Improving Financial QA via Enhancing Argument Understanding with an Argument Predictor.
IEEE International Conference on Big Data and Smart Computing(2024)
摘要
This paper studies enhancing mathematical reasoning in Question Answering by improving the comprehension of arguments. In mathematical QA based on real-world documents, generating a reasoning program with correct arguments is challenging due to the many noisy inputs, unlike in general mathematical QA. In this study, we explore the potential for improving the performance of mathematical QA by enhancing the understanding and extraction of proper arguments to the Question. To this end, we propose an argument predictor to enhance the model's ability to comprehend and extract proper arguments for generating solution programs. Experiments conducted on the FinQA dataset demonstrate the improvement in the model's ability to identify relevant arguments, particularly in scenarios demanding multiple reasoning. The result shows improved program accuracy and execution accuracy, confirming the efficacy of our argument predictor.
更多查看译文
关键词
Question Answering,Numerical Reasoning,Mathematical Reasoning,Finance,Financial QA
AI 理解论文
溯源树
样例
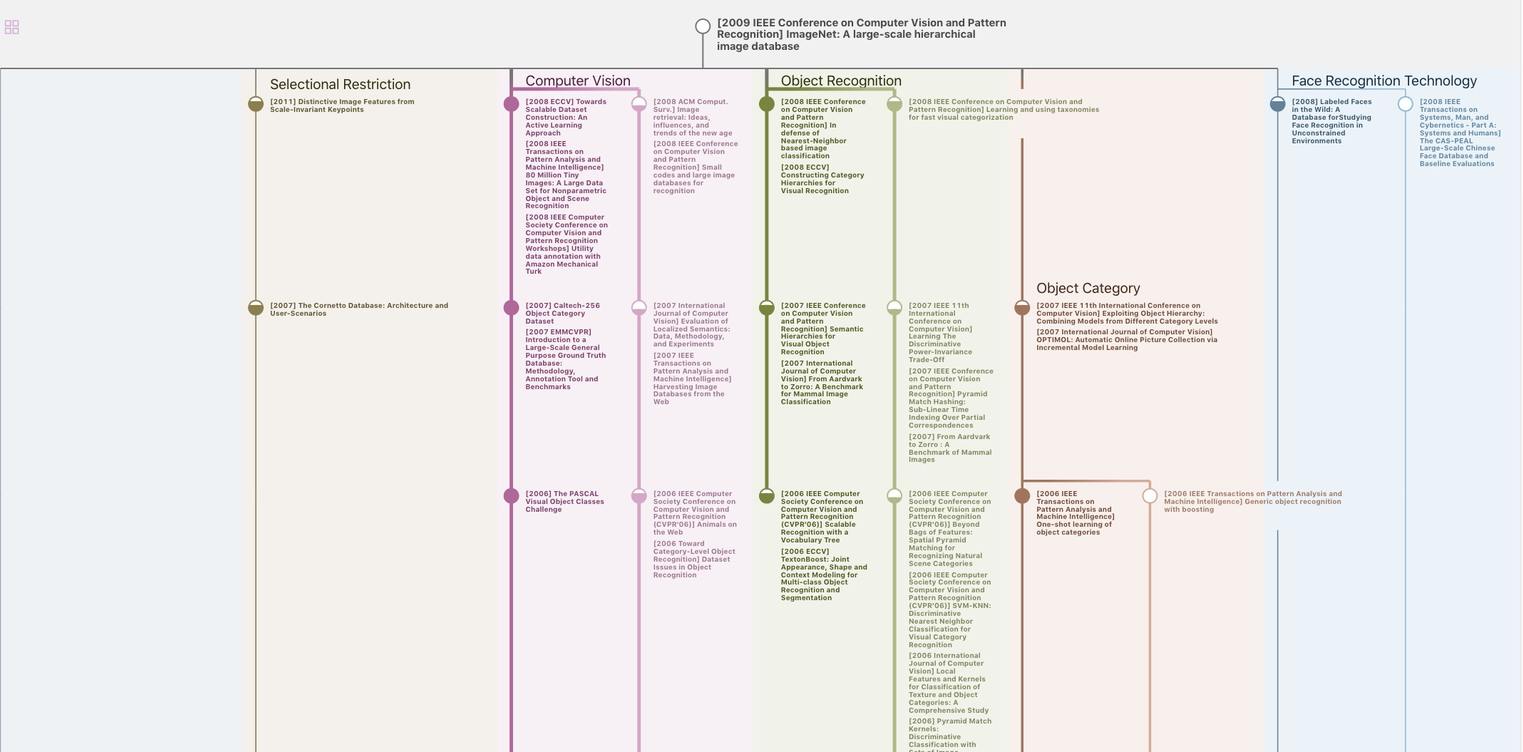
生成溯源树,研究论文发展脉络
Chat Paper
正在生成论文摘要