Adaboost-based Graph Convolutional Networks with Channel Attention for Intrusion Detection in Internet-of-Things
Parallel and Distributed Processing with Applications(2023)
Abstract
With the increasing proliferation of Internet of Things (IoT) and industrial big data technologies, while IoT scenarios offer intelligent services, cyberspace faces various types of threats and attacks. Despite Graph Convolutional Networks (GCN) having been demonstrated as a potent tool for learning graph data representations, many current IoT intrusion detection methods tend to overlook the adoption of graph-related techniques, given the non-graph-structured nature of most IoT traffic data. This leads to the model design overlooking the potential correlations among variables, thus limiting the accuracy of intrusion detection. In this study, we introduce an integrated intrusion detection model that fuses graph learning with a dual attention mechanism(A-GCA). Initially, our research adopts a combination of GCN and Convolutional Neural Networks (CNN) to deeply capture intricate interactions between data nodes. To further enhance the model’s feature-learning capability, we incorporate a channel attention mechanism for emphasizing features with pivotal information. Additionally, a multi-head attention mechanism is adopted to amalgamate a wealth of contextual information, ensuring more vivid feature representations. Regarding the training strategy, we employ a variant of Adaboost which uniquely steers weak classifiers from focusing on high-precision fitting of raw data towards precise binary classification tasks, aiming to augment the model’s overall performance in intrusion detection. Evaluations and performance analyses using real-world datasets UNSW-NB15 and CICIDS2017 indicate that the proposed method achieves improvements of 2.5% and 3.7% over the best baseline model in the accuracy and F1-score, respectively, relative to the baseline, surpassing other approaches.
MoreTranslated text
Key words
intrusion detection,graph convolutional network,channel attention,adaboost,attention mechanism
求助PDF
上传PDF
View via Publisher
AI Read Science
Must-Reading Tree
Example
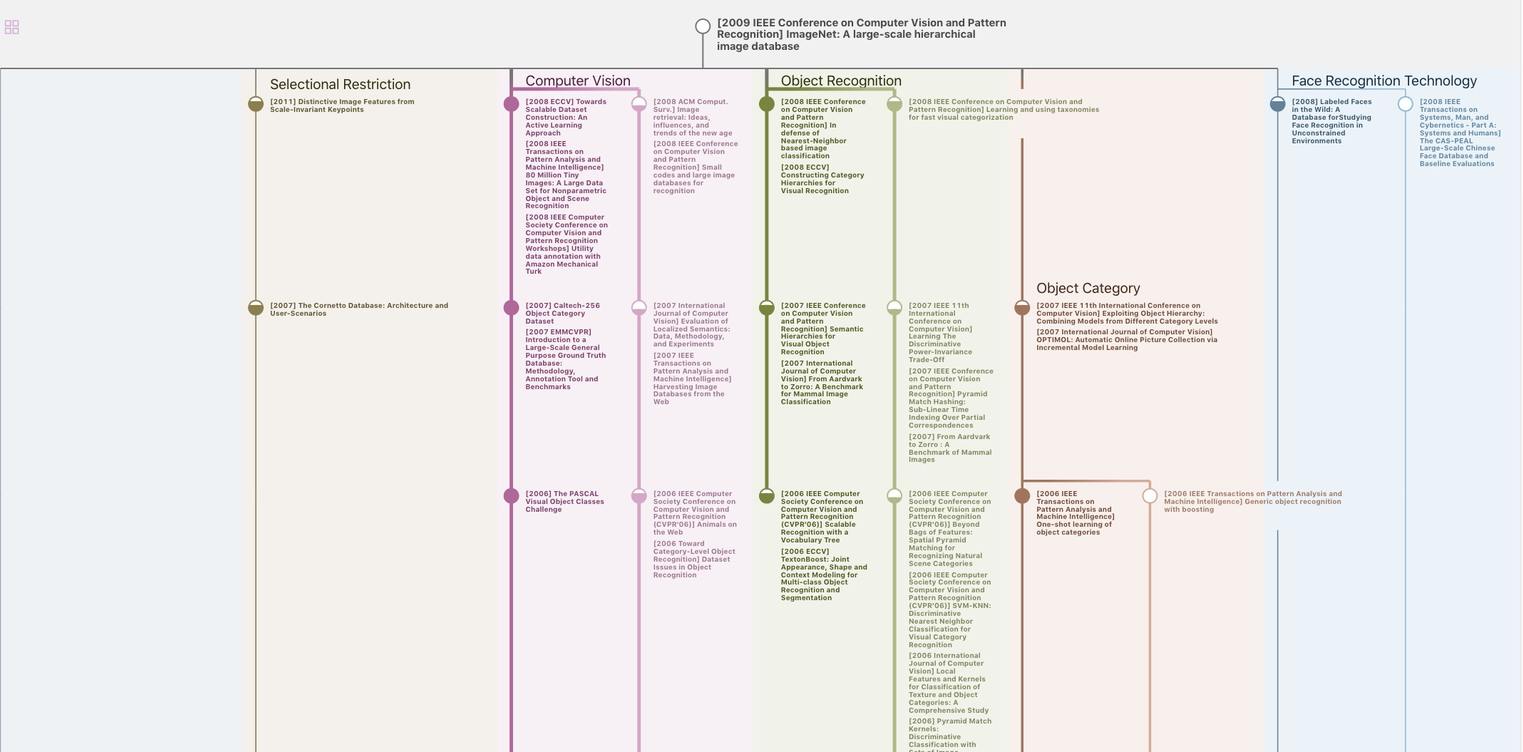
Generate MRT to find the research sequence of this paper
Data Disclaimer
The page data are from open Internet sources, cooperative publishers and automatic analysis results through AI technology. We do not make any commitments and guarantees for the validity, accuracy, correctness, reliability, completeness and timeliness of the page data. If you have any questions, please contact us by email: report@aminer.cn
Chat Paper
Summary is being generated by the instructions you defined