Semi-asynchronous Federated Learning Optimized for NON-IID Data Communication based on Tensor Decomposition.
Parallel and Distributed Processing with Applications(2023)
摘要
Federated Learning (FL) is a new distributed machine learning framework that enables reliable collaborative training without collecting users’ private data, it can successfully address the issue of data silos. However, FL has had trouble scaling to statistically varied data and large-scale models because of its frequent communication and average aggregation procedures. In this paper, we propose a Semi-asynchronous Stochastic Controlled Averaging based on Tensor Decomposition for Federated Learning (SATD-SCAFFOLD), in which we perform tensor decomposition on the client model to reduce communication costs. In addition, we design a semi-asynchronous aggregation approach for the server, which can prevent server from wasting too much time waiting for data from late clients in the case of an unstable network, and the overall model still has excellent performance. Thorough experiments demonstrate that our proposed SATDSCAFFOLD algorithm can reduce both the communication cost and convergence time while maintaining good performance.
更多查看译文
关键词
Federated learning,tensor decomposition,non-IID,Asynchronous Federated
AI 理解论文
溯源树
样例
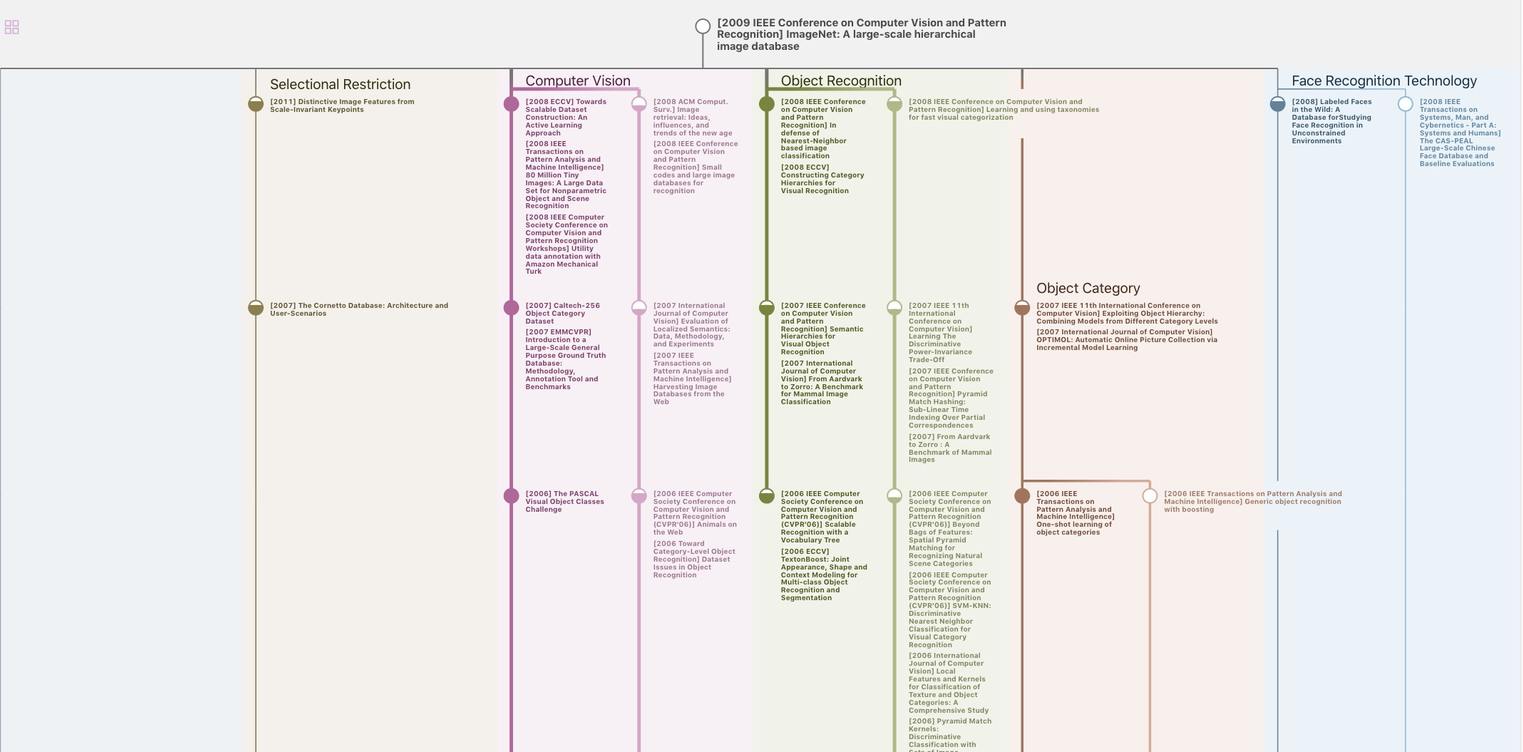
生成溯源树,研究论文发展脉络
Chat Paper
正在生成论文摘要