Brain-inspired spiking neural networks in Engineering Mechanics: a new physics-based self-learning framework for sustainable Finite Element analysis
Engineering with Computers(2024)
摘要
The present study aims to develop a sustainable framework employing brain-inspired neural networks for solving boundary value problems in Engineering Mechanics. Spiking neural networks, known as the third generation of artificial neural networks, are proposed for physics-based artificial intelligence. Accompanied by a new pseudo-explicit integration scheme based on spiking recurrent neural networks leading to a spike-based pseudo explicit integration scheme, the underlying differential equations are solved with a physics-informed strategy. We propose additionally a third-generation spike-based Legendre Memory Unit that handles large sequences. These third-generation networks can be implemented on the coming-of-age neuromorphic hardware resulting in less energy and memory consumption. The proposed framework, although implicit, is viewed as a pseudo-explicit scheme since it requires almost no or fewer online training steps to achieve a converged solution even for unseen loading sequences. The proposed framework is deployed in a Finite Element solver for plate structures undergoing cyclic loading and a Xylo-Av2 SynSense neuromorphic chip is used to assess its energy performance. An acceleration of more than 40
更多查看译文
关键词
Physics informed,Self-learning,Spiking neural network,Legendre Memory Unit,Viscoplasticity,Spike-based pseudo explicit integration scheme
AI 理解论文
溯源树
样例
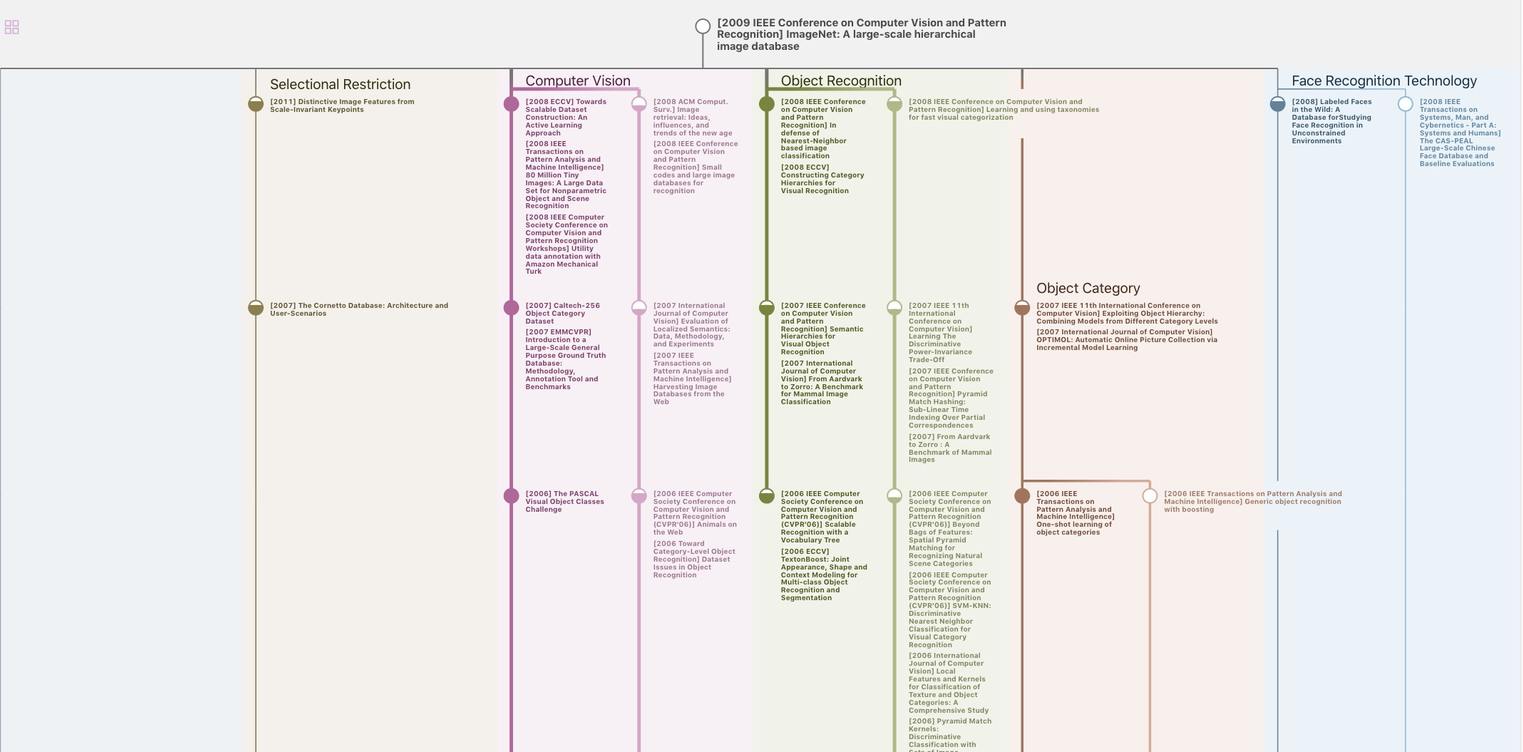
生成溯源树,研究论文发展脉络
Chat Paper
正在生成论文摘要