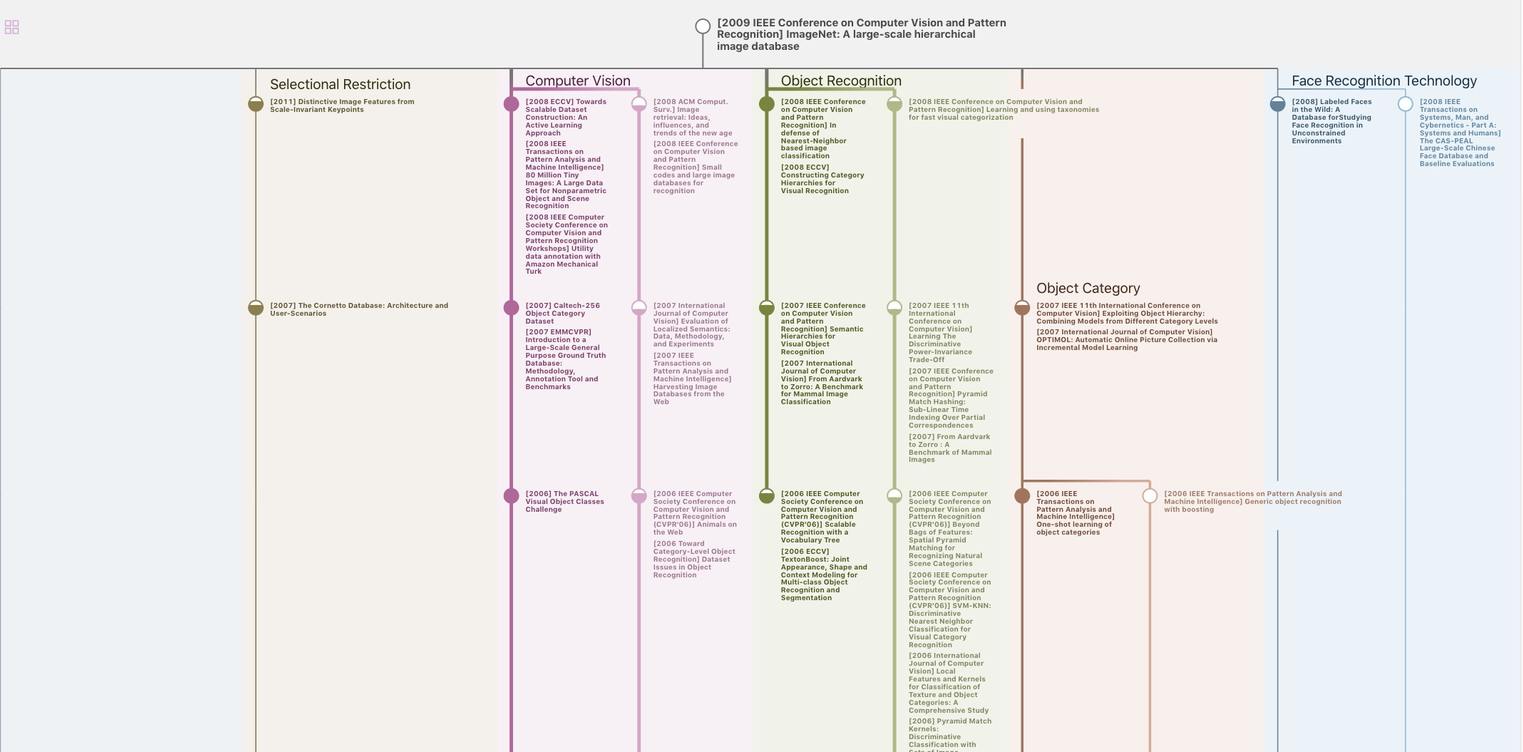
生成溯源树,研究论文发展脉络
Chat Paper
正在生成论文摘要