A Contactless Breathing Pattern Recognition System Using Deep Learning and WiFi Signal
IEEE Internet of Things Journal(2024)
摘要
Breathing pattern is a representation of human breathing in the rate, depth, and rhythm, which can reflect physical and mental health conditions. Capturing and identifying abnormal breathing patterns can help localize associated disorders and have important implications for the patient or the potential patient. In this paper, a breathing patterns recognition system is proposed to monitor and identify abnormal breathing patterns in a contactless, unobtrusive and comfortable way. The system utilize the designed prototype based on WiFi signal and deep learning architecture to achieve the reliable measurement and recognition of respiratory patterns. We first develop a series of data preprocessing method to capture accurately the time-domain breathing signal from received data. Then, we apply a combined convolutional–long short-term memory (CNN-LSTM) network model to classify six distinct respiratory patterns (Eupnea, Tachypnea, Bradypnea, Biots, Cheyne–Stokes, and Kussmaul). The experimental results demonstrate that the proposed system have the ability to effectively classify the afore-mentioned six breathing patterns, which combines a series of novel data processing methods with the obtained CNN-LSTM model. The accuracy, precision, recall and F1-scores obtained by the CNN-LSTM model on the collected test set were 97.8%, 97.9%, 97.8% and 97.8%, respectively. In addition, the proposed system achieved 96.7%, 97.5%, and 98.1% recognition accuracy in different indoor environments. Overall, the proposed contactless breathing patterns recognition system validates the feasibility of long-term continuous respiratory patterns recognition, and provides a potential solution for the auxiliary diagnosis of diseases.
更多查看译文
关键词
Breathing pattern,contactless respiration monitoring,deep neural network,WiFi
AI 理解论文
溯源树
样例
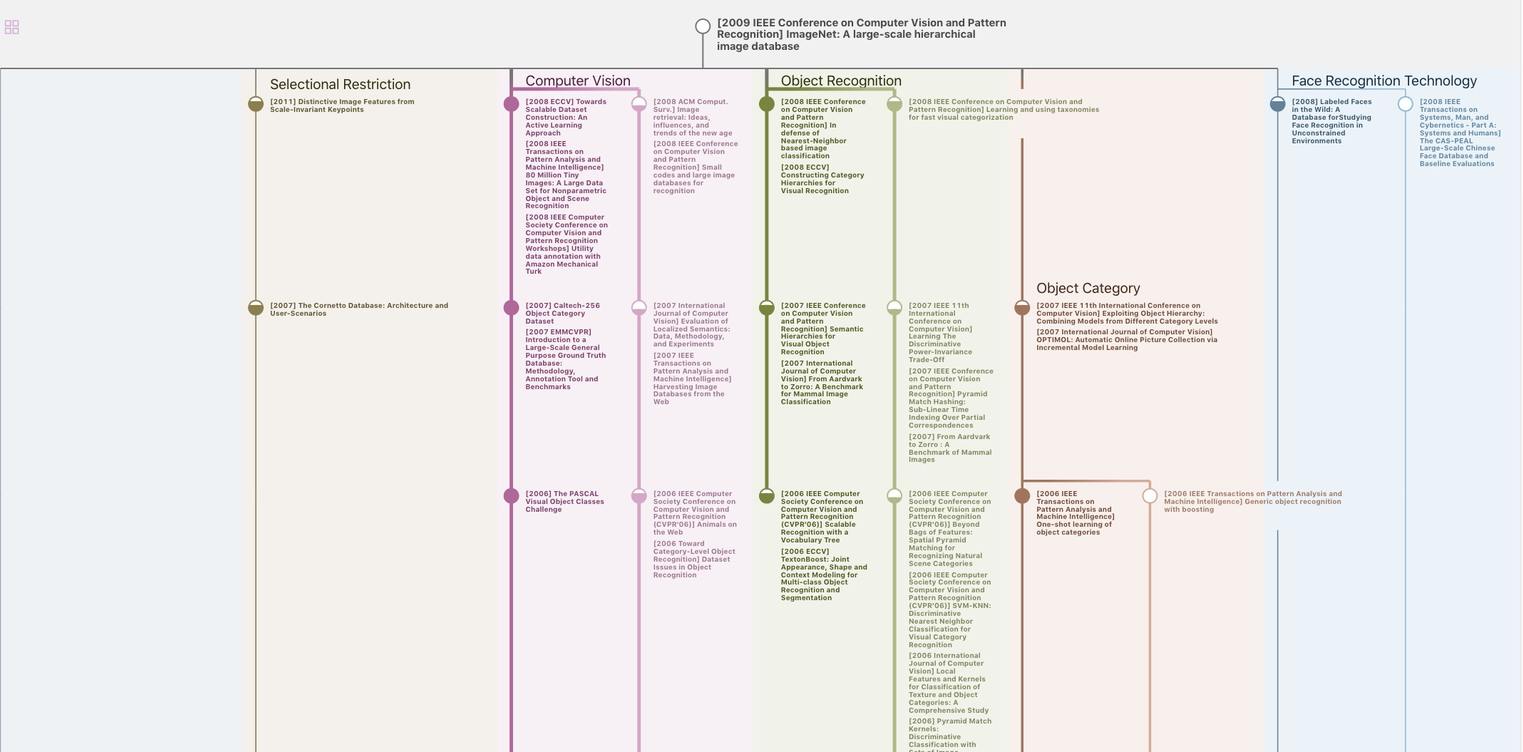
生成溯源树,研究论文发展脉络
Chat Paper
正在生成论文摘要