Scdac: Deep Adaptive Clustering of Single-Cell Transcriptomic Data with Coupled Autoencoder and Dirichlet Process Mixture Model
Bioinformatics (Oxford, England)(2024)
摘要
Motivation Clustering analysis for single-cell RNA sequencing (scRNA-seq) data is an important step in revealing cellular heterogeneity. Many clustering methods have been proposed to discover heterogenous cell types from scRNA-seq data. However, adaptive clustering with accurate cluster number reflecting intrinsic biology nature from large-scale scRNA-seq data remains quite challenging.Results Here, we propose a single-cell Deep Adaptive Clustering (scDAC) model by coupling the Autoencoder (AE) and the Dirichlet Process Mixture Model (DPMM). By jointly optimizing the model parameters of AE and DPMM, scDAC achieves adaptive clustering with accurate cluster numbers on scRNA-seq data. We verify the performance of scDAC on five subsampled datasets with different numbers of cell types and compare it with 15 widely used clustering methods across nine scRNA-seq datasets. Our results demonstrate that scDAC can adaptively find accurate numbers of cell types or subtypes and outperforms other methods. Moreover, the performance of scDAC is robust to hyperparameter changes.Availability and implementation The scDAC is implemented in Python. The source code is available at https://github.com/labomics/scDAC.
更多查看译文
关键词
Droplet-based Sequencing,Clustering
AI 理解论文
溯源树
样例
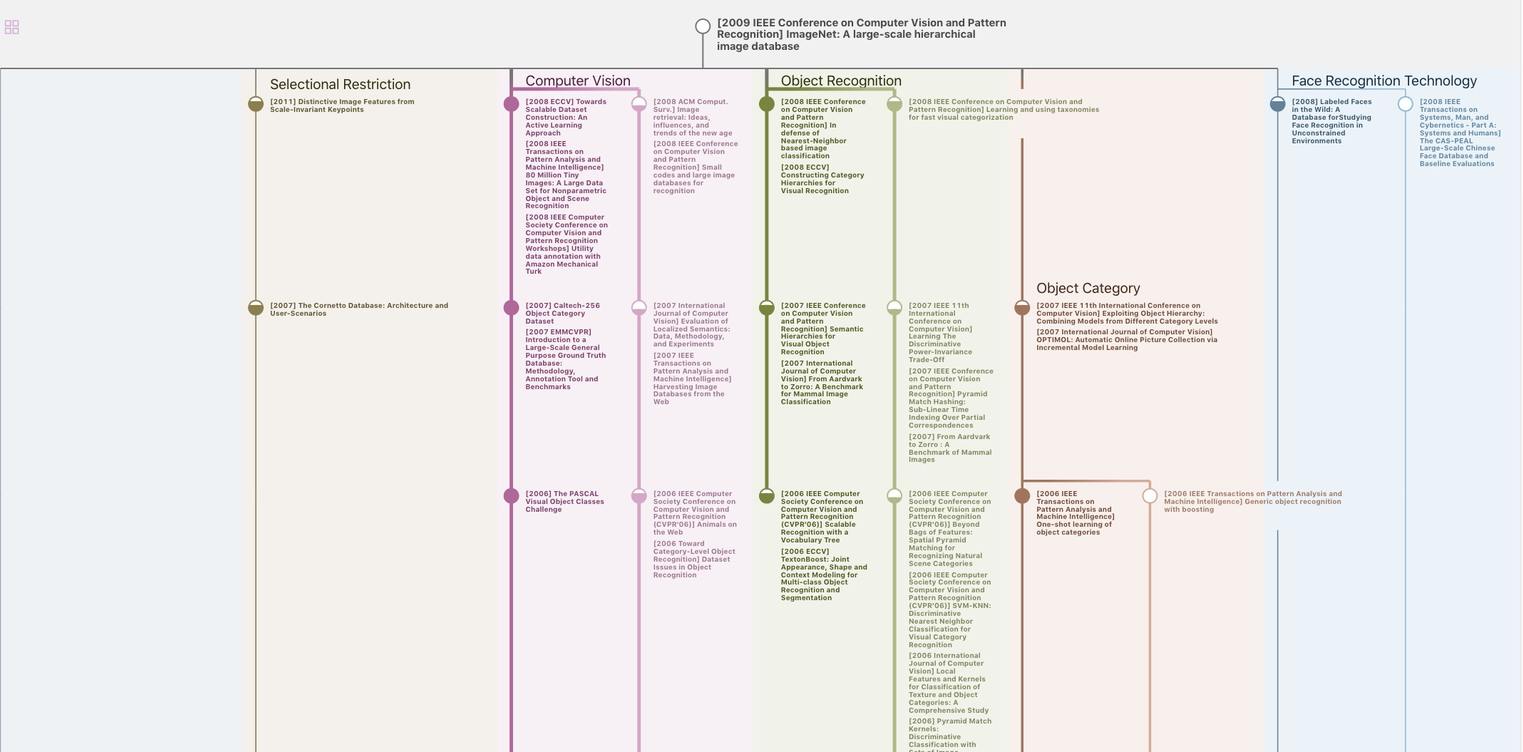
生成溯源树,研究论文发展脉络
Chat Paper
正在生成论文摘要