Joint mixed-effects models for causal inference in clustered network-based observational studies
arxiv(2024)
摘要
Causal inference on populations embedded in social networks poses technical
challenges, since the typical no interference assumption frequently does not
hold. Existing methods developed in the context of network interference rely
upon the assumption of no unmeasured confounding. However, when faced with
multilevel network data, there may be a latent factor influencing both the
exposure and the outcome at the cluster level. We propose a Bayesian inference
approach that combines a joint mixed-effects model for the outcome and the
exposure with direct standardization to identify and estimate causal effects in
the presence of network interference and unmeasured cluster confounding. In
simulations, we compare our proposed method with linear mixed and fixed effects
models and show that unbiased estimation is achieved using the joint model.
Having derived valid tools for estimation, we examine the effect of maternal
college education on adolescent school performance using data from the National
Longitudinal Study of Adolescent Health.
更多查看译文
AI 理解论文
溯源树
样例
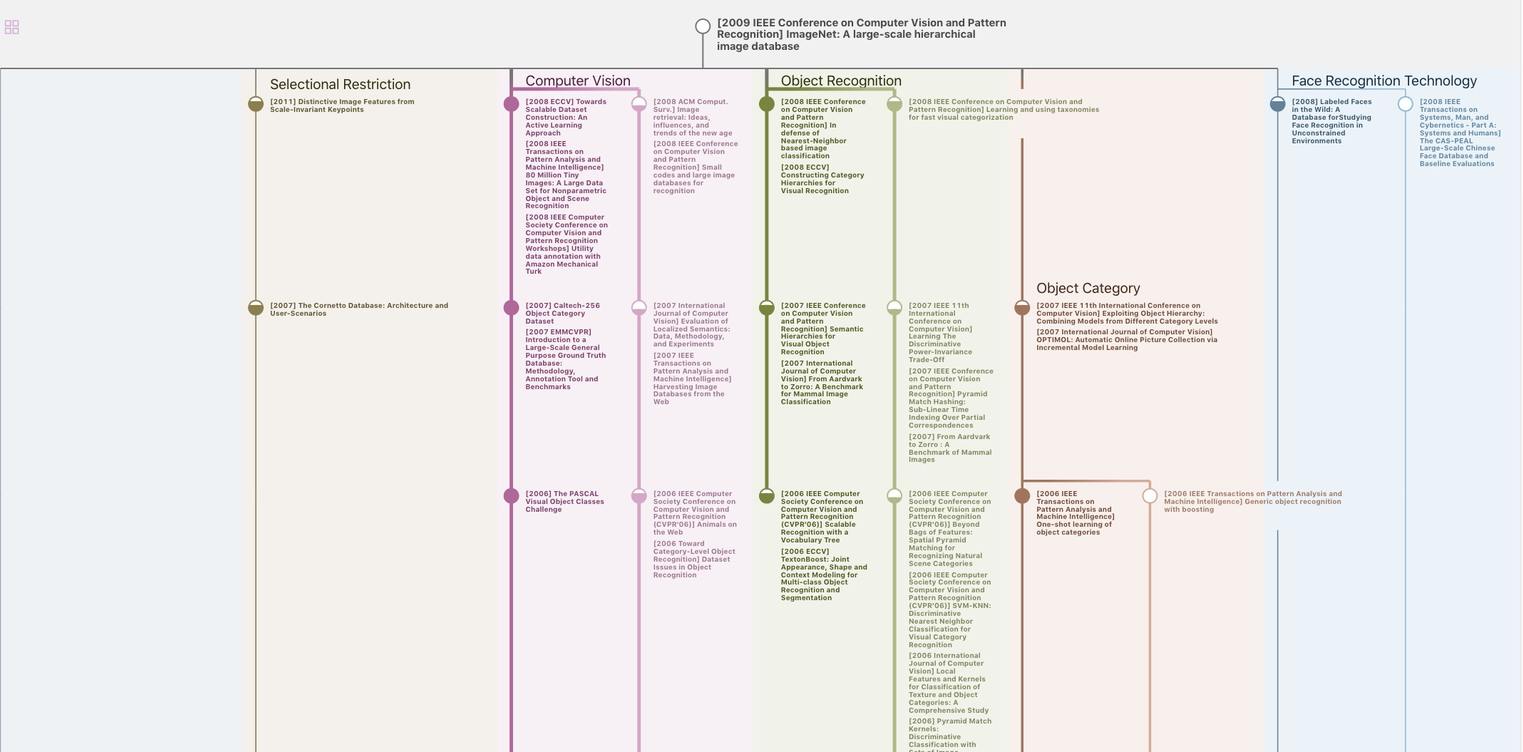
生成溯源树,研究论文发展脉络
Chat Paper
正在生成论文摘要