Geometric Pooling: Maintaining More Representative Information
IEEE ACCESS(2024)
摘要
Graph Pooling technology plays an important role in graph node classification tasks. Sorting pooling technologies maintain large-value units for pooling graphs of varying sizes. However, by analyzing the statistical characteristic of activated units after pooling, we found that a large number of units dropped by sorting pooling are negative-value units that contain representative information and can contribute considerably to the final decision. To maintain more representative information, we proposed a novel pooling technology, called Geometric Pooling (GP), containing the unique node features with negative values by measuring the similarity of all node features. We reveal the effectiveness of GP from the entropy reduction view. The experiments were conducted on TUdatasets to show the effectiveness of GP. The results showed that the proposed GP outperforms the SOTA graph pooling technologies by 1%similar to 5% with fewer parameters.
更多查看译文
关键词
Sorting,Smoothing methods,Entropy,Training data,Convolutional neural networks,Topology,Task analysis,Graph neural networks,pooling,similarity
AI 理解论文
溯源树
样例
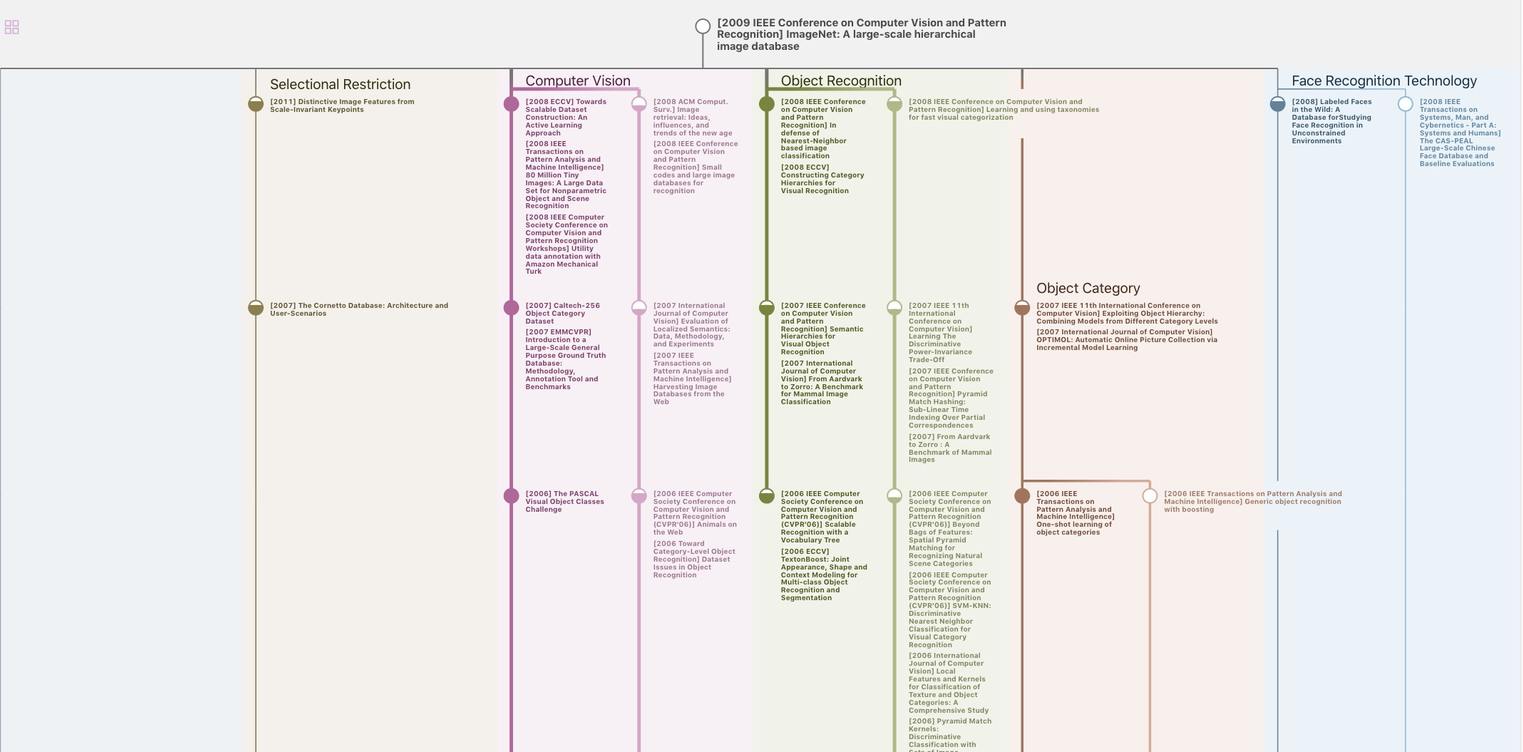
生成溯源树,研究论文发展脉络
Chat Paper
正在生成论文摘要