Clinical prediction of GBA carrier status in Parkinson’s disease
Clinical Parkinsonism & Related Disorders(2024)
摘要
Introduction
Given the unique natural history of GBA-related Parkinson’s disease (GBA-PD) and the potential for novel treatments in this population, genetic testing prioritization for the identification of GBA-PD patients is crucial for prognostication, individualizing treatment, and stratification for clinical trials. Assessing the predictive value of certain clinical traits for the GBA-variant carrier status will help target genetic testing in clinical settings where cost and access limit its availability.
Methods
In-depth clinical characterization through standardized rating scales for motor and non-motor symptoms and self-reported binomial information of a cohort of subjects with PD (n = 100) from our center and from the larger cohort of the Parkinson’s Progression Marker Initiative (PPMI) was utilized to evaluate the predictive values of clinical traits for GBA variant carrier status. The model was cross-validated across the two cohorts.
Results
Leveraging non-motor symptoms of PD, we established successful discrimination of GBA variants in the PPMI cohort and study cohort (AUC 0.897 and 0.738, respectively). The PPMI cohort model successfully generalized to the study cohort data using both MDS-UPDRS scores and binomial data (AUC 0.740 and 0.734, respectively) while the study cohort model did not.
Conclusions
We assessed the predictive value of non-motor symptoms of PD for identifying GBA carrier status in the general PD population. These data can be used to determine a simple, clinically oriented model using either the MDS-UPDRS or subjective symptom reporting from patients. Our results can inform patient counseling about the expected carrier risk and test prioritization for the expected identification of GBA variants.
更多查看译文
关键词
Parkinson’s disease,GBA,Genotype-phenotype,Predictive model,Non-motor symptoms
AI 理解论文
溯源树
样例
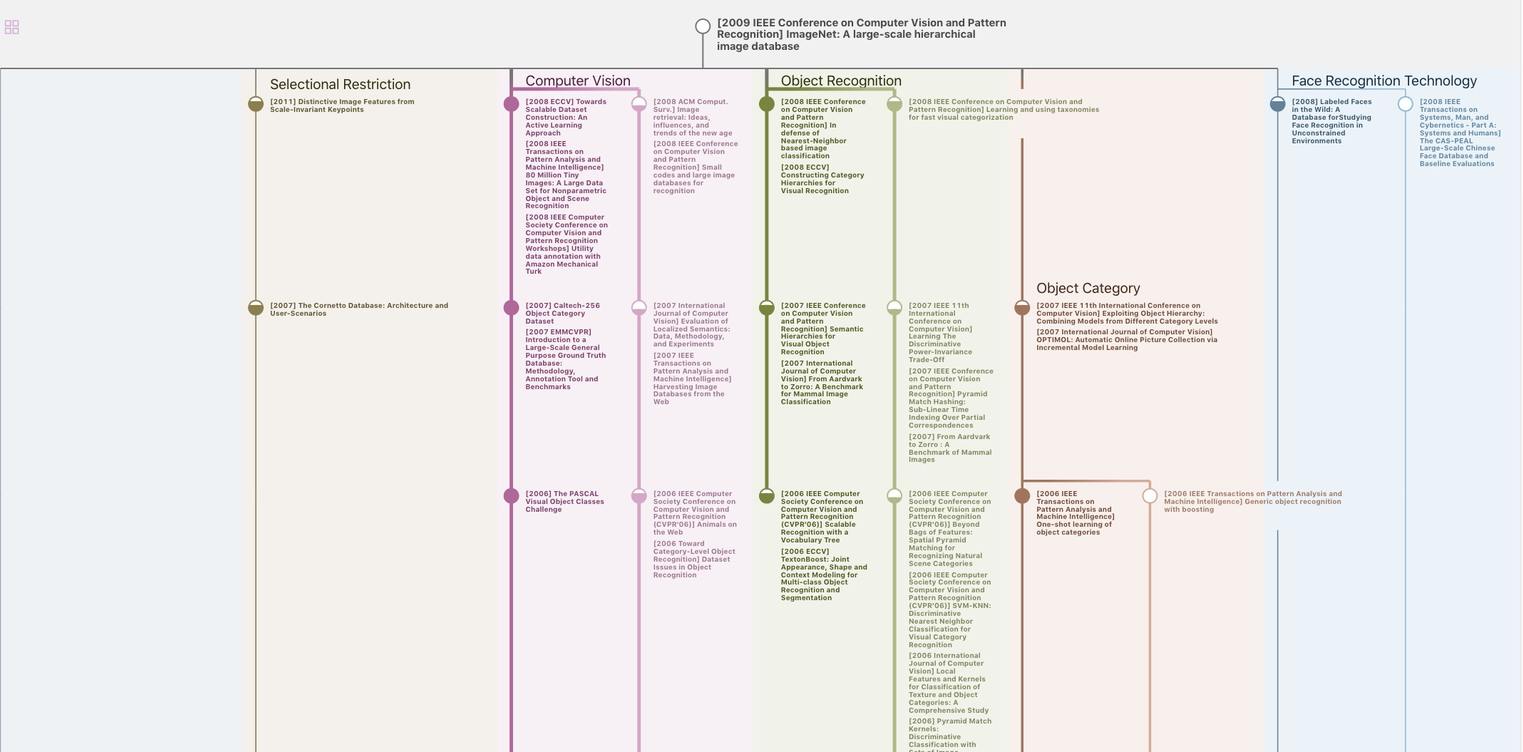
生成溯源树,研究论文发展脉络
Chat Paper
正在生成论文摘要