Explainable machine learning: Compressive strength prediction of FRP-confined concrete column
Materials Today Communications(2024)
摘要
Fibre reinforced polymers (FRP) are widely used for the strengthening of beams, slabs and columns due to their light weight and high strength. Compared to the traditional methods like steel cages or steel jackets, it reduces the time and economic cost significantly. This study focuses on the prediction of the compressive strength of FRP-confined concrete columns, which is influenced by multiple parameters. Initially, through experimental observations and the analysis of parameter mechanisms, the key parameters affecting compressive strength were identified. Six prediction models, namely linear regression, ridge regression, decision tree, random forest, and eXtreme Gradient Boosting, were subsequently constructed and evaluated to determine the optimal machine learning model. A comparison between the machine learning models and mathematical models was conducted. Furthermore, SHapley Additive exPlanation (SHAP) analysis was performed to ascertain the parameter importance and sensitivity of the machine learning model, and the analysis results were compared with existing models. The results indicate that compared to other machine learning and mathematical models, the eXtreme Gradient Boosting model demonstrates superior robustness and accuracy. The thickness and elastic modulus of FRP, as well as the concrete strength, are identified as crucial parameters that positively influence compressive strength. The relationships between the former two parameters and compressive strength follow a logarithmic function, while the relationship between concrete strength and compressive strength is approximately linear. These findings hold implications for the future development of codes and standards.
更多查看译文
关键词
Fibre reinforced polymers (FRP),Concrete column,Compressive strength,Machine learning,SHapley Additive exPlanation (SHAP)
AI 理解论文
溯源树
样例
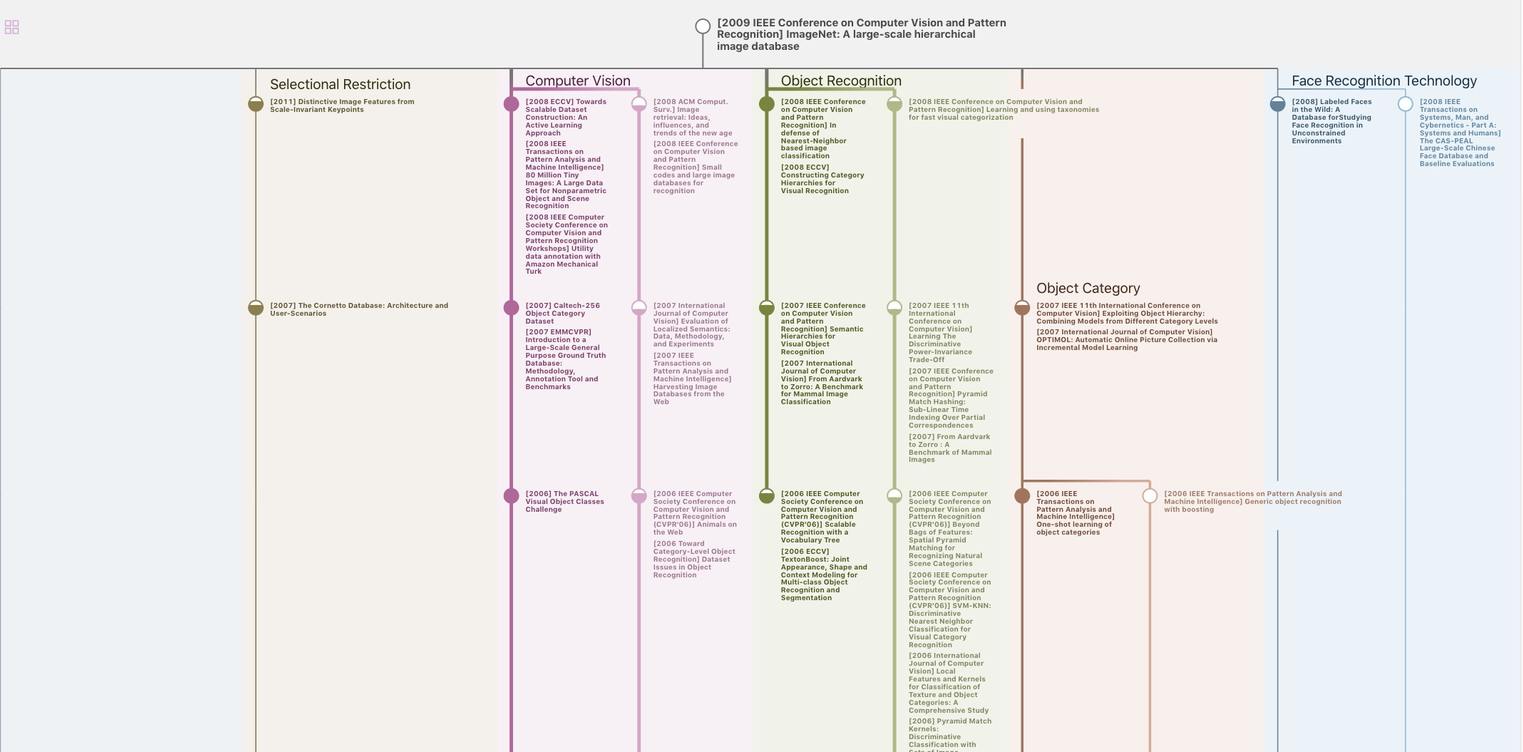
生成溯源树,研究论文发展脉络
Chat Paper
正在生成论文摘要