scCDX: enhancing cancer driver gene identification and model interpretability with single-cell RNA sequencing data and extreme gradient boosting
crossref(2024)
摘要
Abstract Background: Identifying cancer driver genes is a key step toward better understanding the molecular mechanism of cancers, which kill countless people worldwide. In recent years, numerous computational methods using biological data and networks have been developed for cancer driver gene identification. However, despite the rising number of single-cell RNA sequencing (scRNA-seq) data, none of the existing computational approaches have made use of scRNA-seq data. Moreover, it has been reported that deep learning models do not always outperform other machine learning models when applied to tabular data. Therefore, the current task of utilizing omics features, which can be presented in a tabular format, requires experimentation with various models to determine the best one. Results: In this study, we propose scCDX, extreme gradient boosting-based cancer driver gene identification method utilizing scRNA-seq data as well as bulk multi-omics data, topological feature in protein-protein interaction network, and systems-level features from various databases. The experimental results reveal that scCDX provides superior performance compared to graph neural network (GNN)-based state-of-the-art methods for cancer driver gene identification. The baselines were also retrained using the same features to ensure a fair evaluation, as they were trained on different set of features. The experimental results demonstrate that scCDX consistently outperforms, indicating that GNN-based deep learning is unnecessary. Furthermore, the scCDX prediction results were interpreted using the Shapley additive explanation method, which illustrates the contribution of each feature to the output. Conclusions: The study results suggest that selecting the appropriate model based on feature characteristics can achieve better performance than deep learning-based methods. Additionally, demonstrating the ability to interpret the model at the cell type level using high-resolution data, which was previously impossible, can ensure model reliability.
更多查看译文
AI 理解论文
溯源树
样例
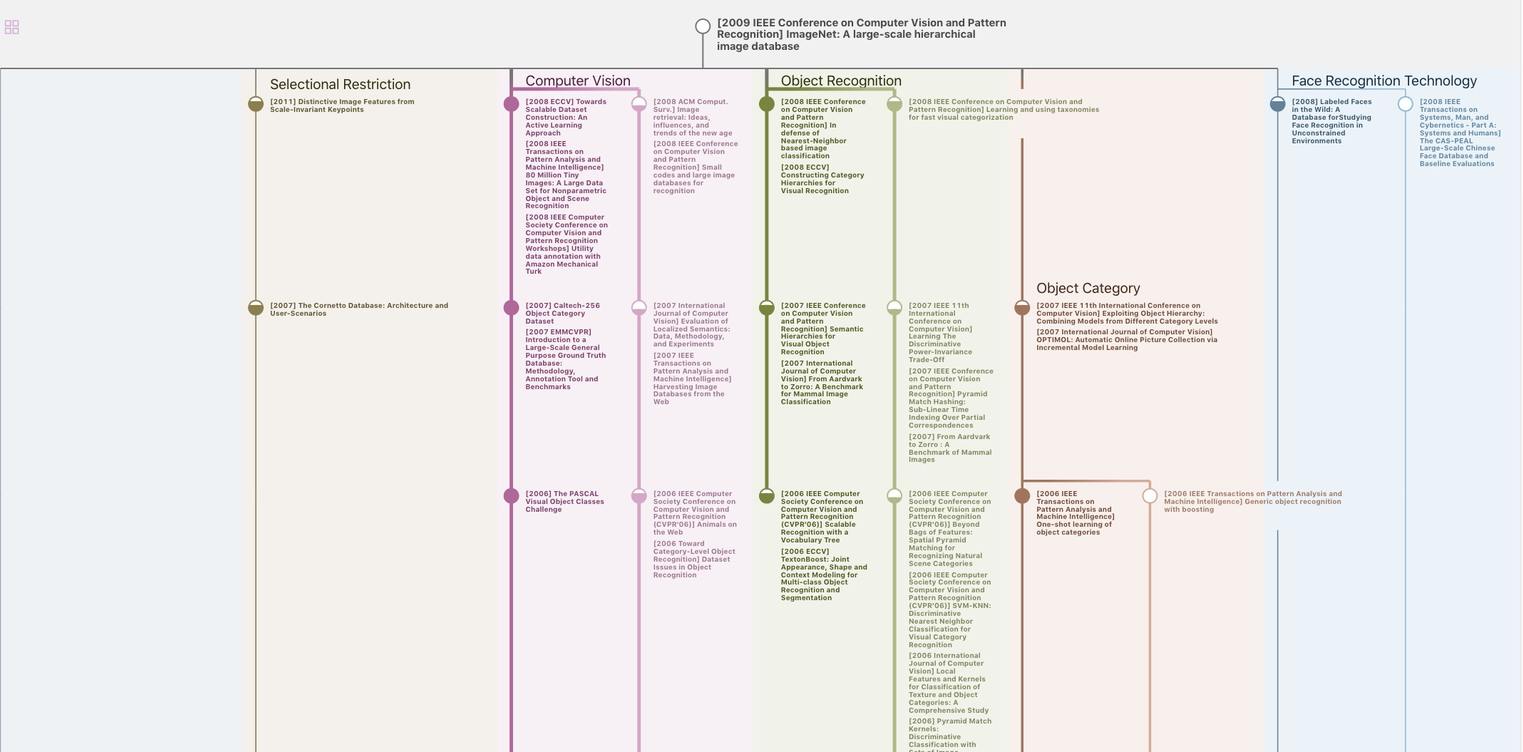
生成溯源树,研究论文发展脉络
Chat Paper
正在生成论文摘要