Adaptive Weighted Ensemble Clustering Via Kernel Learning and Local Information Preservation
Knowledge-based systems(2024)
摘要
Ensemble clustering refers to learning a robust and accurate consensus result from a collection of base clustering results. Despite extensive research on this topic, it remains challenging due to the absence of raw features and real labels of samples. Current ensemble clustering methods based on co-association (CA) matrix have received much attention from researchers. However, the information contained in the CA matrix is difficult to fully exploit within its inherent representation space. To address this issue and further unveil the underlying patterns and nonlinear relationships within the CA matrix, this paper proposes a novel Adaptive Weighted method based on Kernel learning and local information preservation for Ensemble Clustering, termed AWKEC. Unlike other ensemble clustering studies, AWKEC introduces kernel ideas to ensemble clustering to learn consistent sample relationships across multiple kernel spaces. Moreover, considering the properties of the CA matrix, highly reliable local information is preserved and embedded into the learned similarity coefficient matrices via Hadamard product. The experimental results, conducted on 13 datasets and compared to 10 representative comparative methods, validate the effectiveness of the proposed AWKEC.
更多查看译文
关键词
Ensemble clustering,Consensus clustering,Co-association matrix,Kernel learning,Local information preservation
AI 理解论文
溯源树
样例
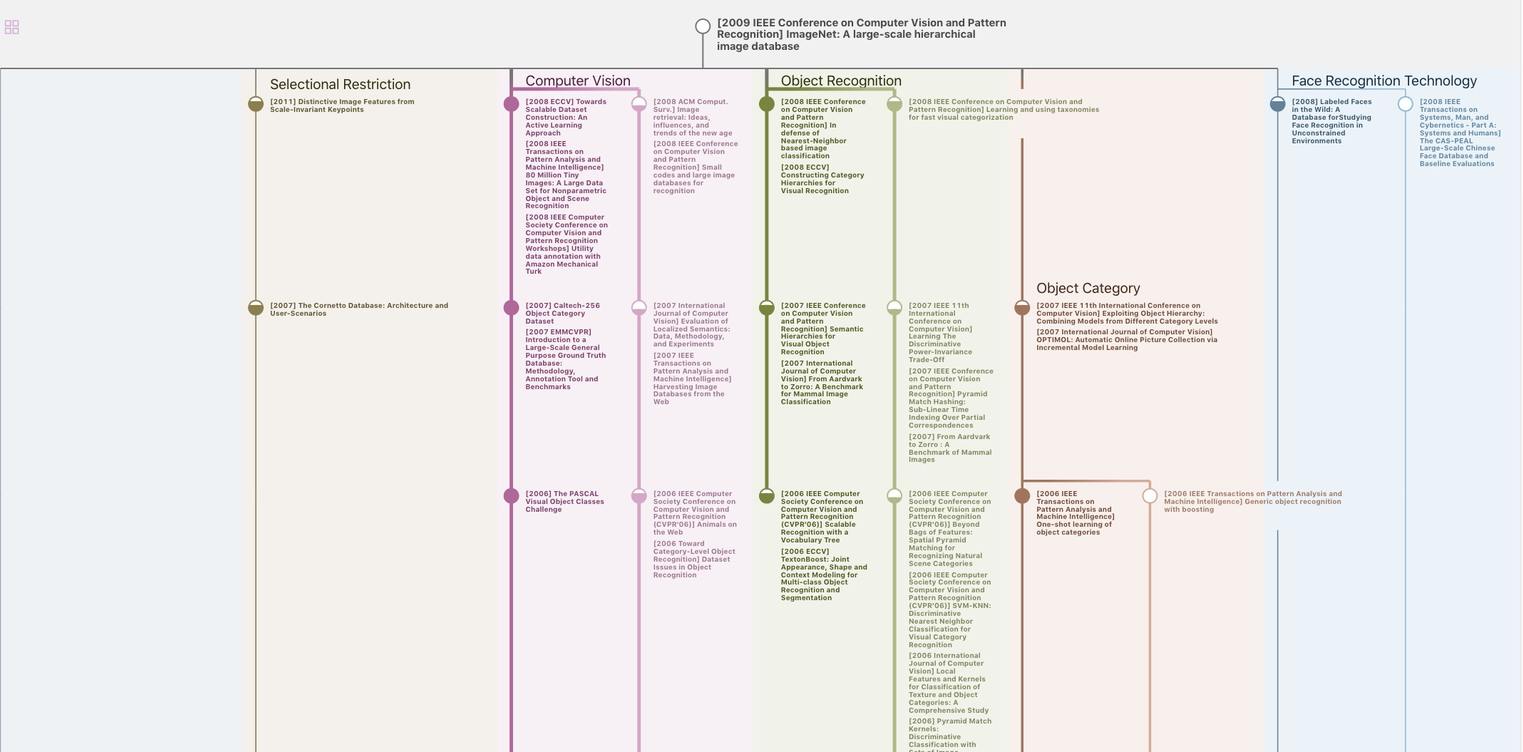
生成溯源树,研究论文发展脉络
Chat Paper
正在生成论文摘要