Triple Component Matrix Factorization: Untangling Global, Local, and Noisy Components
arXiv (Cornell University)(2024)
摘要
In this work, we study the problem of common and unique feature extractionfrom noisy data. When we have N observation matrices from N different andassociated sources corrupted by sparse and potentially gross noise, can werecover the common and unique components from these noisy observations? This isa challenging task as the number of parameters to estimate is approximatelythrice the number of observations. Despite the difficulty, we propose anintuitive alternating minimization algorithm called triple component matrixfactorization (TCMF) to recover the three components exactly. TCMF isdistinguished from existing works in literature thanks to two salient features.First, TCMF is a principled method to separate the three components given noisyobservations provably. Second, the bulk of the computation in TCMF can bedistributed. On the technical side, we formulate the problem as a constrainednonconvex nonsmooth optimization problem. Despite the intricate nature of theproblem, we provide a Taylor series characterization of its solution by solvingthe corresponding Karush-Kuhn-Tucker conditions. Using this characterization,we can show that the alternating minimization algorithm makes significantprogress at each iteration and converges into the ground truth at a linearrate. Numerical experiments in video segmentation and anomaly detectionhighlight the superior feature extraction abilities of TCMF.
更多查看译文
关键词
Feature Extraction,Compressed Sensing,Independent Component Analysis,Sparse Approximation,Change Detection
AI 理解论文
溯源树
样例
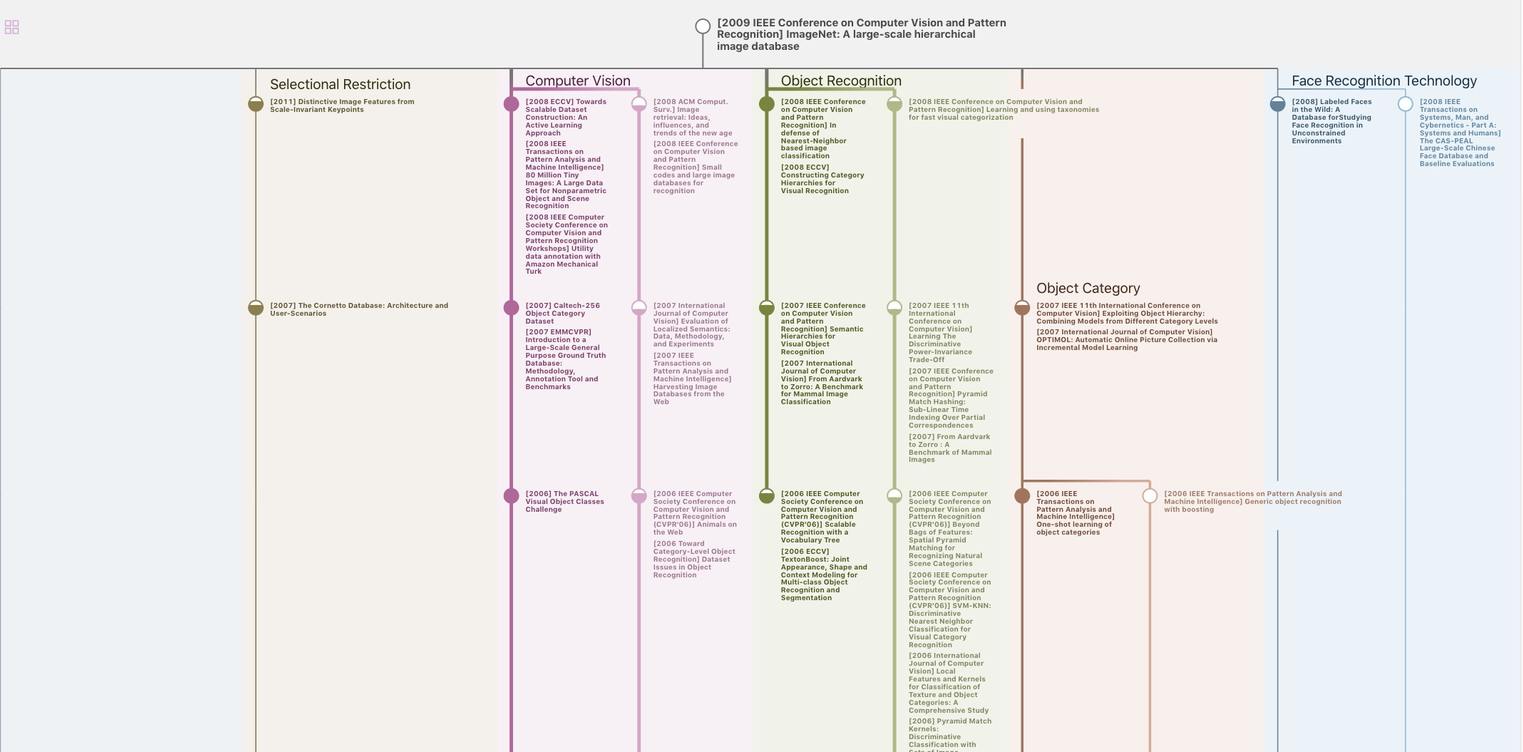
生成溯源树,研究论文发展脉络
Chat Paper
正在生成论文摘要