Leveraging Self-Distillation and Disentanglement Network to Enhance Visual–Semantic Feature Consistency in Generalized Zero-Shot Learning
Electronics(2024)
摘要
Generalized zero-shot learning (GZSL) aims to simultaneously recognize both seen classes and unseen classes by training only on seen class samples and auxiliary semantic descriptions. Recent state-of-the-art methods infer unseen classes based on semantic information or synthesize unseen classes using generative models based on semantic information, all of which rely on the correct alignment of visual–semantic features. However, they often overlook the inconsistency between original visual features and semantic attributes. Additionally, due to the existence of cross-modal dataset biases, the visual features extracted and synthesized by the model may also mismatch with some semantic features, which could hinder the model from properly aligning visual–semantic features. To address this issue, this paper proposes a GZSL framework that enhances the consistency of visual–semantic features using a self-distillation and disentanglement network (SDDN). The aim is to utilize the self-distillation and disentanglement network to obtain semantically consistent refined visual features and non-redundant semantic features to enhance the consistency of visual–semantic features. Firstly, SDDN utilizes self-distillation technology to refine the extracted and synthesized visual features of the model. Subsequently, the visual–semantic features are then disentangled and aligned using a disentanglement network to enhance the consistency of the visual–semantic features. Finally, the consistent visual–semantic features are fused to jointly train a GZSL classifier. Extensive experiments demonstrate that the proposed method achieves more competitive results on four challenging benchmark datasets (AWA2, CUB, FLO, and SUN).
更多查看译文
关键词
generalized zero-shot learning,self-distillation,disentanglement network,visual–semantic feature consistency
AI 理解论文
溯源树
样例
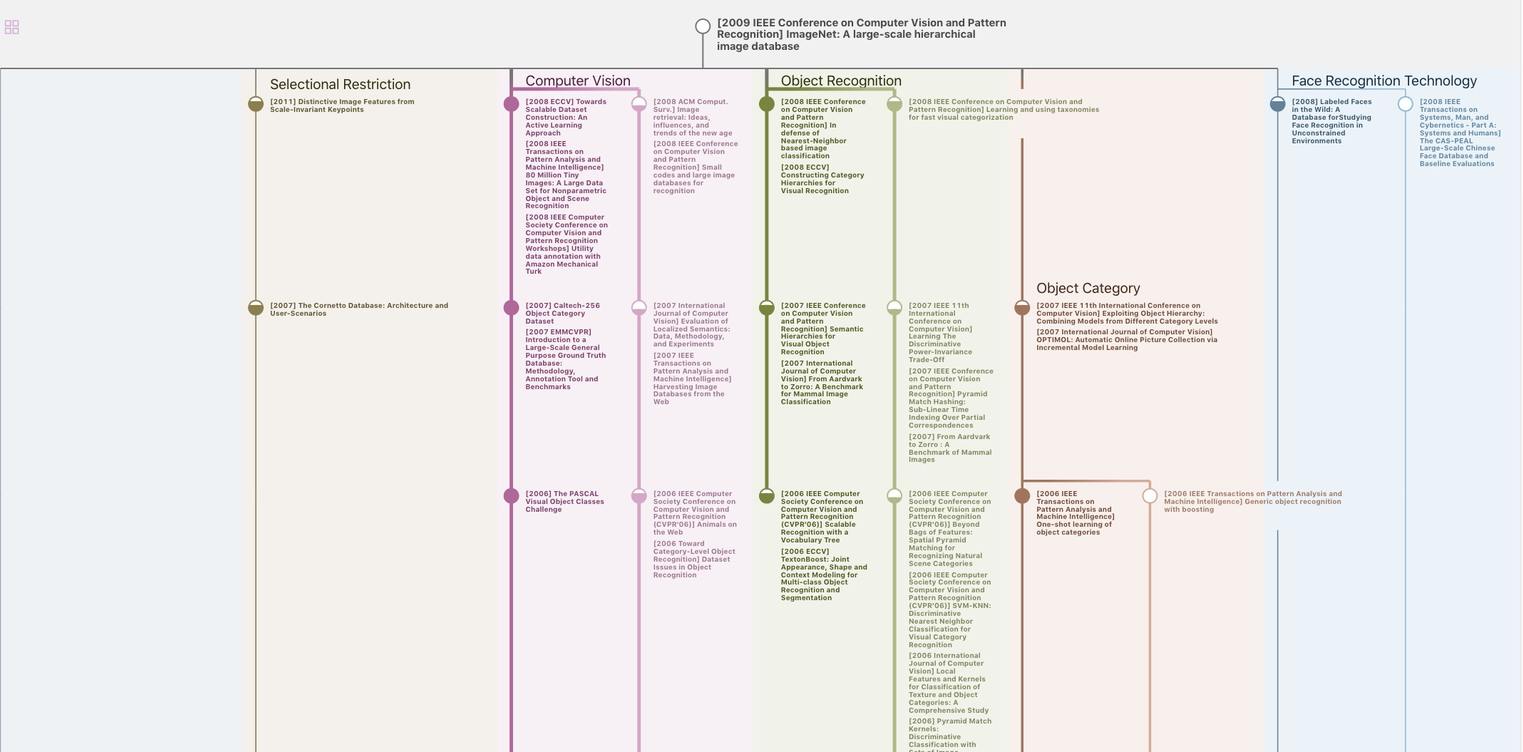
生成溯源树,研究论文发展脉络
Chat Paper
正在生成论文摘要