A Road Surface Defect Segmentation Model Based on GLoRA and Multi-Scale Completeness
2024 IEEE INTERNATIONAL CONFERENCE ON BIG DATA AND SMART COMPUTING, IEEE BIGCOMP 2024(2024)
摘要
The accurate identification of road surface defect images is of paramount importance in the field of automated road maintenance, as it not only reduces maintenance costs but also enhances road safety and maintenance efficiency. However, the complexity of the defect identification task is compounded by challenges such as the small proportion of foreground pixels in road surface defects, severe class imbalance, and low image quality. In this work, we address the bottlenecks in mainstream model training and the lack of completeness in prediction results by proposing a road surface defect semantic segmentation model called G-MCNet, which combines Generalized LoRA (GLoRA) with Multi-scale Completeness. Firstly, we replace traditional fine-tuning method with the efficient parameter tuning method, GLoRA, to adapt to the road surface defect segmentation task in complex backgrounds. Subsequently, an attention sparse sampling strategy and adaptive fusion of spatial and channel features are utilized in the introduction of a new Multi-scale Feature Aggregation Attention Module (MFDAM) to enhance the expressive power of global and local features. Moreover, we introduce capsule units from the capsule network model to represent the pose and spatial relationships of the foreground defects, ensuring the completeness of prediction results through dynamic routing algorithms. To validate the effectiveness of the proposed model, a real-world road defect segmentation dataset is constructed, and comparative experiments are conducted with multiple baseline models on this dataset, as well as publicly available datasets. The experimental results provide evidence of the effectiveness of the proposed model.
更多查看译文
关键词
GLoRA,Multi-scale Completeness,Multi-scale Feature Aggregation Attention Module,Capsule Network,semantic segmentation,road surface defect
AI 理解论文
溯源树
样例
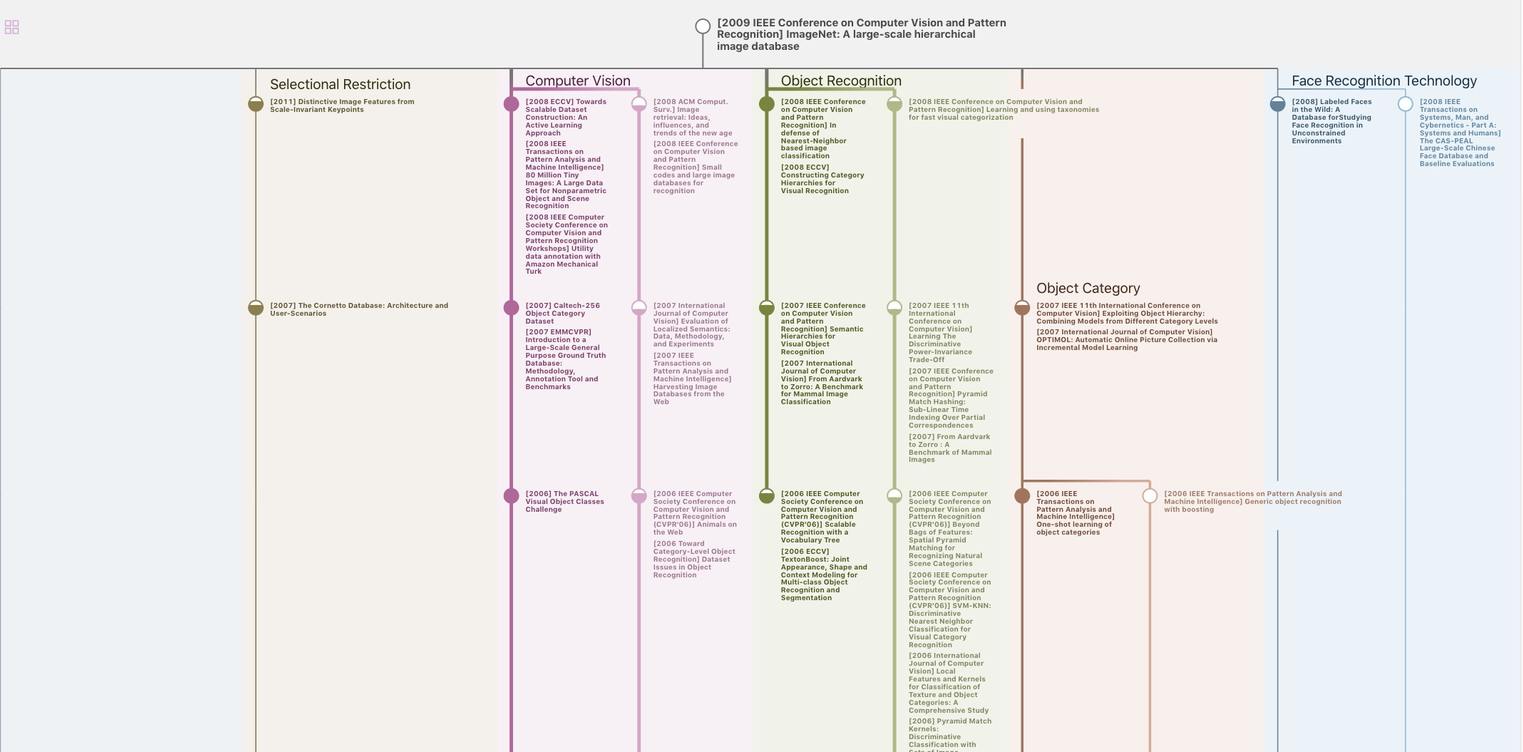
生成溯源树,研究论文发展脉络
Chat Paper
正在生成论文摘要