Deep Reinforcement Learning based Dynamic Channel Bonding for Wi-Fi Networks.
Parallel and Distributed Processing with Applications(2023)
摘要
This paper presents a decentralized Deep Reinforcement Learning (DRL)-based dynamic channel bonding (DCB) algorithm (i.e., drlDCB) for Wi-Fi networks. Most existing RL-based channel bonding algorithms either are centralized (i.e., requiring a central controller and global information for training), or can easily fall into local optimum (e.g., over-using channels for demand) as the number of stations increases. Moreover, they may converge slowly due to the use of measured throughput or delay over a duration as reward. In contrast, drlDCB is a purely distributed algorithm where each access point gets trained using local information, and addresses the local optimum and the slow convergence problems through the following new designs: 1) a new piecewise throughput model to avoid occupying too many channels if demand is satisfied, and 2) a new reward design using estimated throughput. The use of estimated throughput allows per-frame adaptation and fast convergence speed, while the accurate estimation generalizes the application scenarios, allowing an offline-trained model to be used in a dynamic environment. Simulation results in the static scenario with fixed loads and the dynamic scenario with random loads show that drlDCB outperforms state-of-the-art DCB algorithms in terms of network throughput, delay and convergence speed.
更多查看译文
关键词
Wi-Fi networks,Dynamic Channel Bonding,Channel Assignment,Deep Reinforcement Learning
AI 理解论文
溯源树
样例
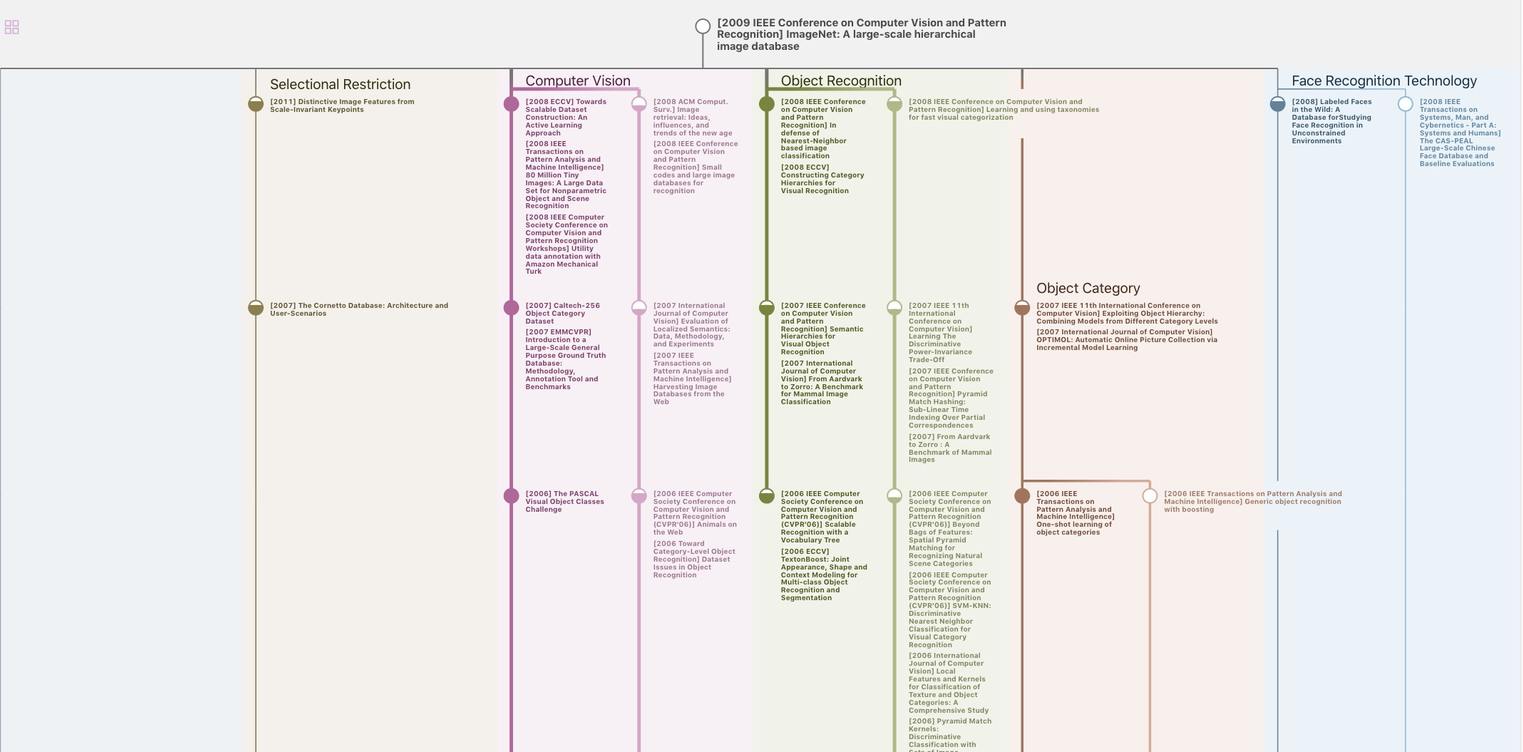
生成溯源树,研究论文发展脉络
Chat Paper
正在生成论文摘要