Magnetic resonance radiomics differentiates gliosarcoma from glioblastoma multiforme
crossref(2024)
摘要
Abstract Background: Traditional radiological imaging has a limited ability to differentiate gliosarcoma (GS) from glioblastoma multiforme (GBM). The aim of this study was to develop a magnetic resonance (MR) radiomic method to preoperatively differentiate GS from GBM and predict the survival of patients with GS. Methods: A single-center retrospective review was conducted on 39 patients with GS and 316 patients with GBM. MR radiomic models for differentiating GS from GBM were built with machine learning methods. Model performance was evaluated with discrimination, calibration, and decision curve analysis. Multivariate Cox regression was used to calculate risk scores from MR radiomics and clinical features and to predict survival for patients with GS. Results: The final MR radiomic model provided an area under the curve (AUC) of 0.9420 (95% CI: 0.8922–0.9918) with an accuracy of 0.9280 (95% CI: 0.8119-0.9760) in the training cohort, and an AUC of 0.9447 (95% CI: 0.9025–0.9870) with an accuracy of 0.8972 (95% CI: 0.8318-0.9533) in the validation cohort, for differentiating GS from GBM. The AUC for predicting one-year survival of GS patients using our radiomic method was 0.882. GS patients with lower risk scores had significantly longer survival than those with higher risk scores (p=0.0007, log-rank test). Conclusions: This study showed promising results for differentiating GS from GSM using an MR-based machine learning radiomic method. In addition, combining the MR radiomic features and clinical data into a risk score demonstrated the potential for this approach to predict prognosis for GS patients.
更多查看译文
AI 理解论文
溯源树
样例
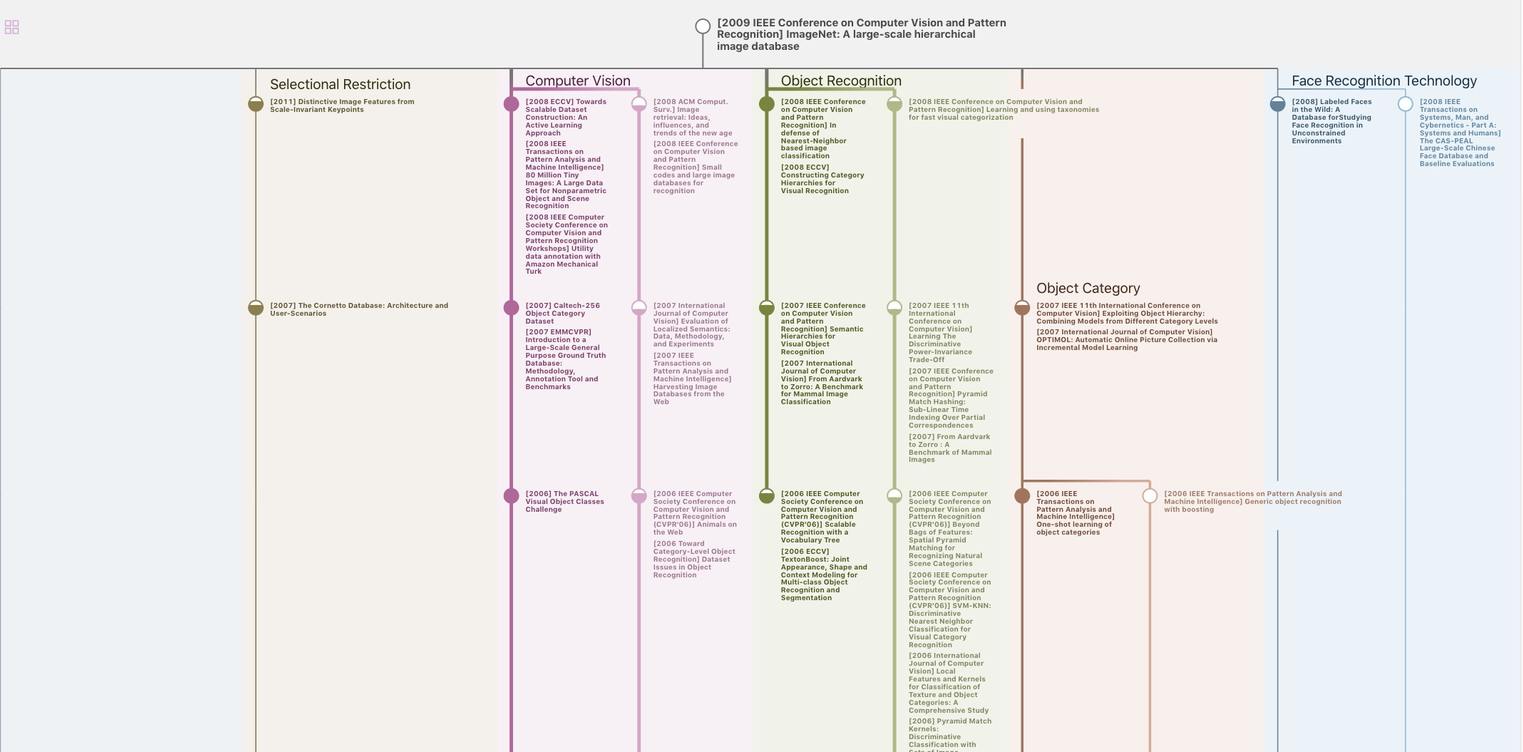
生成溯源树,研究论文发展脉络
Chat Paper
正在生成论文摘要