H2ABM: Heterogeneous Agent-based Model on Hypergraphs to Capture Group Interactions
PROCEEDINGS OF THE 2024 SIAM INTERNATIONAL CONFERENCE ON DATA MINING, SDM(2024)
摘要
Heterogeneous agent-based models (HABMs) can simulate the dynamics of multiple types of entities and their interactions on contact networks. In recent years, they have gathered great interest and are widely applied in multiple fields, such as personalized recommendations, publication ranking, and epidemic modeling. Nevertheless, conventional HABMs on graphs can only capture pair-wise interactions between agents but fail to capture the more complex dynamics of group interactions (e.g., multiple people in the same location simultaneously), consequently leading to suboptimal performance. To address this, we propose using hypergraphs to capture such group interactions better and extend the current graph-based HABMs to hypergraphs. Specifically, we use MRSA (Methicillin-resistant Staphylococcus aureus, a kind of infectious disease acquired by patients during treatment at healthcare facilities) spread in the University of Virginia hospital as an example to showcase how we extend an existing graph-based HABM, Graph-HeterSIS, to a hypergraph-based HABM (H(2)ABM), Hypergraph-HeterSIS. We show how the hyper graphs can capture the structural dioerence between contacts before and during the first wave of COVID-19 outbreak in Virginia better than graphs. Our experiments show that H2ABM better captures the underlying group interactions and better fits and forecasts MRSA cases.
更多查看译文
关键词
Heterogeneous agent-based models,Hypergraphs,Modeling,Forecasting
AI 理解论文
溯源树
样例
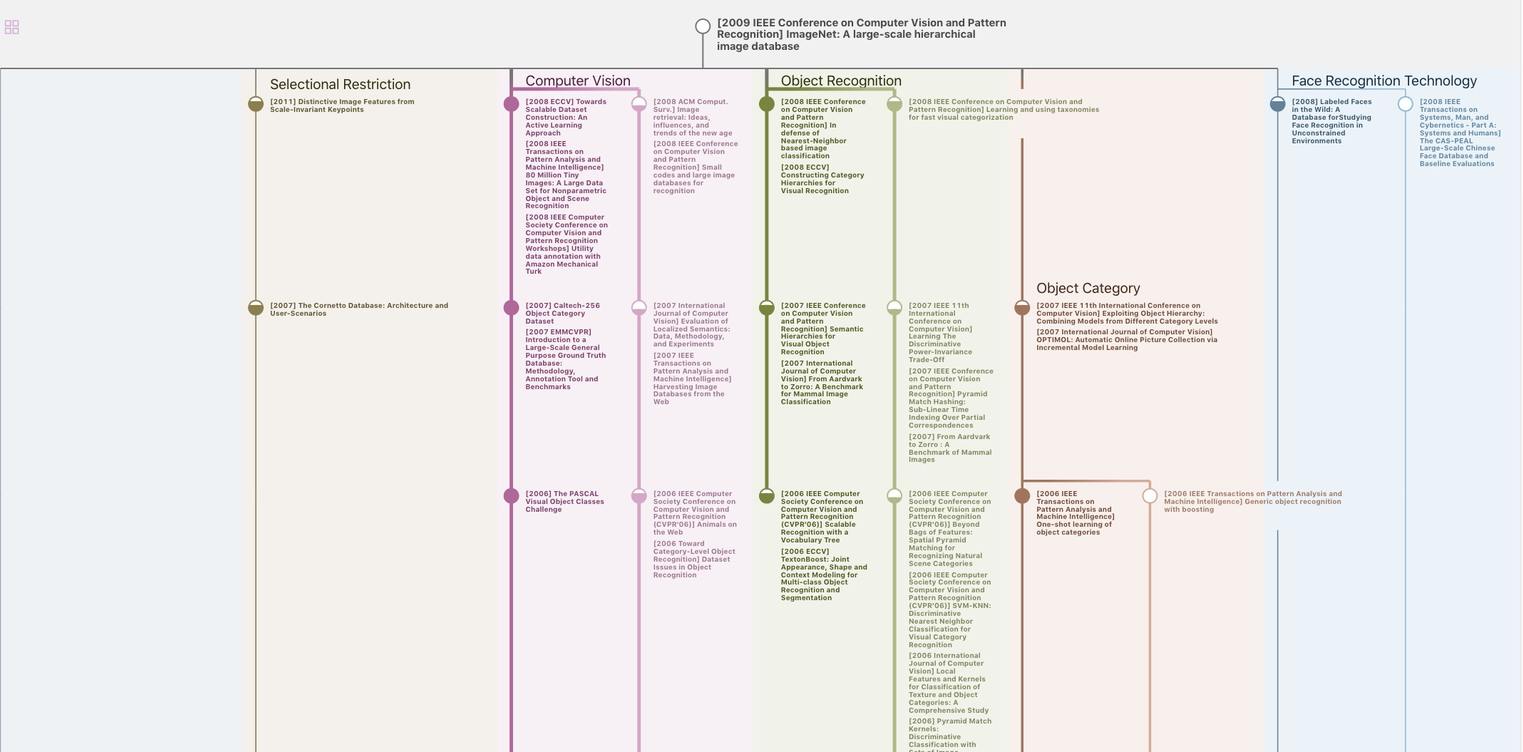
生成溯源树,研究论文发展脉络
Chat Paper
正在生成论文摘要