Pre-training enhanced unsupervised contrastive domain adaptation for industrial equipment remaining useful life prediction
Advanced Engineering Informatics(2024)
摘要
An essential task in industrial intelligence is to accurately predict the remaining useful life(RUL) of industrial equipment, and there has been tremendous progress in RUL prediction based on data-driven methods. However, these methods rely heavily on the data representation ability of the model and the assumption of consistency in data distribution. In practical industrial environments, due to different working conditions, industrial time series data exhibit high-dimensional, dynamic, and noisy characteristics, which often leads to ineffective transferability of trained models from one environment to similar yet unlabeled new environments. To tackle the aforementioned issues, this paper first designed a dual parallel time–frequency feature extraction network for extracting effective time-series features with different dimensions and importance levels. Afterwards, an enhanced pre-training framework is proposed that employs similarity contrast learning to unearth the latent representational information in industrial time-series data. Finally, a domain adaptation method based on momentum-contrast adversarial learning is proposed, which preserves the structural information specific to the target domain during adversarial learning domain-invariant features, mitigating the negative transfer effect. A series of rigorous experiments were conducted on two widely recognized industrial benchmark dataset, focusing on cross-domain scenarios. The results demonstrate that our approach achieves state-of-the-art performance in industrial cross-domain prediction scenarios.
更多查看译文
关键词
Domain adaptation,Contrastive learning,Industrial intelligence
AI 理解论文
溯源树
样例
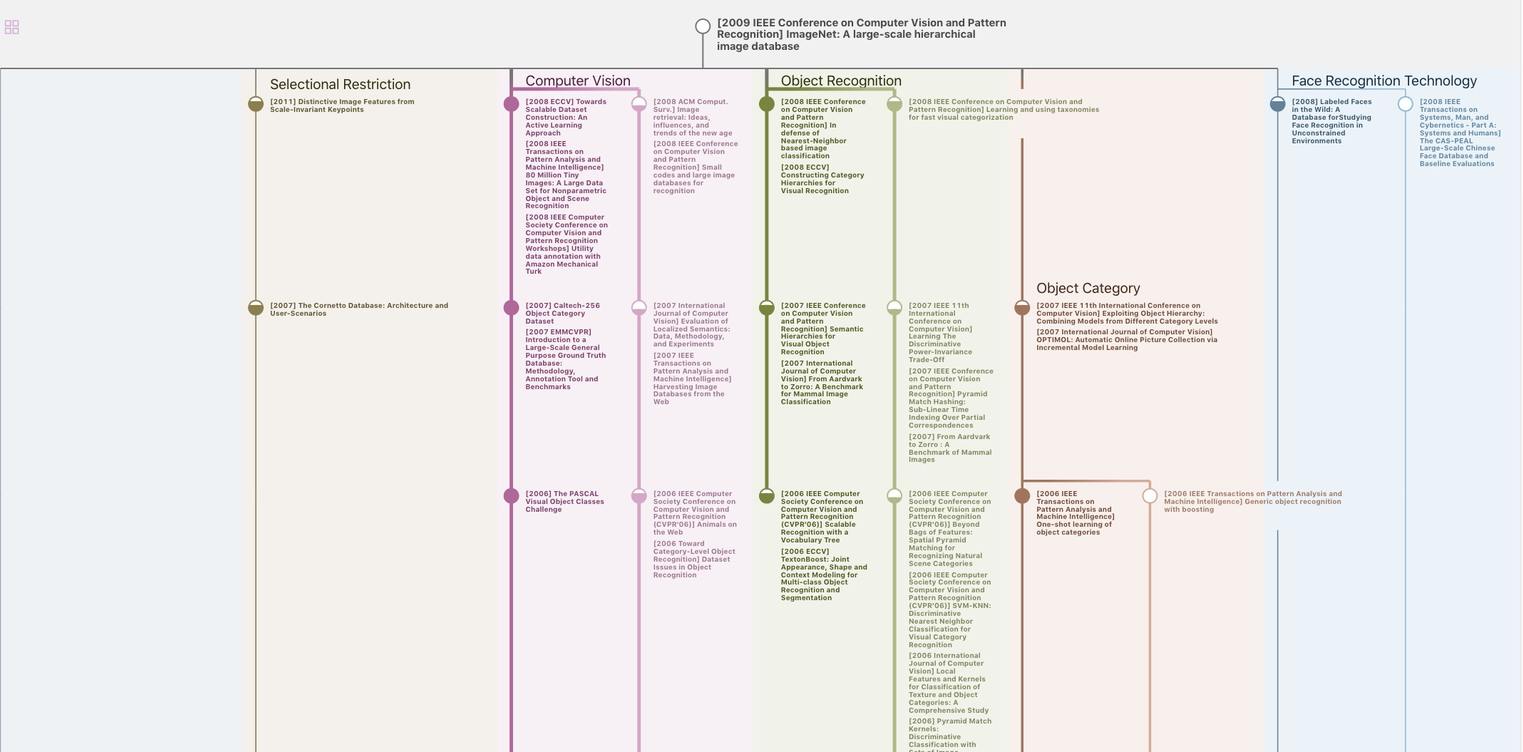
生成溯源树,研究论文发展脉络
Chat Paper
正在生成论文摘要