Predicting removal efficiency of organic pollutants by soil vapor extraction based on an optimized machine learning method
Science of The Total Environment(2024)
摘要
Soil vapor extraction (SVE) was a cost-effective technology for remediating volatile and semi-volatile organic contaminated soils. Many factors, including SVE parameters, soil properties, and contaminant characteristics, significantly influenced the remediation efficiency of SVE. The optimal conditions for organic contaminant removal efficiency were site-specific and varied among studies. Therefore, a generalized model was needed to predict the remediation efficiency in organic contaminated soils. This study employed machine learning to predict the removal efficiency of organic pollutants by SVE. The model's development was based on a trainset, and its predictive capabilities were evaluated using a testset. An XGBoost (XGB) model was derived from literature data (R2 = 0.9775). Time, pollutant type, and temperature were identified as the three most important features affecting SVE remediation efficiency. The accuracy (R2 = 0.9799) and universality of the model were enhanced through an optimization scheme. The developed XGB model demonstrated the ability to predict the removal efficiency of organic pollutants by considering all collected influential factors. The mechanism of multi-factor interaction on remediation efficiency was clarified. Overall, this study would contribute to evaluating the remediation potential of SVE for specific organic contaminated soils, aiding in maximizing the removal efficiency of organic pollutants under optimal conditions.
更多查看译文
关键词
Machine learning models,Organic pollutants,Soil vapor extraction,Soil remediation
AI 理解论文
溯源树
样例
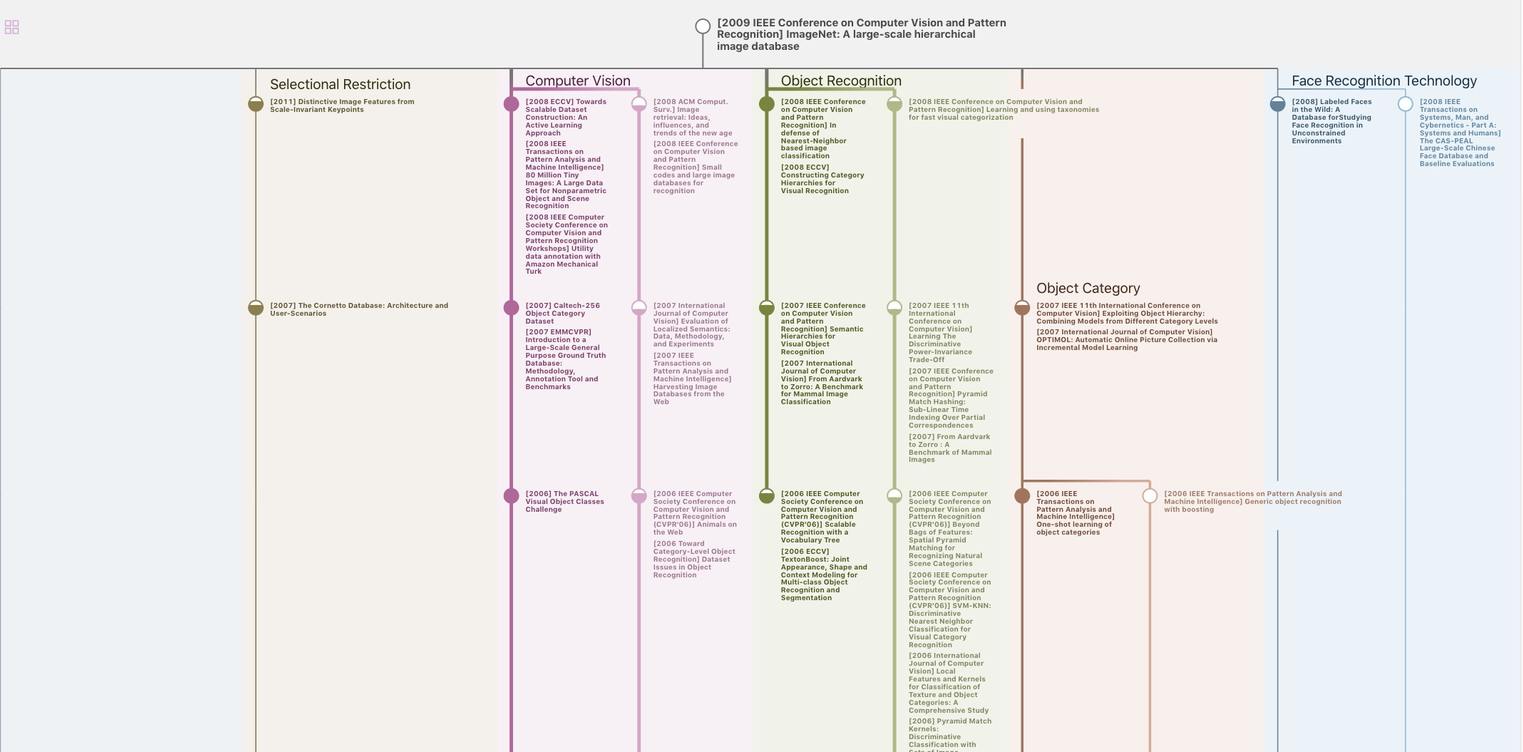
生成溯源树,研究论文发展脉络
Chat Paper
正在生成论文摘要