STTMC: A Few-shot Spatial Temporal Transductive Modulation Classifier
IEEE Transactions on Machine Learning in Communications and Networking(2024)
摘要
?The advancement of deep learning (DL) techniques has led to significant progress in Automatic Modulation Classification (AMC). However, most existing DL-based AMC methods require massive training samples, which are difficult to obtain in non-cooperative scenarios. The identification of modulation types under small sample conditions has become an increasingly urgent problem. In this paper, we present a novel few-shot AMC model named the Spatial Temporal Transductive Modulation Classifier (STTMC), which comprises two modules: a feature extraction module and a graph network module. The former is responsible for extracting diverse features through a spatiotemporal parallel network, while the latter facilitates transductive decision-making through a graph network that uses a closed-form solution. Notably, STTMC classifies a group of test signals simultaneously to increase stability of few-shot model with an episode training strategy. Experimental results on the RadioML.2018.01A and RadioML.2016.10A datasets demonstrate that the proposed method perform well in 3way-Kshot, 5way-Kshot and 10way-Kshot configurations. In particular, STTMC outperforms other existing AMC methods by a large margin.
更多查看译文
关键词
Automatic Modulation Classification,few-shot learning,graph network
AI 理解论文
溯源树
样例
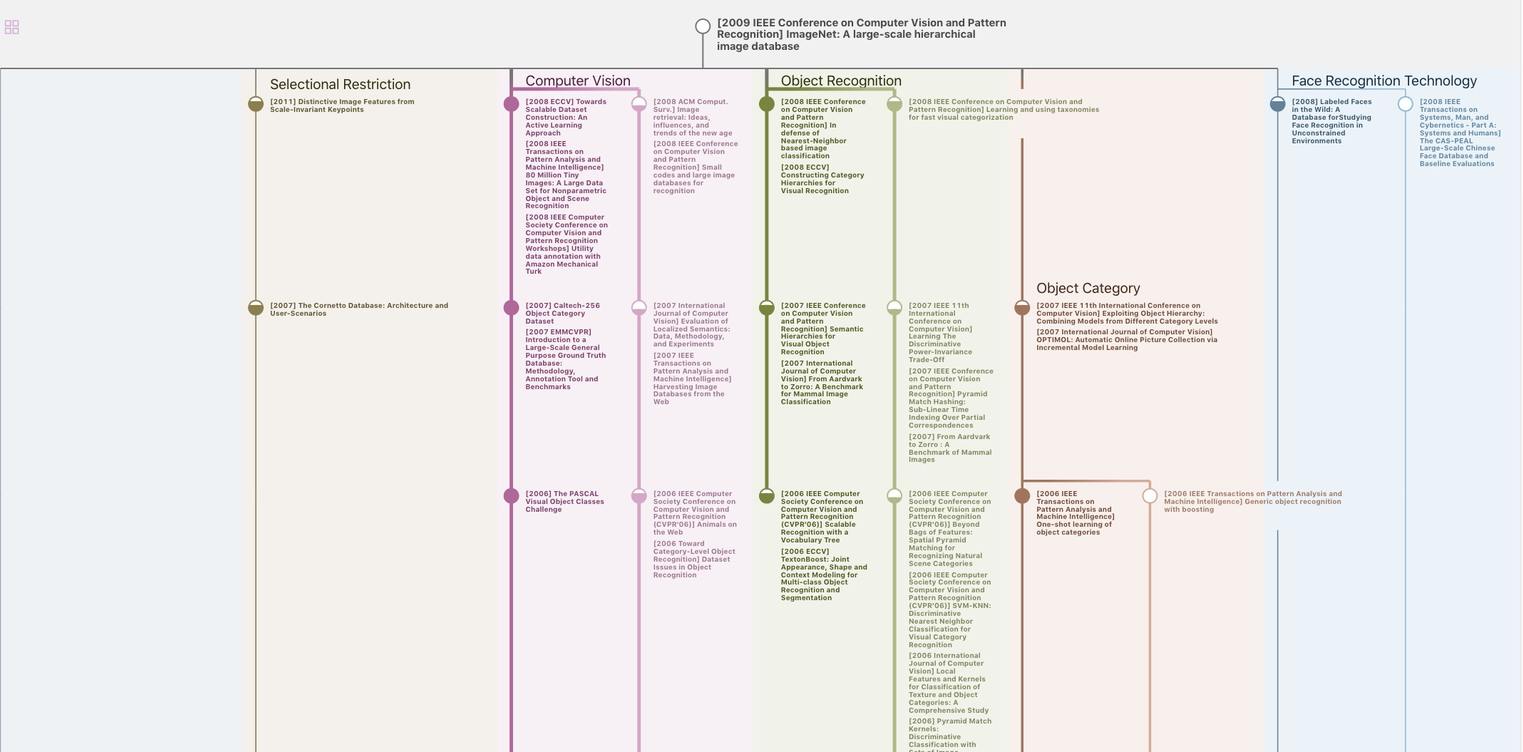
生成溯源树,研究论文发展脉络
Chat Paper
正在生成论文摘要