Predicting involuntary admission following inpatient psychiatric treatment using machine learning trained on electronic health record data
crossref(2024)
摘要
Background Involuntary admissions to psychiatric hospitals are on the rise. If patients at elevated risk of involuntary admission could be identified, prevention may be possible. Objectives To develop and validate a prediction model for involuntary admission of patients receiving care within a psychiatric service system using machine learning trained on routine clinical data from electronic health records (EHRs). Methods EHR data from all adult patients who had been in contact with the Psychiatric Services of the Central Denmark Region between 2013 and 2021 were retrieved. We derived 694 patient predictors (covering e.g., diagnoses, medication, and coercive measures) and 1,134 predictors from free text using term frequency - inverse document frequency and sentence transformers. At every voluntary inpatient discharge (prediction time), without an involuntary admission in the two years prior, we predicted involuntary admission 180 days ahead. XGBoost and Elastic Net regularized logistic regression models were trained on 85% of the dataset. The best performing model was tested on the remaining 15% of the data. Results The model was trained on 50,634 voluntary inpatient discharges among 17,968 unique patients. The cohort comprised 1,672 voluntary inpatient discharges followed by an involuntary admission. The XGBoost model performed best in the training phase and obtained an area under the receiver operating curve of 0.84 in the test phase. Conclusion A machine learning model using routine clinical EHR data can accurately predict involuntary admission. If implemented as a clinical decision support tool, this model may guide interventions aimed at reducing the risk of involuntary admission.
### Competing Interest Statement
AAD has received a speaker honorarium from Otsuka Pharmaceutical. SDØ received the 2020 Lundbeck Foundation Young Investigator Prize and SDØ owns/has owned units of mutual funds with stock tickers DKIGI, IAIMWC, SPIC25KL and WEKAFKI, and owns/has owned units of exchange traded funds with stock tickers BATE, TRET, QDV5, QDVH, QDVE, SADM, IQQH, IQQJ, USPY, EXH2, 2B76, IS4S, OM3X and EUNL.
### Funding Statement
The study is supported by grants to SDØ from the Lundbeck Foundation (grant number: R344- 2020-1073), the Central Denmark Region Fund for Strengthening of Health Science (grant number: 1-36-72-4-20) and the Danish Agency for Digitisation Investment Fund for New Technologies (grant number 2020-6720). SDØ has received grants for other purposes from the Novo Nordisk Foundation (grant number: NNF20SA0062874), the Danish Cancer Society (grant number: R283- A16461), the Lundbeck Foundation (grant number: R358-2020-2341) and Independent Research Fund Denmark (grant numbers: 7016-00048B and 2096-00055A). These funders had no role in the study design, data analysis, interpretation of data, or writing of the manuscript.
### Author Declarations
I confirm all relevant ethical guidelines have been followed, and any necessary IRB and/or ethics committee approvals have been obtained.
Yes
The details of the IRB/oversight body that provided approval or exemption for the research described are given below:
The study was approved by the Legal Office of the Central Denmark Region in accordance with the Danish Health Care Act section 46, paragraph 2. The Danish Committee Act exempts studies based only on EHR data from ethical review board assessment (waiver for this project: 1-10-72-1-22). Handling and storage of data complied with the European Union General Data Protection Regulation. The project is registered on the list of research projects having the Central Denmark Region as data steward.
I confirm that all necessary patient/participant consent has been obtained and the appropriate institutional forms have been archived, and that any patient/participant/sample identifiers included were not known to anyone (e.g., hospital staff, patients or participants themselves) outside the research group so cannot be used to identify individuals.
Yes
I understand that all clinical trials and any other prospective interventional studies must be registered with an ICMJE-approved registry, such as ClinicalTrials.gov. I confirm that any such study reported in the manuscript has been registered and the trial registration ID is provided (note: if posting a prospective study registered retrospectively, please provide a statement in the trial ID field explaining why the study was not registered in advance).
Yes
I have followed all appropriate research reporting guidelines, such as any relevant EQUATOR Network research reporting checklist(s) and other pertinent material, if applicable.
Yes
The data used for this study cannot be shared according to Danish Law as it can be used to identify individual patients.
更多查看译文
AI 理解论文
溯源树
样例
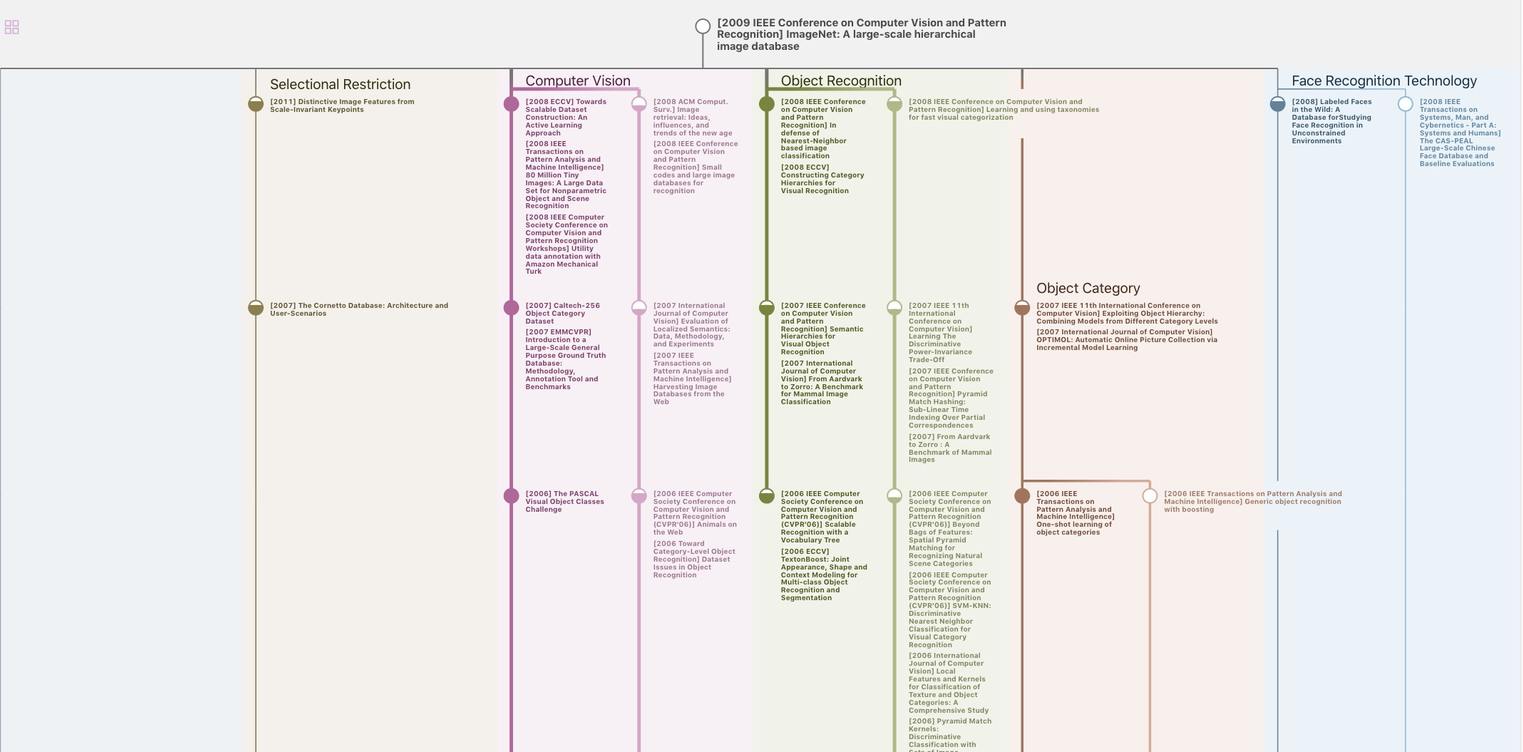
生成溯源树,研究论文发展脉络
Chat Paper
正在生成论文摘要