Multi-Representation Joint Dynamic Domain Adaptation Network for Cross-Database Facial Expression Recognition
Electronics(2024)
摘要
In order to obtain more fine-grained information from multiple sub-feature spaces for domain adaptation, this paper proposes a novel multi-representation joint dynamic domain adaptation network (MJDDAN) and applies it to achieve cross-database facial expression recognition. The MJDDAN uses a hybrid structure to extract multi-representation features and maps the original facial expression features into multiple sub-feature spaces, aligning the expression features of the source domain and target domain in multiple sub-feature spaces from different angles to extract features more comprehensively. Moreover, the MJDDAN proposes the Joint Dynamic Maximum Mean Difference (JD-MMD) model to reduce the difference in feature distribution between different subdomains by simultaneously minimizing the maximum mean difference and local maximum mean difference in each substructure. Three databases, including eNTERFACE, FABO, and RAVDESS, are used to design a large number of cross-database transfer learning facial expression recognition experiments. The accuracy of emotion recognition experiments with eNTERFACE, FABO, and RAVDESS as target domains reach 53.64%, 43.66%, and 35.87%, respectively. Compared to the best comparison method chosen in this article, the accuracy rates were improved by 1.79%, 0.85%, and 1.02%, respectively.
更多查看译文
关键词
transfer learning,facial expression recognition,multi-representation joint dynamic domain adaptation network,Joint Dynamic Maximum Mean Difference
AI 理解论文
溯源树
样例
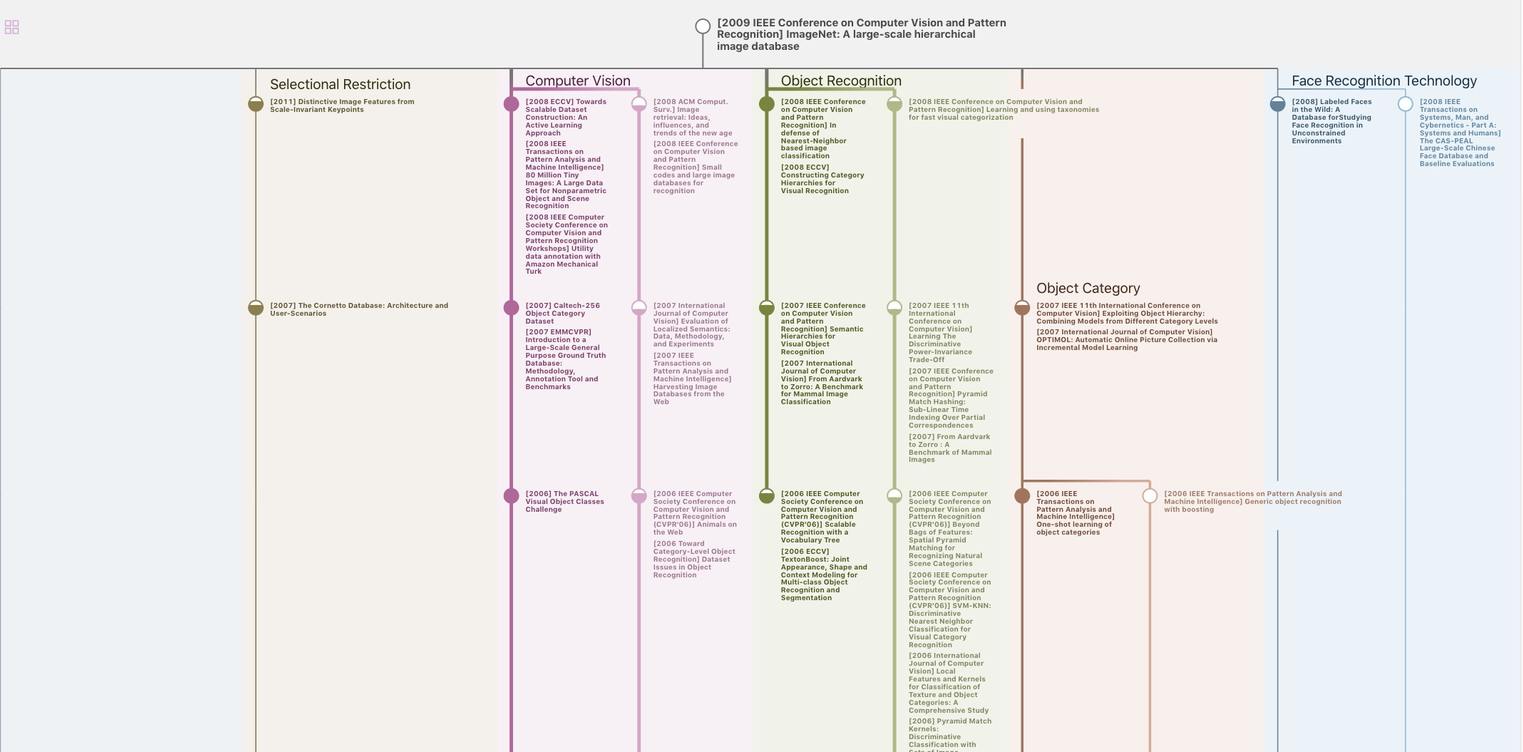
生成溯源树,研究论文发展脉络
Chat Paper
正在生成论文摘要