Identifying and Ranking of Best Influential Spreaders With Extended Clustering Coefficient Local Global Centrality Method
IEEE ACCESS(2024)
摘要
The detection and ranking of influential nodes in complex networks are crucial for various practical applications such as identifying potential drug targets in protein-to-protein interaction networks, critical devices in communication networks, key people in social networks, and transportation hubs in logistics networks. The knowledge of influential spreaders in complex networks is extremely useful for controlling the spread of information. Centrality measures are known for effectively quantifying the influential nodes information in large-scale complex networks. Researchers have proposed different centrality measures in the literature, including Degree, Betweenness, Closeness, and Clustering coefficient centralities. However, these measures have certain limitations when implemented over large-scale complex networks. Most of these measures can be classified as global and local structural approaches. The global structure based algorithms are too complex to evaluate key nodes, particularly in large-scale networks, whereas the local measures overlook the essential global network information. To address these challenges, an extended clustering coefficient local global centrality (ECLGC) is proposed, which combines the local and global structural information to measure the node's influence in large-scale networks. The effectiveness and computational efficiency of the proposed measure are compared with existing centrality measures on real-world network datasets. Susceptible-Infected-Recovered (SIR) model is utilized to evaluate the performance of the ECLGC to capture the high-information dissemination compared to conventional measures. Further, we demonstrate that the proposed measure outperforms the conventional measures in terms of spreading efficiency.
更多查看译文
关键词
Influential nodes,complex networks,extended clustering coefficient local global centrality,clustering coefficient
AI 理解论文
溯源树
样例
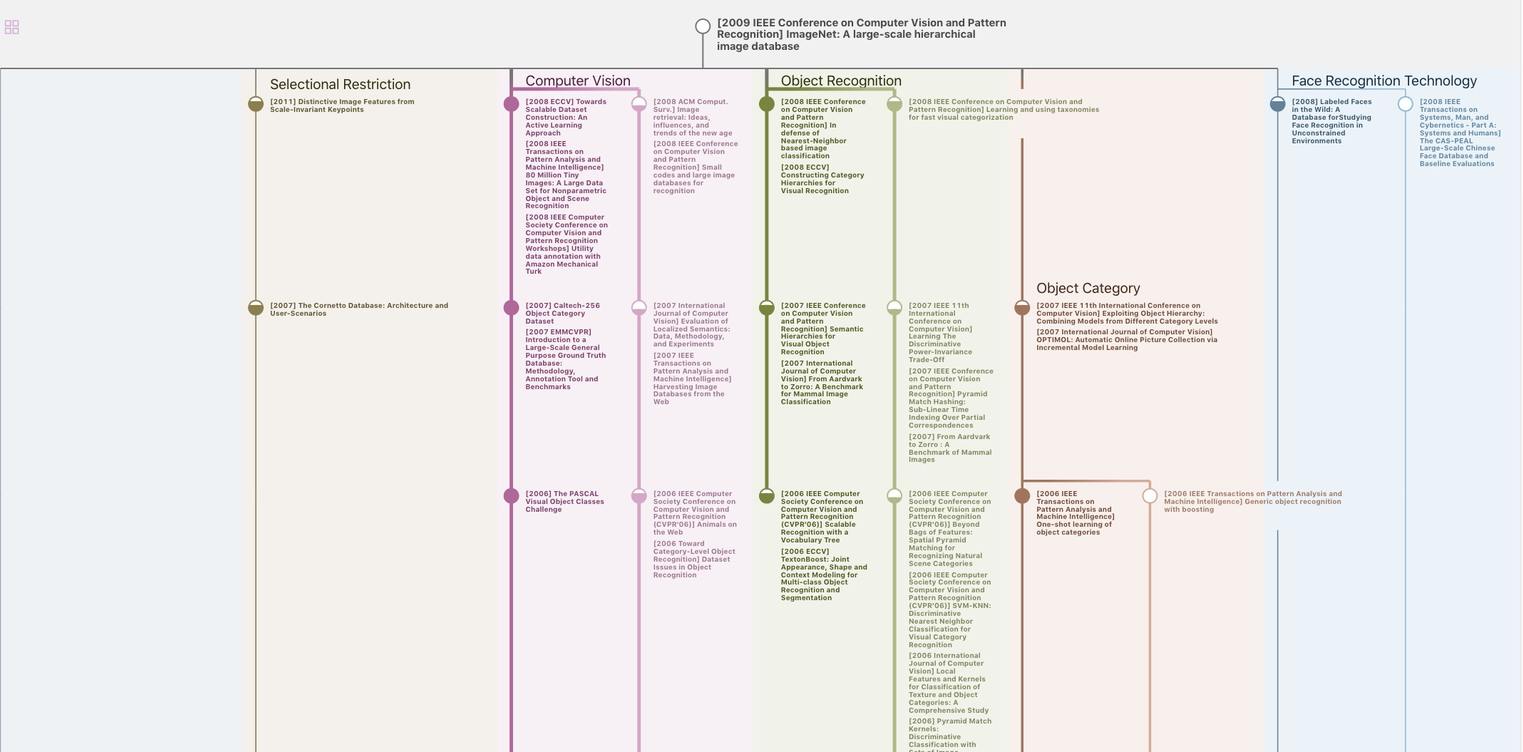
生成溯源树,研究论文发展脉络
Chat Paper
正在生成论文摘要