Point Cloud Geometry Scalable Coding with a Quality-Conditioned Latents Probability Estimator
CoRR(2024)
摘要
The widespread usage of point clouds (PC) for immersive visual applications
has resulted in the use of very heterogeneous receiving conditions and devices,
notably in terms of network, hardware, and display capabilities. In this
scenario, quality scalability, i.e., the ability to reconstruct a signal at
different qualities by progressively decoding a single bitstream, is a major
requirement that has yet to be conveniently addressed, notably in most
learning-based PC coding solutions. This paper proposes a quality scalability
scheme, named Scalable Quality Hyperprior (SQH), adaptable to learning-based
static point cloud geometry codecs, which uses a Quality-conditioned Latents
Probability Estimator (QuLPE) to decode a high-quality version of a PC
learning-based representation, based on an available lower quality base layer.
SQH is integrated in the future JPEG PC coding standard, allowing to create a
layered bitstream that can be used to progressively decode the PC geometry with
increasing quality and fidelity. Experimental results show that SQH offers the
quality scalability feature with very limited or no compression performance
penalty at all when compared with the corresponding non-scalable solution, thus
preserving the significant compression gains over other state-of-the-art PC
codecs.
更多查看译文
AI 理解论文
溯源树
样例
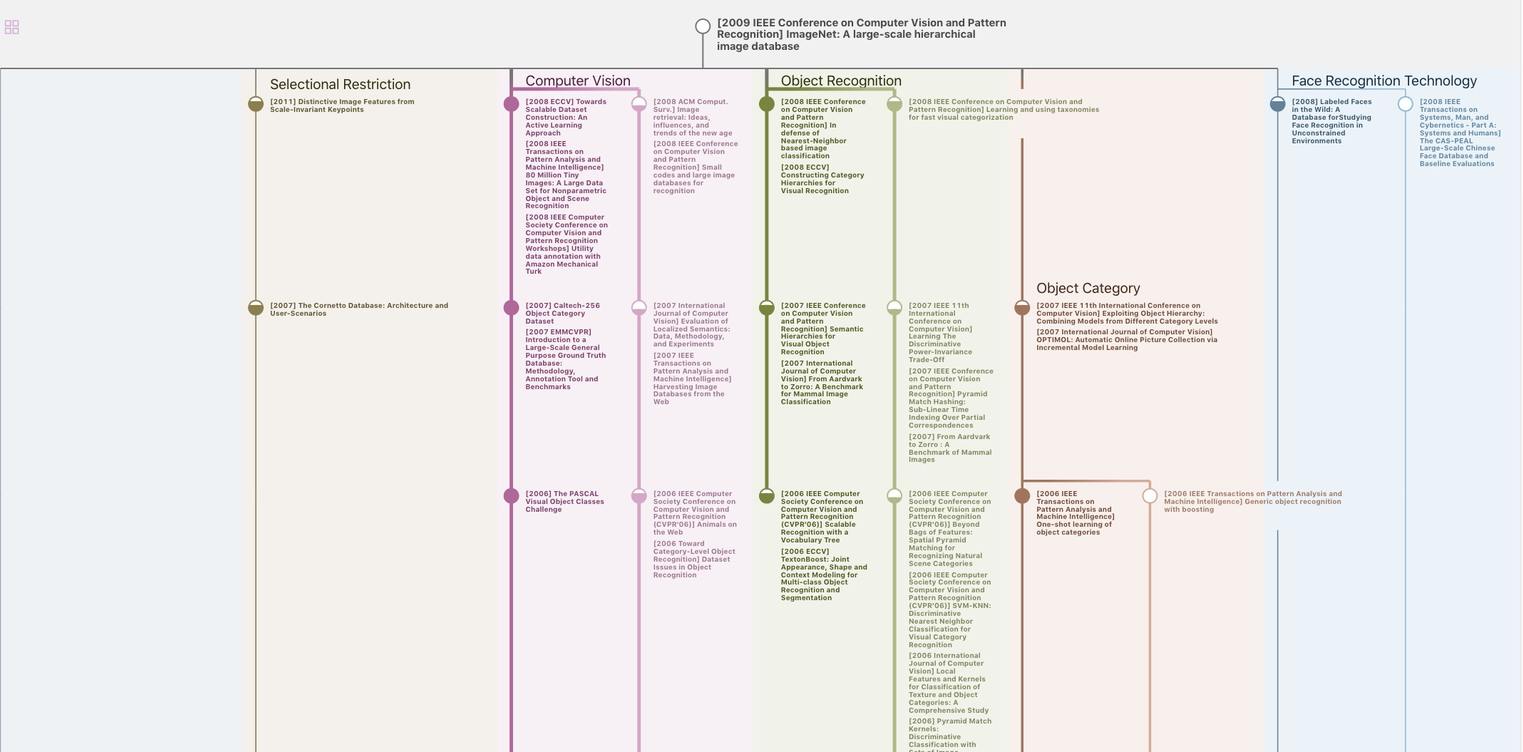
生成溯源树,研究论文发展脉络
Chat Paper
正在生成论文摘要