Target Detection Algorithm Based on Improved YOLOv5 for Edge Computing Devices.
International Conference on Robotics, Intelligent Control and Artificial Intelligence(2023)
摘要
This paper selects YOLOv5 as the basic model of edge computing device target detection. Aiming at the performance imbalance of the current deep neural network target detection algorithms and the high latency of deploying YOLOv5 on edge computing devices, an improved target detection algorithm based on YOLOv5 is named AG-YOLOv5 (Attention Ghost YOLOv5). The improvement of the algorithm mainly focuses on the backbone network and necking,to improve the comprehensive performance of the model. Firstly, in the backbone network, the Deep Stem module was used to replace convolutions, the improved C3Ghost is named S-C3Ghost(Strong C3Ghost) that was used to replace the CSP module, and SimSPPF was used instead of SPPF to achieve lightweight network and to improve inference speed. Secondly, attention mechanisms were added to the end and neck of the backbone network to improve the accuracy of object detection. The final function section utilizes Wlou instead of Clou as the loss function, and uses HardSwish activation function instead of SiLU, which improves the comprehensive performance of the model.
更多查看译文
关键词
yolov5,edgecomputing devices,C3Ghost,TensorRT,target detection
AI 理解论文
溯源树
样例
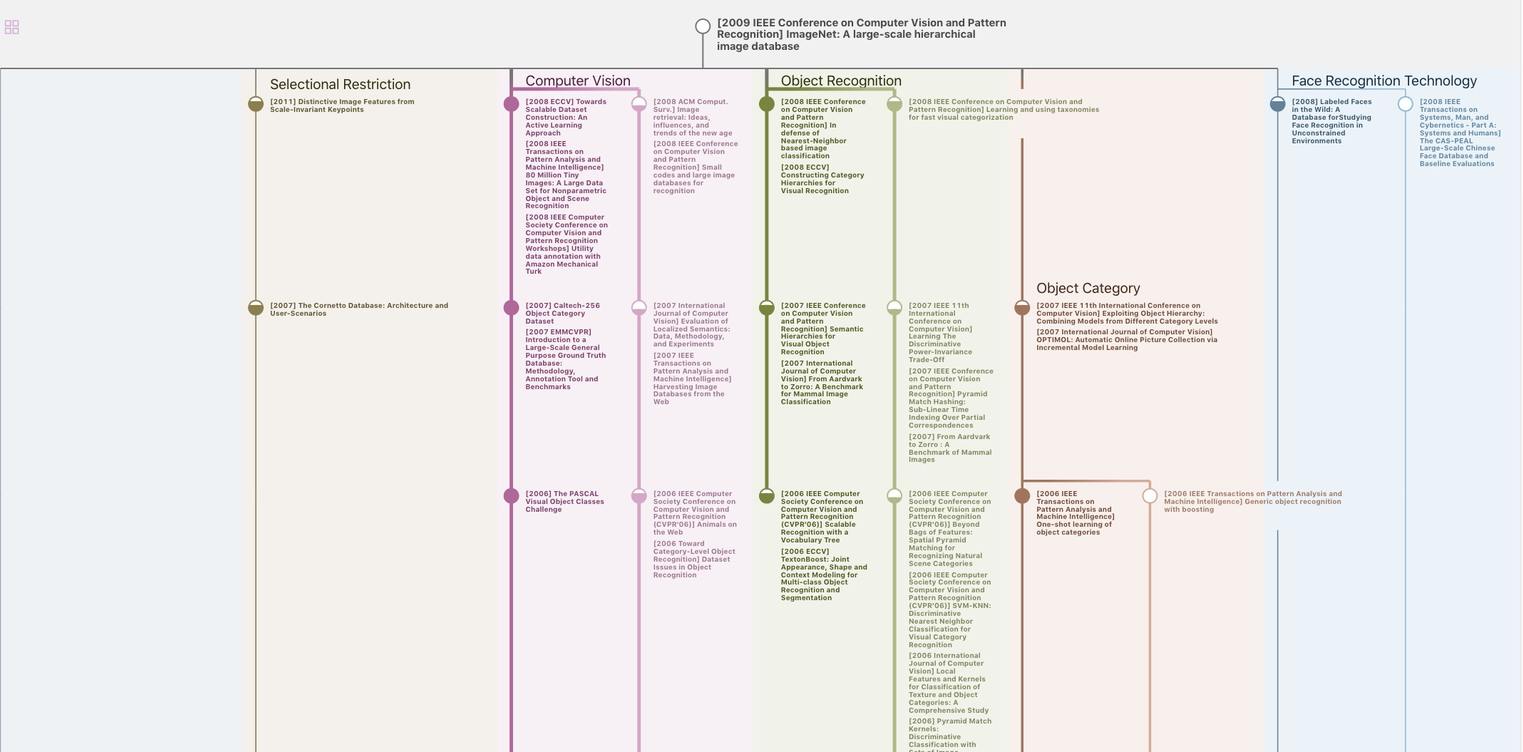
生成溯源树,研究论文发展脉络
Chat Paper
正在生成论文摘要