Enhancing Numerical Reasoning Performance by Augmenting Distractor Numerical Values.
IEEE International Conference on Big Data and Smart Computing(2024)
摘要
Large language models have exhibited remarkable proficiency in various domains and tasks including Numerical Reasoning task. However, datasets for Numerical Reasoning tasks targeted by LLMs often contain relatively few number of numerical values within the context. This raises doubts about whether LLMs can infer well and provide accurate answers even when presented with contexts including many numerical values. Indeed in a simple pilot study, we have found that LLM's ability to distinguish between essential and non-essential numerical values when solving numerical reasoning tasks is quite poor. To relieve this phenomenon, this paper proposes a framework to improve the robustness of LLMs in handling irrelevant numerical values for problem-solving by augmenting the original context of the problem while adding extra numerical values to the context. The experiment demonstrated that the model trained on the augmented dataset by our methodology increased robustness in Numerical Reasoning tasks with higher accuracies.
更多查看译文
关键词
data augmentation,numerical reasoning,large language model,math word problem
AI 理解论文
溯源树
样例
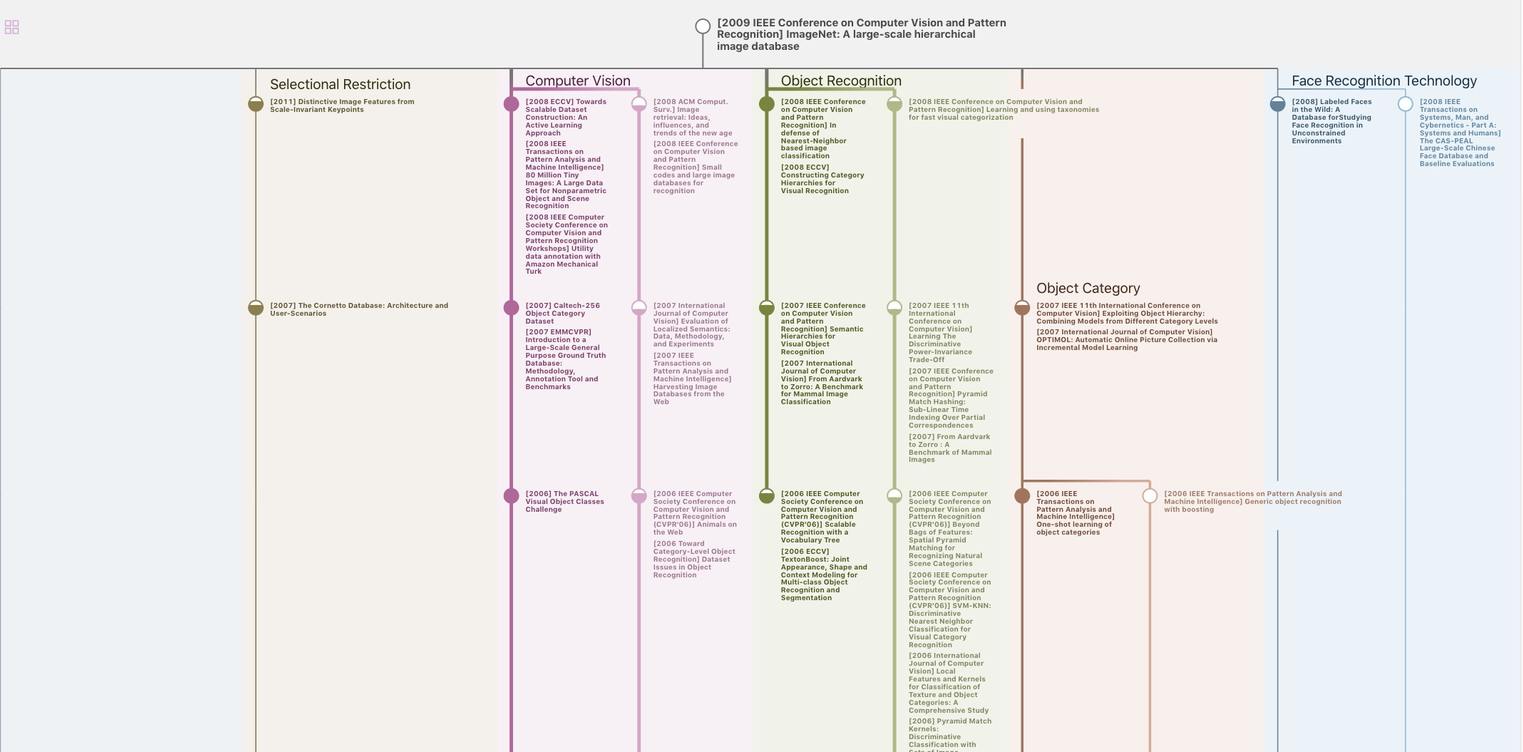
生成溯源树,研究论文发展脉络
Chat Paper
正在生成论文摘要