Machine Fault Diagnosis Using EMD-Gammatone Texture Representation and A Lightweight Self-Attention SqueezeNet.
IEEE International Conference on Big Data and Smart Computing(2024)
摘要
Machine fault diagnosis involves the intricate process of detecting and isolating faults, which is particularly challenging due to various sources of noise and the complex nature of the faults. In automatic fault diagnosis, feature engineering becomes daunting because of the time-varying characteristics of the fault signals. As artificial intelligence continues to advance, deep learning models have been increasingly employed for machine fault classification. However, a significant limitation of state-of-the-art models is their high computational complexity, making them unsuitable for deployment on portable devices. In this paper, a lightweight fault diagnosis model is proposed that consists of a self-attention SqueezeN et architecture along with a hybrid texture representation technique using empirical mode decomposition (EMD) and gammatone-spectrogram (GS) filter. In the model, initially, the dominant signal is extracted from the ID audio fault signals by discarding lower intrinsic mode functions (IMFs) from EMD and then the dominant signals are converted to 2D texture maps applying the GS filter. Then, the generated texture maps are fed to the modified self-attention SqueezeNet classifier, with reduced model width and depth as input for training and validation. In the experimental evaluation, two public benchmark datasets- ToyADMOS and MIMII are used to validate the model. Finally, the model classifies the machine audio faults using the trained features. The experimental results demonstrated that the hybrid EMD-Gammatone texture imaging outperforms the other state-of-the-art methods like MFCC, Gammatone, and Hilbert Huang Transform with a self-attention (SA) based SqueezeNet architecture. The EMD-Gammatone spectrum-based feature extraction accurately detected the faults by exhibiting an accuracy of 89.32% and 96.46% for MIMII and ToyADMOS datasets, respectively. In addition, with the EMD-Gammatone Spectrogram images, the proposed model outperforms the conventional SqueezeNet and other state-of-the-art deep architectures with comparatively higher Precision, Recall, and FI scores. Furthermore, the proposed model gains reduced computational complexity due to 93.4
%
fewer trainable parameters of SqueezeN et than the conventional SqueezeN et model and the attention mechanism that focuses on important regions or features of the input.
更多查看译文
关键词
Gammatone Spectrogram,Machine Fault Classification,Self-Attention SqueezeNet
AI 理解论文
溯源树
样例
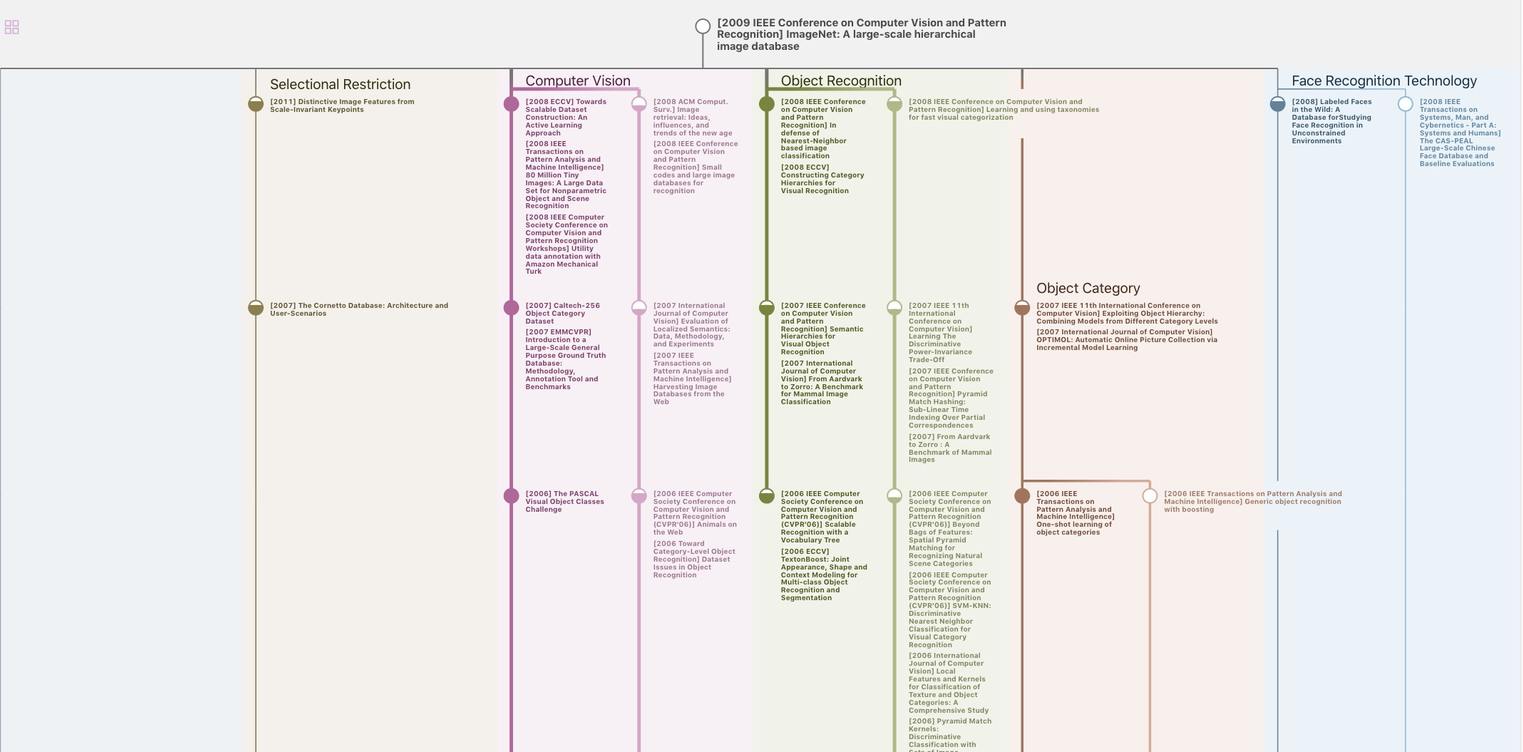
生成溯源树,研究论文发展脉络
Chat Paper
正在生成论文摘要