An enhanced structural developmental neural network with information saturation for continual unsupervised learning
Neurocomputing(2024)
摘要
In this paper, an enhanced structural developmental neural network with information saturation (ESDNNIS) is proposed for continual unsupervised learning. It improves SDNNIS in the following respects: (1) it makes a distinction between the developmental stages of neurons to better regulate the updating of neurons; (2) it adopts the forgetting mechanism to control the network size; (3) it changes the way coverage domain parameter is initialized and updated to divide clusters more accurately; and (4) it adds a parent–child relationship update mechanism to provide better robustness to sample input order. ESDNNIS inherits the unsupervised incremental learning and class segmentation capabilities of SDNNIS, and achieves better class incremental clustering for more complex datasets while using fewer neurons than SDNNIS. The experimental results show that the accuracy of clustering results of ESDNNIS is improved by 10.94% on average compared with SDNNIS, while the number of generated neurons is reduced by 71.93% on average.
更多查看译文
关键词
Continual learning,Unsupervised learning,Structural developmental neural network,Information saturation
AI 理解论文
溯源树
样例
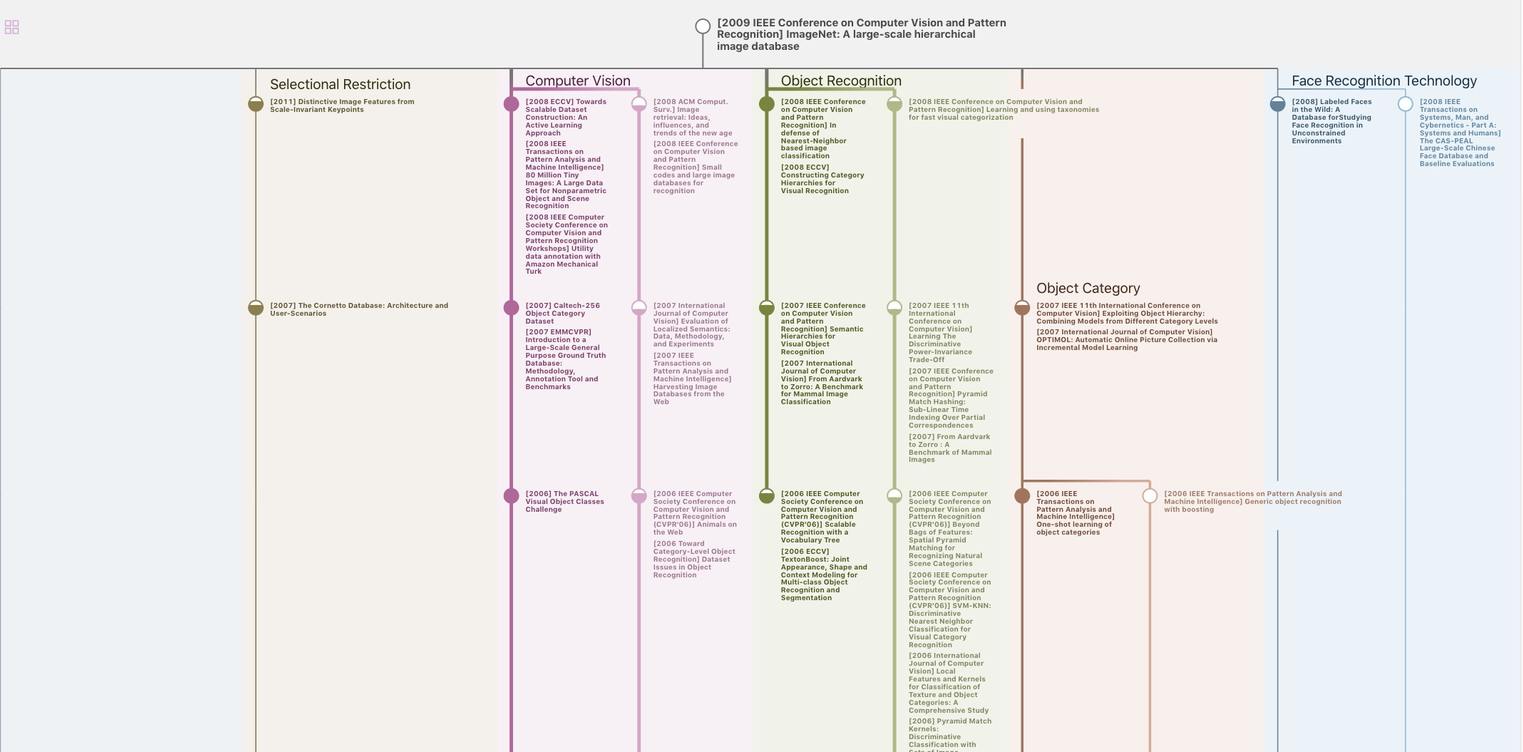
生成溯源树,研究论文发展脉络
Chat Paper
正在生成论文摘要