AHFormer: Hypergraph embedding coding transformer and adaptive aggregation network for intelligent fault diagnosis under noise interference
Advanced Engineering Informatics(2024)
摘要
Recently, convolutional neural network-based methods are widely employed in the field of fault diagnosis to capture feature information from samples. However, during the process of device signal acquisition, external strong noise interference can result in a superimposed state of collected signals and noise, making it difficult to distinguish between them. This leads to a significant decline in the performance of diagnostic models. To improve fault diagnosis accuracy under conditions of strong noise interference, we propose a method called AHFormer, which is based on hypergraph embedding encoded Transformer and adaptive information fusion. AHFormer extracts and integrates both local and global features, thus enhancing the preservation of structural and semantic information throughout the network evolution process. In specific terms, we first encode the sample signals into hypergraph structures to utilize hyperedges for capturing higher-order correlations within the samples. Subsequently, we employ the filtering properties of hypergraph convolution to perform secondary denoising on the samples. Concurrently, we utilize a message-passing mechanism to aggregate local information within the samples, enhancing the complementarity among different pieces of information within the sample signals. Next, an improved Transformer employs to extract the global information from the samples. Finally, to effectively utilize semantic information with different characteristics, we have designed an adaptive information aggregation module. We conduct Case studies, ablation experiments, and robustness analyzes on datasets of different sizes. The experimental results show that under Gaussian noise, impulse noise and stochastic masking interference, the accuracy of AHFormer method is as high as 98.88%, 98.78% and 91.14%, respectively, which is much higher than other baseline methods. In the quantitative evaluation of features, the J value is increased by an average of 35.9% compared with the best method, showing excellent anti-interference and robustness.
更多查看译文
关键词
Adaptive learning,Fault diagnosis,Hypergraph neural networks,Transformer
AI 理解论文
溯源树
样例
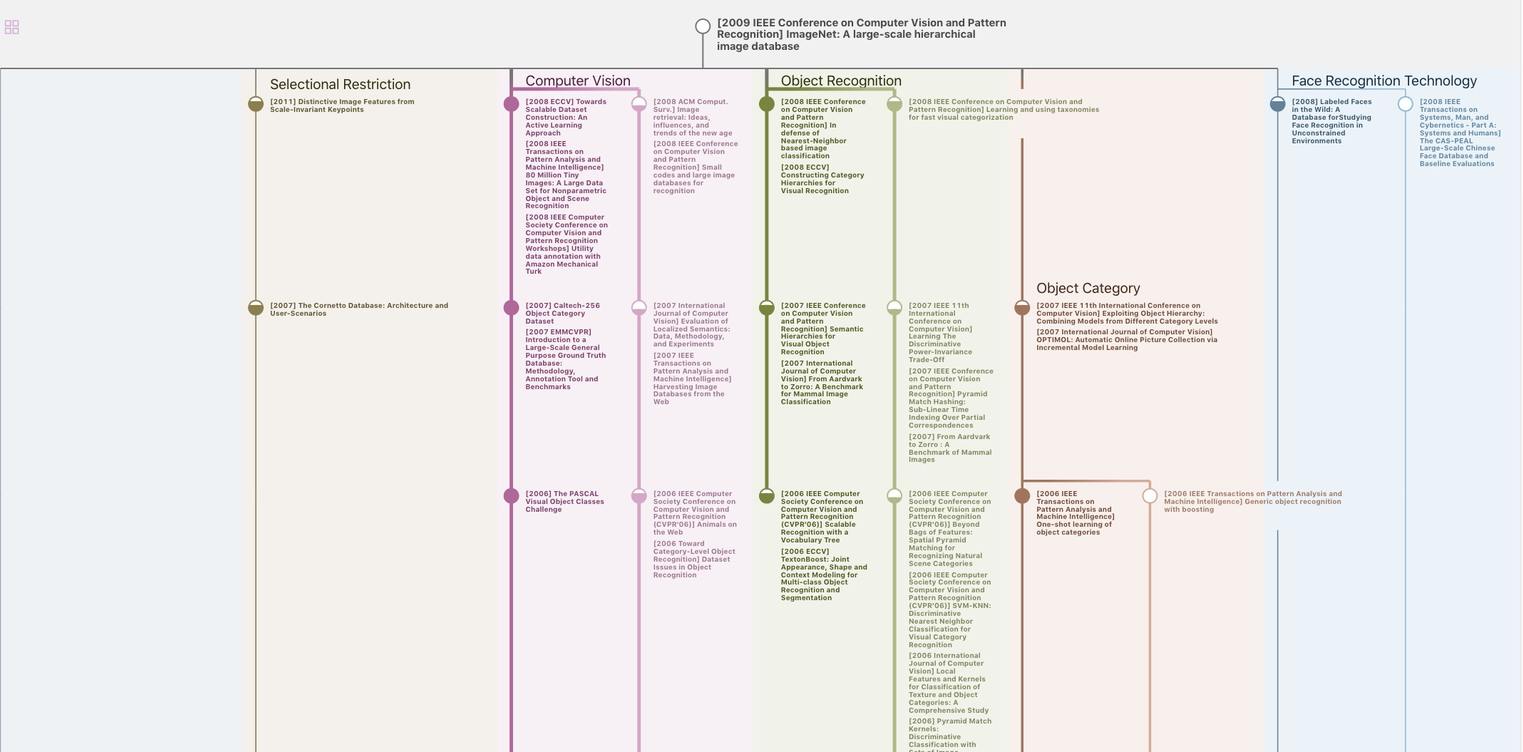
生成溯源树,研究论文发展脉络
Chat Paper
正在生成论文摘要