Collaborative Learning-Based Modulation Recognition for 6 G Multibeam Satellite Communication Systems Via Blind and Semi- Blind Channel Equalization
IEEE Transactions on Aerospace and Electronic Systems(2024)
摘要
Blind modulation recognition (BMR) involves identifying the modulation scheme of intercepted signals, an essential component of terrestrial radio management. While deep learning (DL) has advanced BMR research in terrestrial contexts, 6 G multi-beam mobile satellite (MMS) systems present many challenges. One pivotal hurdle is the reliance on perfect channel-state information (CSI) for signal equalization in terrestrial MIMO BMR literature. In MMS systems, the vast satellite-ground distance can lead to outdated CSI, making traditional equalization techniques less effective. Addressing this, we introduce a viable BMR algorithm that leverages blind and semi- blind channel equalization to overcome challenges like inter-beam interference (IBI), limited CSI, and Shadowed-Rician (SR) fading. By transforming the MMS system into an equivalent MIMO satellite system, we enable enhanced MIMO channel equalization to counteract IBI and SR attenuations. Our proposed locality-globality convolutional-transformer deep neural network (LG-CTDNet) merges the strengths of CNNs in local feature extraction with Transformers in global feature discernment. A decision fusion strategy is then incorporated to utilize cooperative diversity across multiple eavesdroppers. Numerical tests validate the effectiveness of our blind and semi- blind equalization techniques, emphasizing their superiority over existing DL-based methods in satellite modulation signal identification even in conditions with limited or absent CSI.
更多查看译文
关键词
6 G multi-beam satellite system,blind and semi- blind equalization,inter-beam interference,shadowed-rician fading channel,modulation recognition
AI 理解论文
溯源树
样例
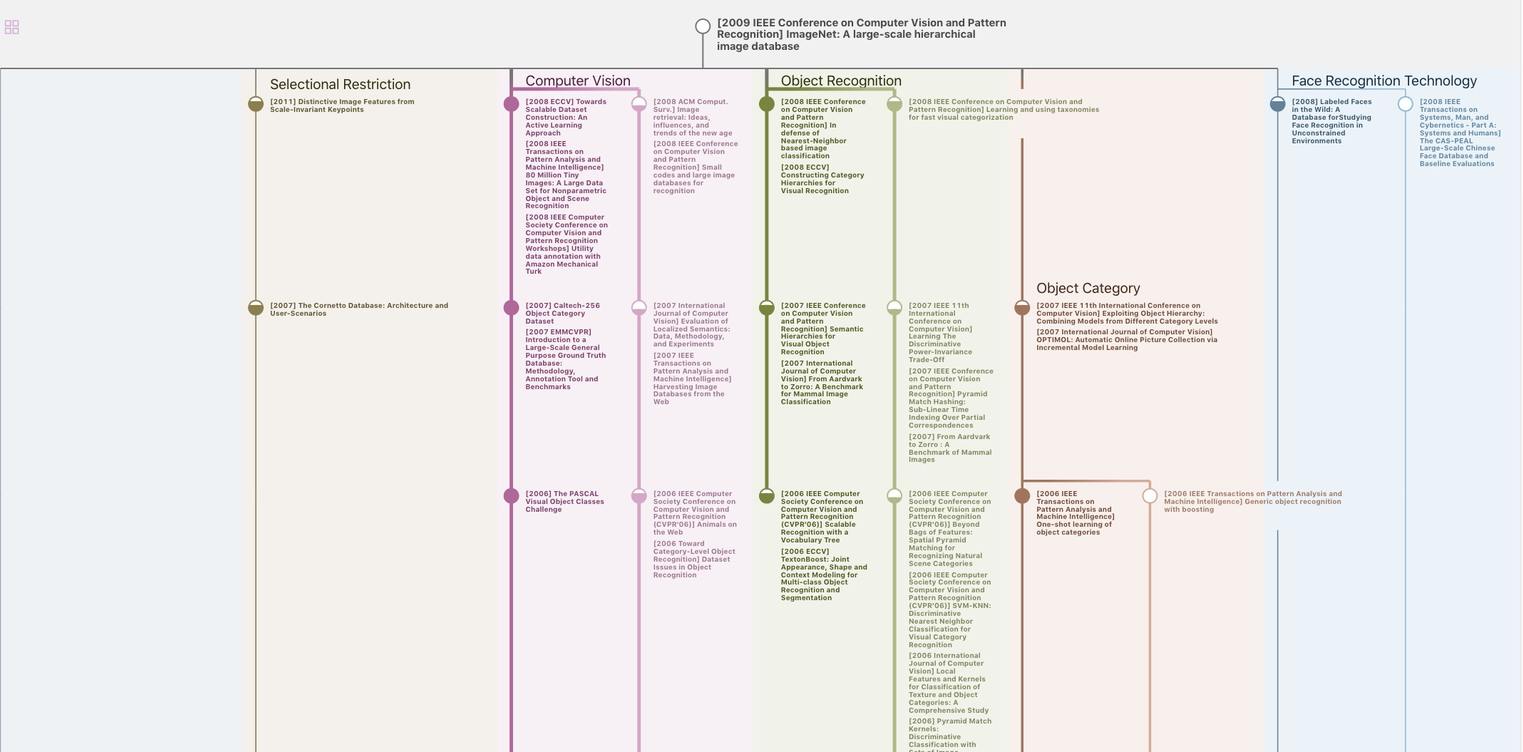
生成溯源树,研究论文发展脉络
Chat Paper
正在生成论文摘要