A psychopathological theory-driven early relapse prediction model using speech and language in psychosis
crossref(2024)
摘要
Language-based prediction of psychotic relapse using speech-derived markers offers a promising avenue for targeted prevention in early intervention. However, the sheer number of speech markers and the 'black box' nature of predictive models present significant challenges for clinical translation. Successful real-world predictions require the underlying model to be a ‘reasonable facsimile’ of the clinical phenomena that map on the predicted outcomes. We propose a theory-driven approach drawing on the notion that the predictors of early psychotic relapse should mark (1) the presence of schizophrenia in its early stages, and (2) track the positive dimension of formal thought disorder (positive FTD or disorganization) in psychosis. By leveraging Natural Language Processing (NLP) methods, we derive 3 lexical, syntactic and narrative style markers - semantic similarity, clause complexity, and analytic thinking index. We adopt a prospective design, acquiring speech samples during the acute phase of psychosis (n=68) and following them through remission and subsequent relapses (hospitalizations). With a Bayesian analysis framework, we demonstrate the utility of clinical theory-guided variable selection (disorganization and clause complexity in particular) in improving the predictive accuracy of speech-based models. The psychopathological theory-based speech-NLP model performed better than and provided incremental value to the clinical intuition of expecting a poorly functioning, cannabis-using man with educational deficits to show an early relapse. The findings underscore the potential of automated speech analysis in early relapse prediction during the initial phase of psychosis intervention.
更多查看译文
AI 理解论文
溯源树
样例
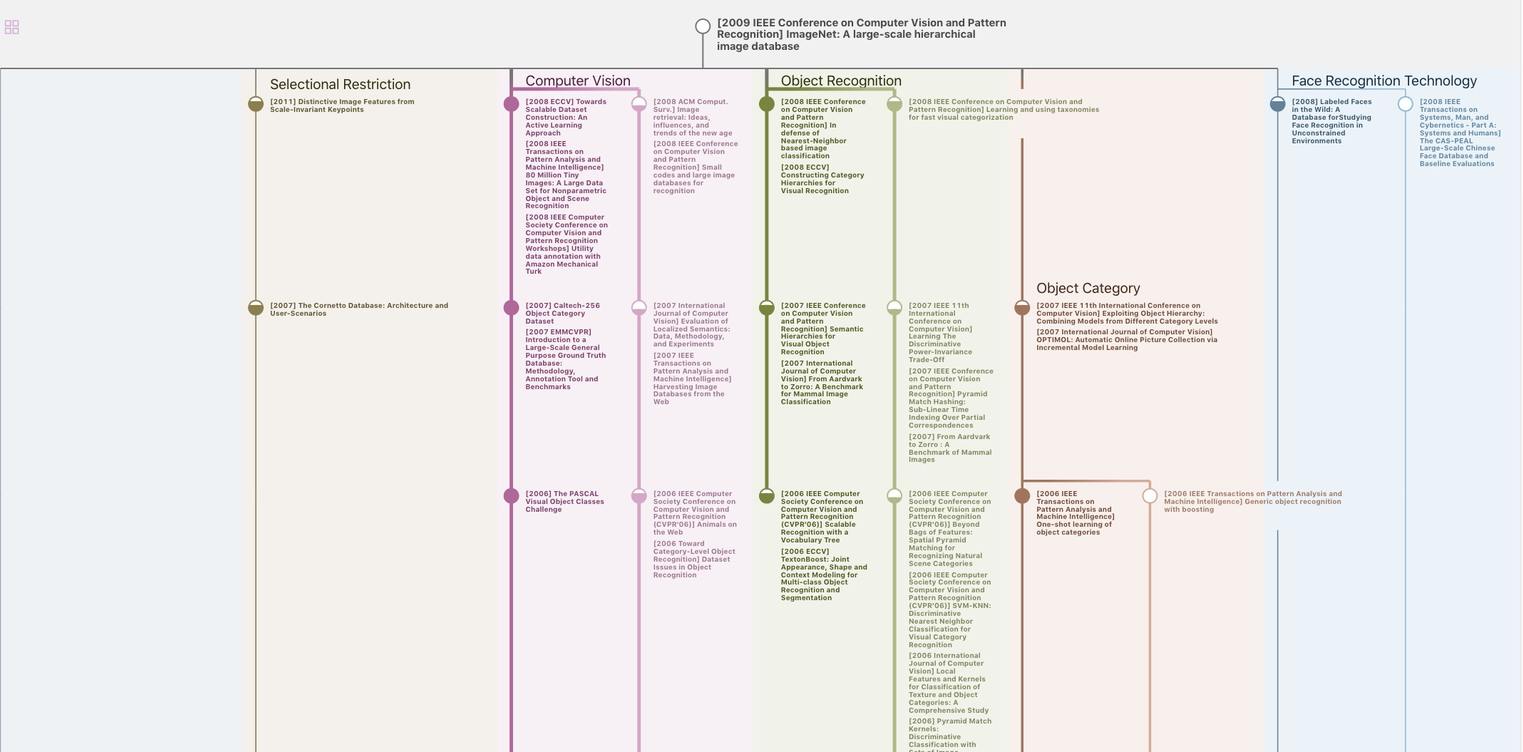
生成溯源树,研究论文发展脉络
Chat Paper
正在生成论文摘要