Evaluating biomedical feature fusion on machine learning’s predictability and interpretability of COVID-19 severity types
medrxiv(2024)
摘要
Background Accurately differentiating severe from non-severe COVID-19 clinical types is critical for the healthcare system to optimize workflow, as severe patients require intensive care. Current techniques lack the ability to accurately predict COVID-19 patients’ clinical type, especially as SARS-CoV-2 continues to mutate.
Objective In this work, we explore both predictability and interpretability of multiple state-of-the-art machine learning (ML) techniques trained and tested under different biomedical data types and COVID-19 variants.
Methods Comprehensive patient-level data were collected from 362 patients (214 severe, 148 non-severe) with the original SARS-CoV-2 variant in 2020 and 1000 patients (500 severe, 500 non-severe) with the Omicron variant in 2022-2023. The data included 26 biochemical features from blood testing and 26 clinical features from each patient’s clinical characteristics and medical history. Different types of ML techniques, including penalized logistic regression (LR), random forest (RF), k -nearest neighbors (kNN), and support vector machines (SVM) were applied to build predictive models based on each data modality separately and together for each variant set.
Results All ML models performed similarly under different testing scenarios. The fused characteristic modality yielded the highest area under the curve (AUC) score achieving 0.914 on average. The second highest AUC was 0.876 achieved by the biochemical modality alone, followed by 0.825 achieved by clinical modality alone. All ML models were robust when cross-tested with original and Omicron variant patient data. Upon model interpretation, our models ranked elevated d-dimer (biochemical feature), elevated high sensitivity troponin I (biochemical feature), and age greater than 55 years (clinical feature) as the most predictive features of severe COVID-19.
Conclusions We found ML to be a powerful tool for predicting severe COVID-19 based on comprehensive individual patient-level data. Further, ML models trained on the biochemical and clinical modalities together witness enhanced predictive power. The improved performance of these ML models when trained and cross-tested with Omicron variant data supports the robustness of ML as a tool for clinical decision support.
### Competing Interest Statement
The authors have declared no competing interest.
### Funding Statement
This study was funded by the U.S. Centers for Disease Control and Prevention grant U01CK000677 Building Mathematical Modeling Workforce Capacity to Support Infectious Disease and Healthcare Research (HIRe Modeling Fellowship).
### Author Declarations
I confirm all relevant ethical guidelines have been followed, and any necessary IRB and/or ethics committee approvals have been obtained.
Yes
The details of the IRB/oversight body that provided approval or exemption for the research described are given below:
An institutional review board (IRB) of Wuhan Union Hospital, Tongji College of Medicine, Huazhong University of Science and Technology gave ethical approval of this work. (IRB approval #IEC-J-345)
I confirm that all necessary patient/participant consent has been obtained and the appropriate institutional forms have been archived, and that any patient/participant/sample identifiers included were not known to anyone (e.g., hospital staff, patients or participants themselves) outside the research group so cannot be used to identify individuals.
Yes
I understand that all clinical trials and any other prospective interventional studies must be registered with an ICMJE-approved registry, such as ClinicalTrials.gov. I confirm that any such study reported in the manuscript has been registered and the trial registration ID is provided (note: if posting a prospective study registered retrospectively, please provide a statement in the trial ID field explaining why the study was not registered in advance).
Yes
I have followed all appropriate research reporting guidelines, such as any relevant EQUATOR Network research reporting checklist(s) and other pertinent material, if applicable.
Yes
All data produced are available online at
更多查看译文
AI 理解论文
溯源树
样例
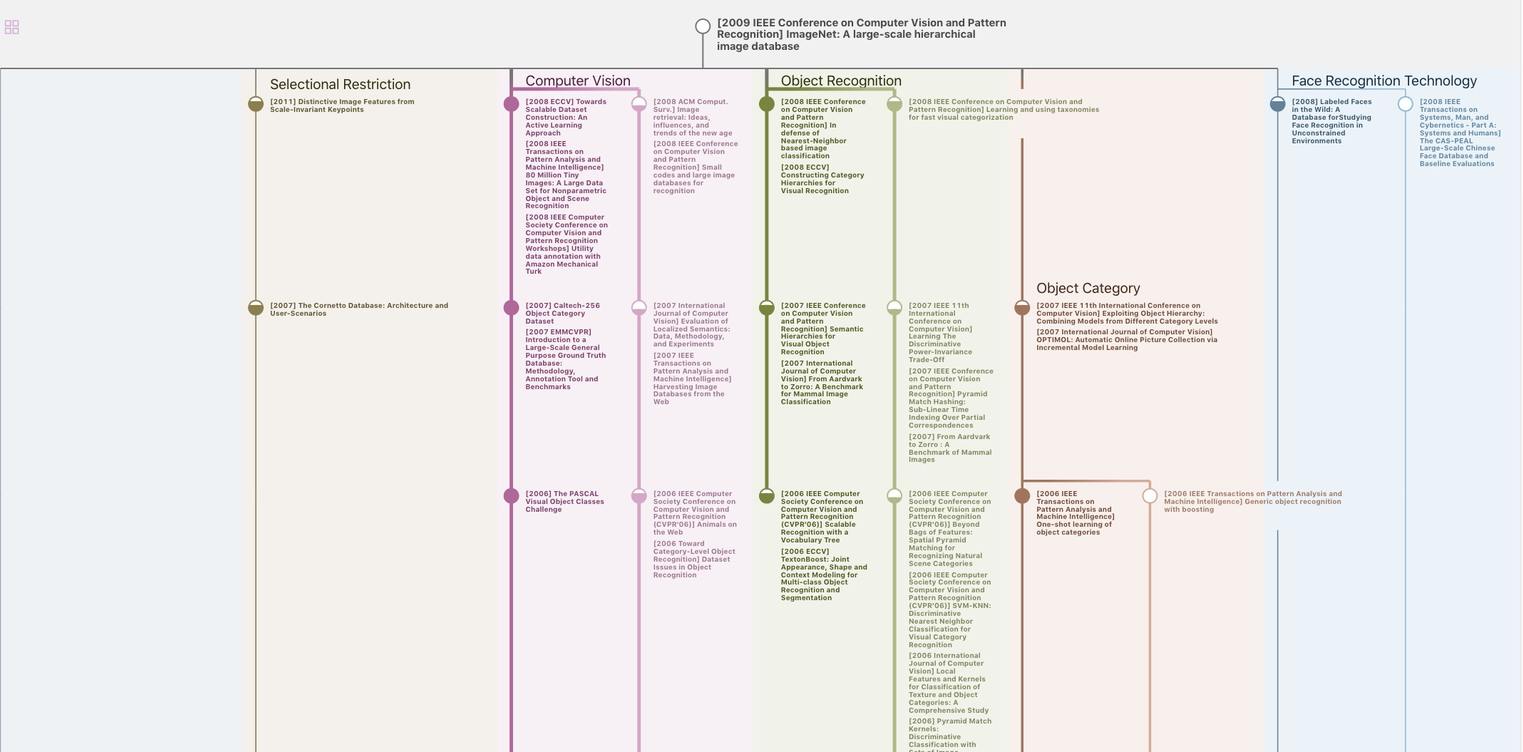
生成溯源树,研究论文发展脉络
Chat Paper
正在生成论文摘要