Optical next generation reservoir computing
CoRR(2024)
摘要
Artificial neural networks with dynamics exhibit remarkable capability in
processing information. Reservoir computing (RC) is a canonical example that
features rich computing expressivity and compatibility with physical
implementations for enhanced efficiency. Recently, a new RC paradigm known as
next generation reservoir computing (NGRC) further improves expressivity but
compromises the physical openness, posing challenges for neuromorphic
realizations. Here we demonstrate optical NGRC with large-scale computations
performed by light scattering through disordered media. In contrast to
conventional optical RC implementations, we drive our optical reservoir
directly with time-delay inputs. We show that, much like digital NGRC that
relies on polynomial features of delayed inputs, our optical reservoir also
implicitly generates these polynomial features for desired functionalities. By
leveraging the domain knowledge of the reservoir inputs, the optical NGRC not
only predicts the short-term dynamics of the low-dimensional Lorenz63 and
high-dimensional Kuramoto-Sivashinsky chaotic time series, but also replicates
their long-term ergodic properties. Optical NGRC shows superiority in shorter
training length, fewer hyperparameters and increased interpretability compared
to conventional optical RC, while achieving state-of-the-art forecasting
performance. Given its scalability and versatility, the optical NGRC framework
also paves the way for next generation physical RC, new applications and
architectures in a broad sense.
更多查看译文
AI 理解论文
溯源树
样例
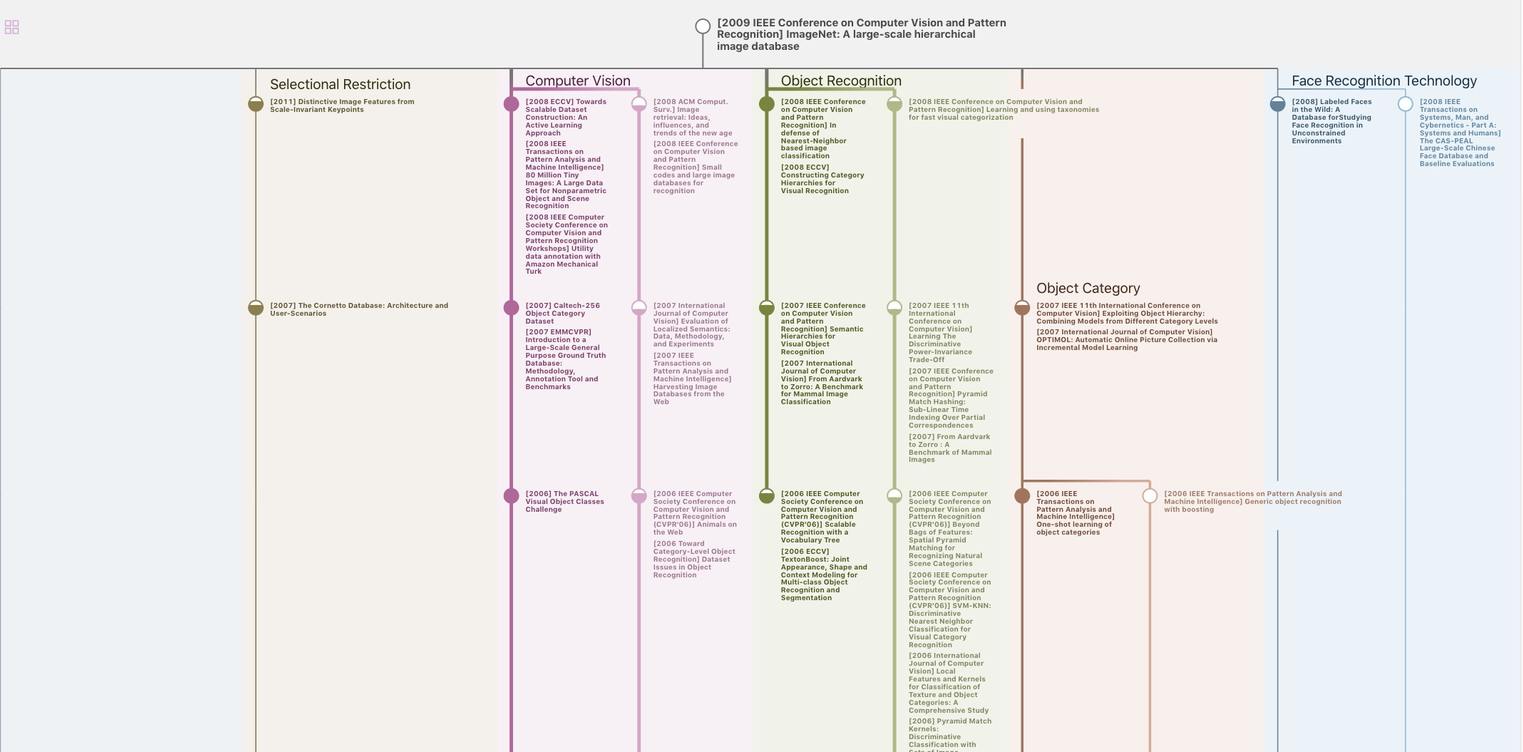
生成溯源树,研究论文发展脉络
Chat Paper
正在生成论文摘要