Incorporating Explanations into Human-Machine Interfaces for Trust and Situation Awareness in Autonomous Vehicles
2024 35TH IEEE INTELLIGENT VEHICLES SYMPOSIUM, IEEE IV 2024(2024)
Abstract
Autonomous vehicles often make complex decisions via machine learning-basedpredictive models applied to collected sensor data. While this combination ofmethods provides a foundation for real-time actions, self-driving behaviorprimarily remains opaque to end users. In this sense, explainability ofreal-time decisions is a crucial and natural requirement for building trust inautonomous vehicles. Moreover, as autonomous vehicles still cause serioustraffic accidents for various reasons, timely conveyance of upcoming hazards toroad users can help improve scene understanding and prevent potential risks.Hence, there is also a need to supply autonomous vehicles with user-friendlyinterfaces for effective human-machine teaming. Motivated by this problem, westudy the role of explainable AI and human-machine interface jointly inbuilding trust in vehicle autonomy. We first present a broad context of theexplanatory human-machine systems with the "3W1H" (what, whom, when, how)approach. Based on these findings, we present a situation awareness frameworkfor calibrating users' trust in self-driving behavior. Finally, we perform anexperiment on our framework, conduct a user study on it, and validate theempirical findings with hypothesis testing.
MoreTranslated text
Key words
Autonomous Vehicles,Situational Awareness,Trust In Autonomous Vehicles,User Study,Self-driving,Explainable Artificial Intelligence,Null Hypothesis,Visual Impairment,Vehicle Control,Alternative Hypothesis,Pedestrian,Interfacial Interaction,Mental Models,Traffic Light,Perceptions Of Safety,Feelings Of Safety,Human-machine Interaction,Human Drivers,Multimodal Learning,Traffic Scenarios,Feelings Of Comfort,Vehicle Behavior,Visual Question Answering,Explanatory Information,Traffic Situation,Correct Explanation,Bounding Box,User Satisfaction,Behavioral Information
PDF
View via Publisher
AI Read Science
Must-Reading Tree
Example
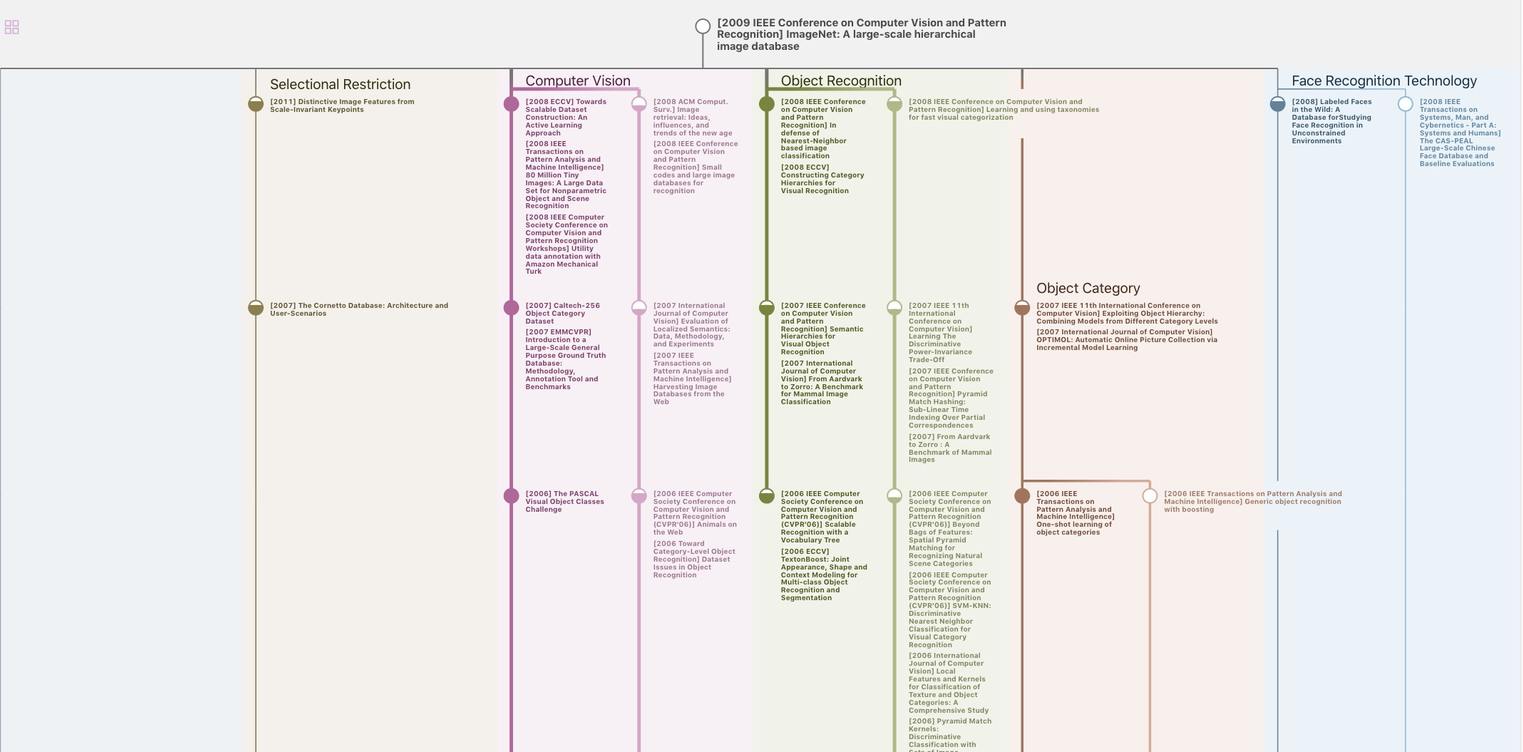
Generate MRT to find the research sequence of this paper
Data Disclaimer
The page data are from open Internet sources, cooperative publishers and automatic analysis results through AI technology. We do not make any commitments and guarantees for the validity, accuracy, correctness, reliability, completeness and timeliness of the page data. If you have any questions, please contact us by email: report@aminer.cn
Chat Paper
Summary is being generated by the instructions you defined