End-to-end UAV Intelligent Training via Deep Reinforcement Learning.
International Conference on Robotics, Intelligent Control and Artificial Intelligence(2023)
摘要
In this paper, we propose an end-to-end training method based on deep reinforcement learning for motion control problems in Unmanned-Aerial-Vehicle (UAV) active target tracking and autonomous obstacle avoidance. Proximal policy optimization (PPO) is employed as a deep reinforcement learning method to train artificial neural networks in an end-to-end manner using a continuous reward function. The proposed approach combines visual perception and deep reinforcement learning policy into an end-to-end decision control model. It takes RGB visual images observed by the UAV as input states, and outputs discrete control actions for UAV flight. Environment augmentation techniques and custom reward functions are utilized to enhance training efficiency and generalization capability. The training and validation are conducted in a simulation environment built using the Unreal Engine and Microsoft AirSim. The results of the simulation experiments demonstrate that the proposed approach can achieve autonomous tracking control of maneuvering targets for UAVs with good robustness and generalization. Moreover, for UAV autonomous obstacle avoidance tasks, the trained UAV based on this method can achieve fast and stable visual obstacle avoidance in unknown environments.
更多查看译文
关键词
Deep Reinforcement Learning,Active Target Tracking,Autonomous Obstacle Avoidance
AI 理解论文
溯源树
样例
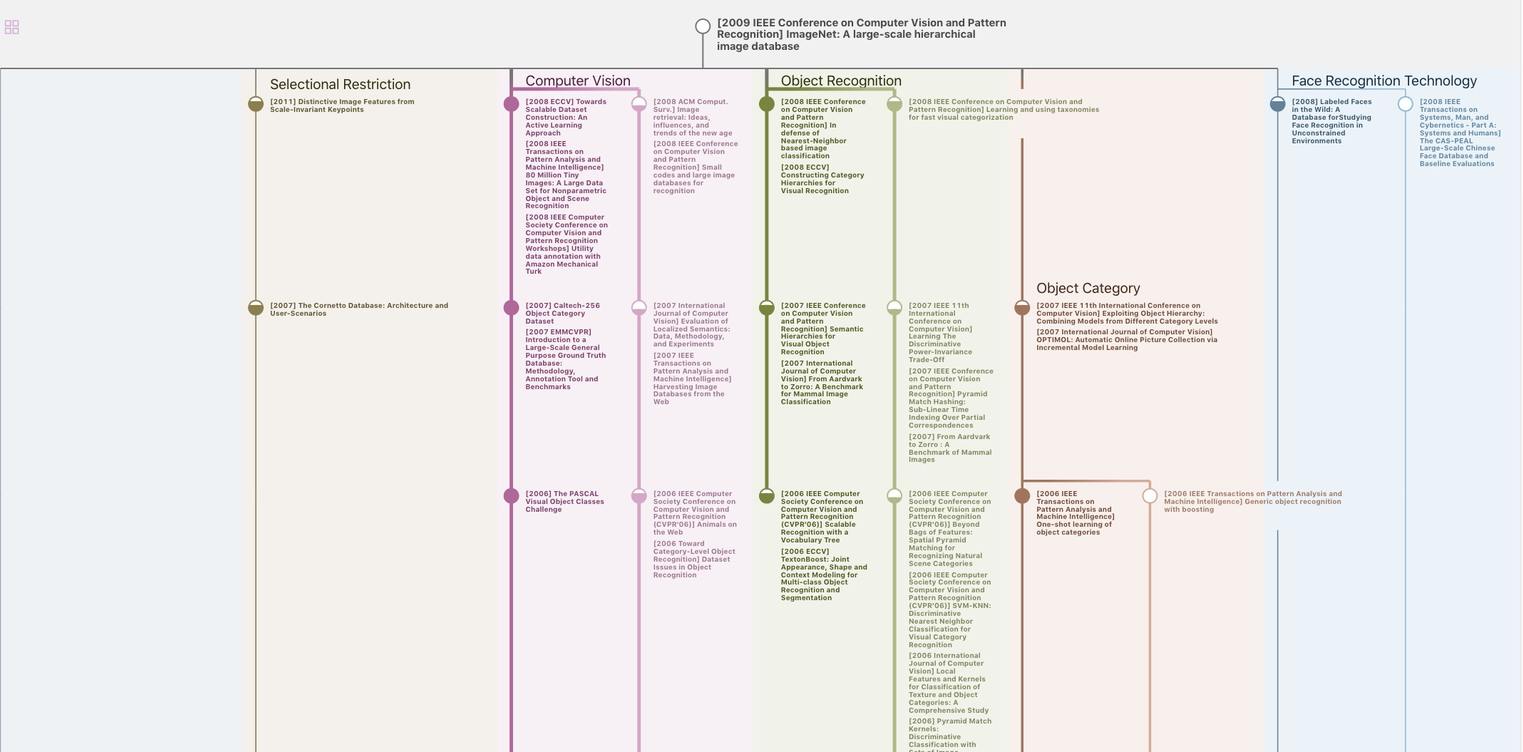
生成溯源树,研究论文发展脉络
Chat Paper
正在生成论文摘要