A simulation study of sampling in difficult settings: Statistical superiority of a little-used method
Statistical Journal of the IAOS(2024)
摘要
Taking a representative sample to determine prevalence of variables such as disease or vaccination in a population presents challenges, especially when little is known about the population. Several methods have been proposed for second stage cluster sampling. They include random sampling in small areas (the approach used in several international surveys), random walks within a specified geographic area, and using a grid superimposed on a map. We constructed 50 virtual populations with varying characteristics, such as overall prevalence of disease and variability of population density across towns. Each population comprised about a million people spread over 300 towns. We applied ten sampling methods to each. In 1,000 simulations, with different sample sizes per cluster, we estimated the prevalence of disease and the relative risk of disease given an exposure and calculated the Root Mean Squared Error (RMSE) of these estimates. We compared the sampling methods using the RMSEs. In our simulations a grid method was the best statistically in the great majority of circumstances. It showed less susceptibility to clustering effects, likely because it sampled over a much wider area than the other methods. We discuss the findings in relation to practical sampling issues.
更多查看译文
AI 理解论文
溯源树
样例
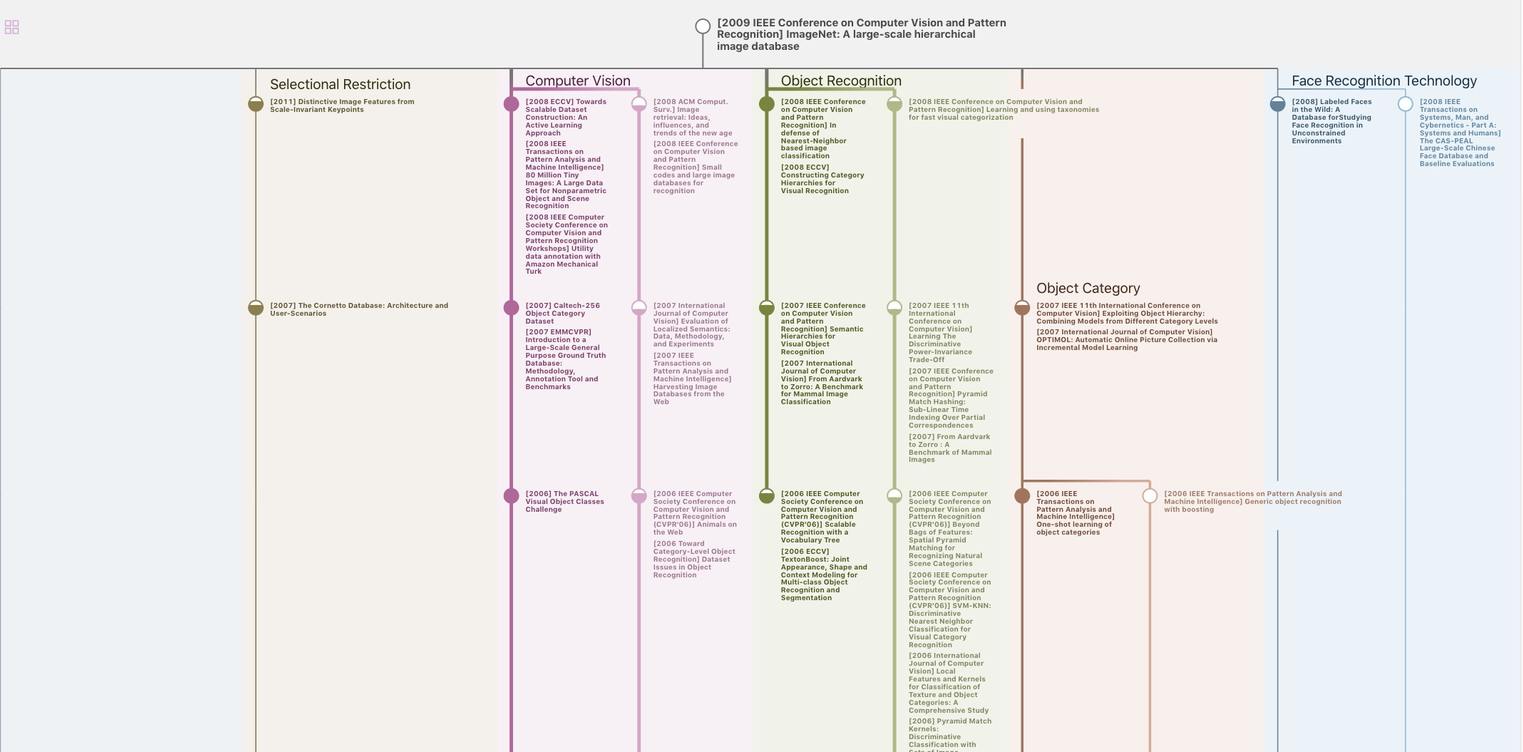
生成溯源树,研究论文发展脉络
Chat Paper
正在生成论文摘要