MLR-SimSiam: A Contrastive Pre-training Model based on Polarimetric Jittering and Mutual Learning Regularizer for PolSAR Image Classification
IEEE Geoscience and Remote Sensing Letters(2024)
摘要
Polarimetric synthetic aperture radar (PolSAR) image classification is a significant research area in the interpretation of PolSAR images. In recent years, PolSAR classification algorithms based on deep learning (DL) have been widely used, and achieved excellent performance in the case of sufficient training samples. However, due to the particularity of radar imaging, the scarcity of annotated data poses a challenge for PolSAR image classification based on DL. Contrastive learning (CL) is an effective approach to solve the problem of insufficient training samples. However, current CL methods have overlooked the utilization of the rich polarimetric information which is unique to PolSAR data. Thus, we propose a novel CL model to address the aforementioned issue. Firstly, inspired by the polarimetric scattering mechanism, the proposed method constructs hard positive samples combining polarimetric information. Then, a mutual learning regularizer (MLR) is used to design a loss function that bridges the semantic gap between different positive instances while introducing the hard positive samples. Finally, a transductive CL framework and gradient stop method are employed for pre-training, preventing model collapse and excessive computing requirements. The proposed method has been tested on two benchmark PolSAR datasets. On the same network backbone, the proposed method achieves up to 4.07% and 5.43% improvement in overall accuracy (OA) and kappa coefficient (KC), and up to 1.08% and 1.43% improvement compared to the the state-of-the-art methods. The experimental results demonstrate impressive performance on few-shot classification task of PolSAR image classification.
更多查看译文
关键词
PolSAR classification,contrastive learning,scattering mechanism,few-shot learning,mutual learning regularizer
AI 理解论文
溯源树
样例
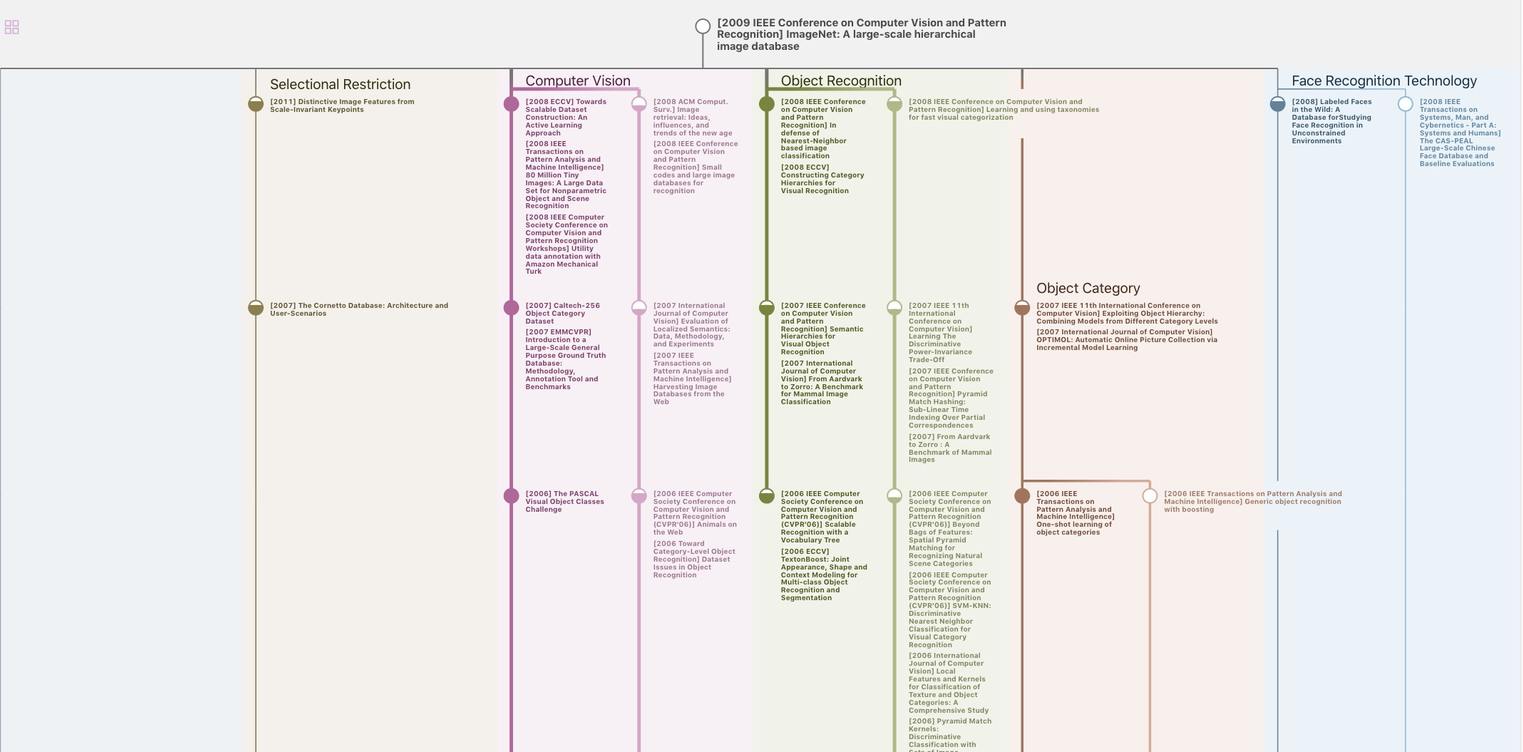
生成溯源树,研究论文发展脉络
Chat Paper
正在生成论文摘要