An Adaptive Exponentially Weighted Moving Average Based Support Vector Data Description Machine for Multivariate Process
crossref(2024)
摘要
Abstract In recent years, modern manufacturing systems have become increasingly complex and generate a wide variety of data. This poses challenges for finding patterns and effective monitoring processes. One-class classification (OCC) is a machine learning technique suitable for finding data patterns. OCC algorithms learn a model of normal process behavior and flag deviations. This helps to detect outliers and process shifts. Support Vector Data Description (SVDD), an OCC type, was used to monitor high-dimensional data. It constructs a hypersphere around normal data and flag outliers. However, SVDD is sensitive to volatile process shifts. Adaptive Exponentially Weighted Moving Average (AEWMA) control charts address this issue. They dynamically adjust the control limits based on the process-mean changes. This study proposes a new AEWMA-based SVDD control chart (D'AEWMA) for monitoring multivariate processes. The D'AEWMA chart outperformed the traditional D'EWMA chart in detecting out-of-control signals and minimizing false alarms.
更多查看译文
AI 理解论文
溯源树
样例
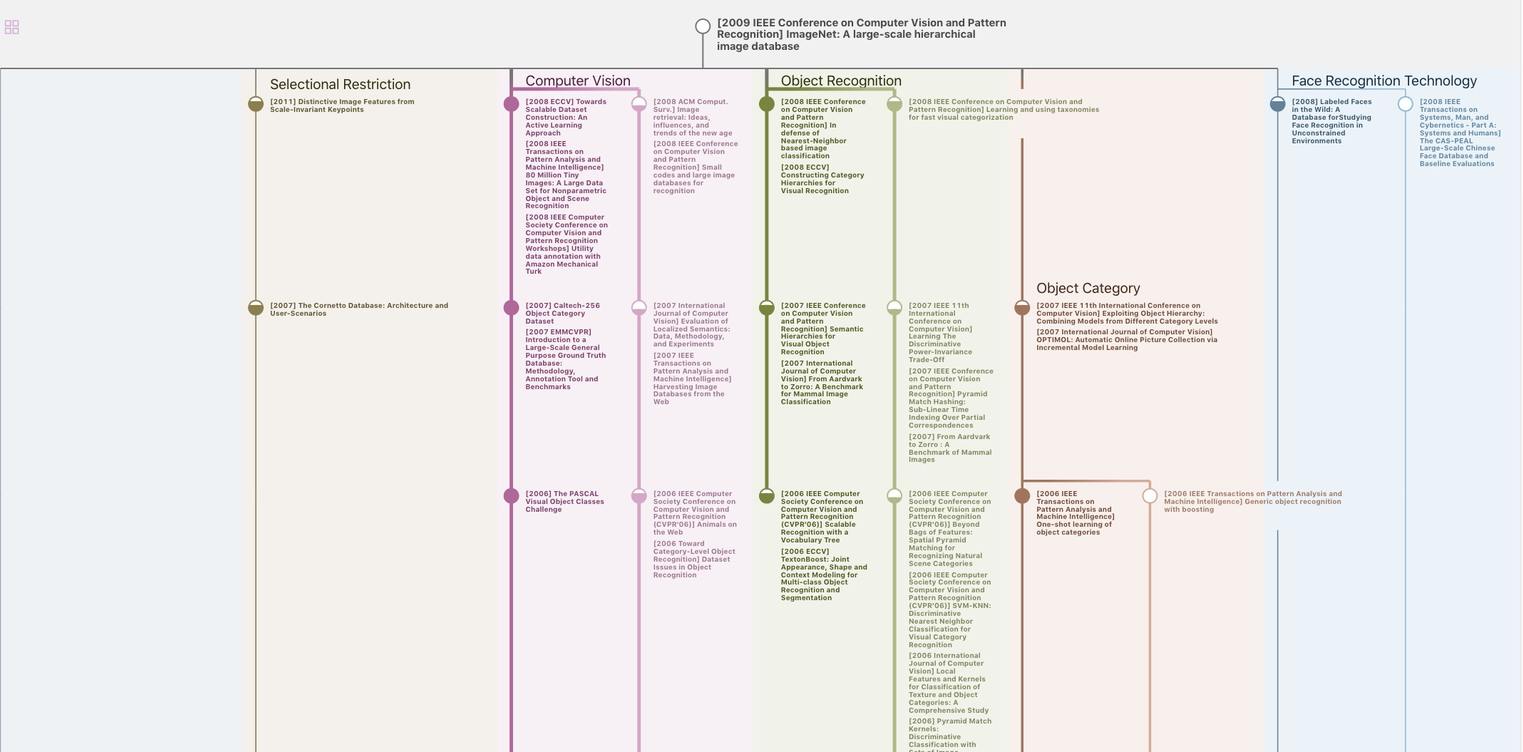
生成溯源树,研究论文发展脉络
Chat Paper
正在生成论文摘要