Text-to-Image Segmentation with Open-Vocabulary and Multitasking
crossref(2024)
摘要
Open-vocabulary learning has recently gained prominence as a means to enable image segmentation for arbitrary categories based on textual descriptions. This advancement has extended the applicability of segmentation systems to a broader range of generally purpose scenarios. However, current methods often revolve around specialized architectures and parameters tailored to specific segmentation tasks, resulting in a fragmented landscape of segmentation models. In response to these challenges, we introduce OVAMTSeg, a versatile framework designed for Open-Vocabulary and Multitask Image Segmentation. OVAMTSeg harnesses adaptive prompt learning to empower the model to capture category-sensitive concepts, enhancing its robustness across diverse multi-task and scenario contexts. Text prompts are employed to effectively capture semantic and contextual features of the text, while cross-attention and cross-modal interactions enable the fusion of image and text features. Furthermore, a transformer-based decoder is incorporated for dense prediction. Extensive experimental results underscore the effectiveness of OVAMTSeg, showcasing its state-of-the-art performance and superior generalization capabilities across three segmentation tasks. Notable achievements include a 47.5 mIoU in referring expression segmentation, 51.6 mIoU on Pascal-VOC with four unseen classes, 46.6 mIoU on Pascal-Context in zero-shot segmentation, 65.9 mIoU on Pascal-5i, and 35.7 mIoU on COCO-20i datasets for one-shot segmentation.
更多查看译文
AI 理解论文
溯源树
样例
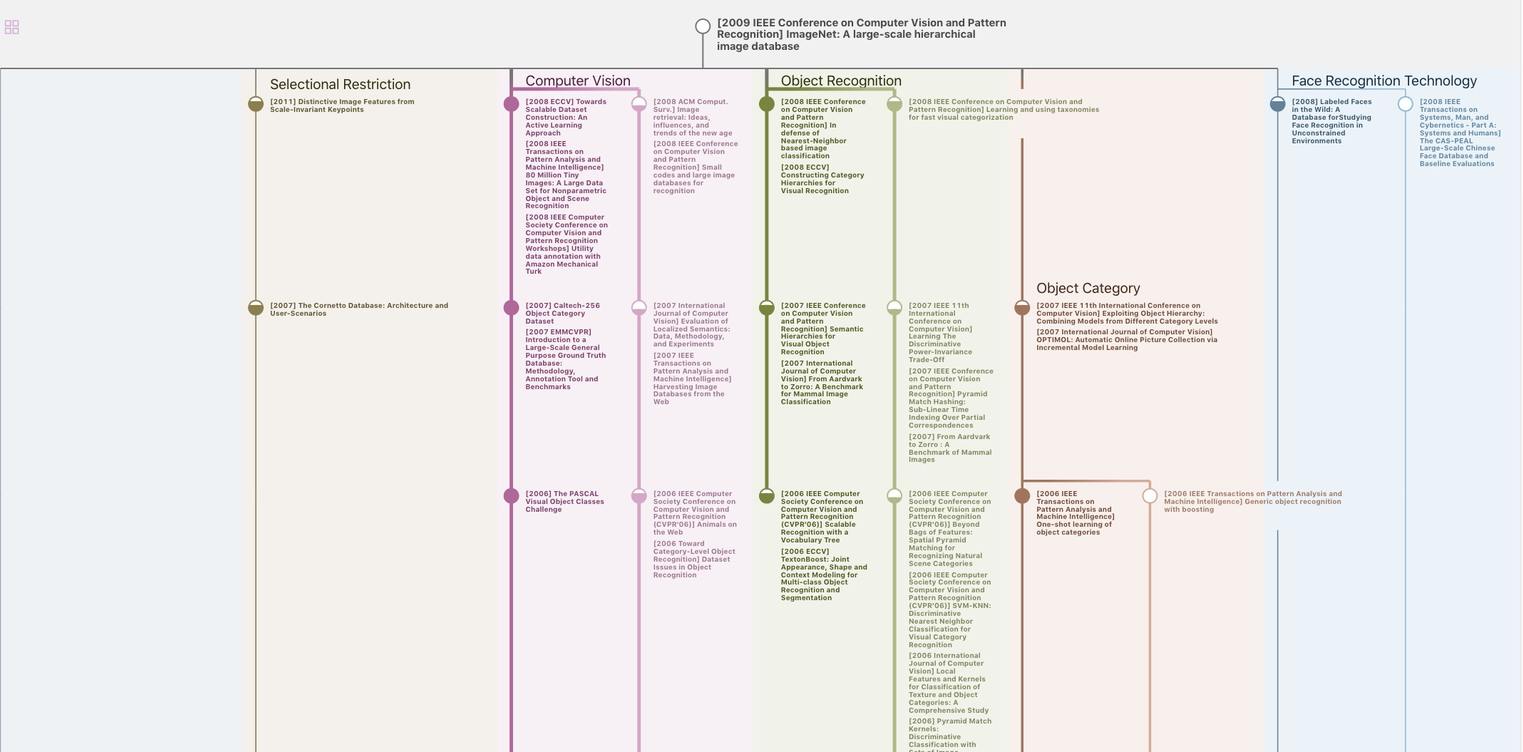
生成溯源树,研究论文发展脉络
Chat Paper
正在生成论文摘要