Improved hierarchical Bayesian modeling framework with arbitrary polynomial chaos for probabilistic model updating
Mechanical Systems and Signal Processing(2024)
摘要
Bayesian finite element model updating techniques have found widespread application in the structural health monitoring. The conventional Bayesian modeling framework (CBMF) identifies the posterior distribution of structural parameters using data from a single experiment. However, structural parameters exhibit variability due to changes in environmental or experimental conditions, an aspect overlooked by CBMF. In recent years, the hierarchical Bayesian modeling framework (HBMF) has gradually attracted attention. This framework incorporates an additional layer of hyperparameters and utilizes multiple sets of experimental data to describe the variability in structural parameters caused by changes in experimental conditions. Unfortunately, the increase in hyperparameters and data size leads the HBMF to face the dilemma of exceedingly high computational costs. To address this issue, this study proposes an improved hierarchical Bayesian modeling framework (IHBMF). To enhance the computational efficiency of IHBMF, the importance sampling method is employed to simplify the computation of the likelihood function for hyperparameters. Subsequently, a surrogate model based on arbitrary polynomial chaos expansion is introduced to further reduce the computational cost of IHBMF. The framework's accuracy and efficiency were validated through analysis of a simply supported beam and a steel pedestrian bridge. The results demonstrate that IHBMF not only delivers a more accurate quantification of structural parameter uncertainty than CBMF but also exhibits higher computational efficiency compared to HBMF, showcasing its superior capability in structural health monitoring applications.
更多查看译文
关键词
Polynomial Chaos,Bayesian model updating,Hierarchical models,Uncertainty quantification
AI 理解论文
溯源树
样例
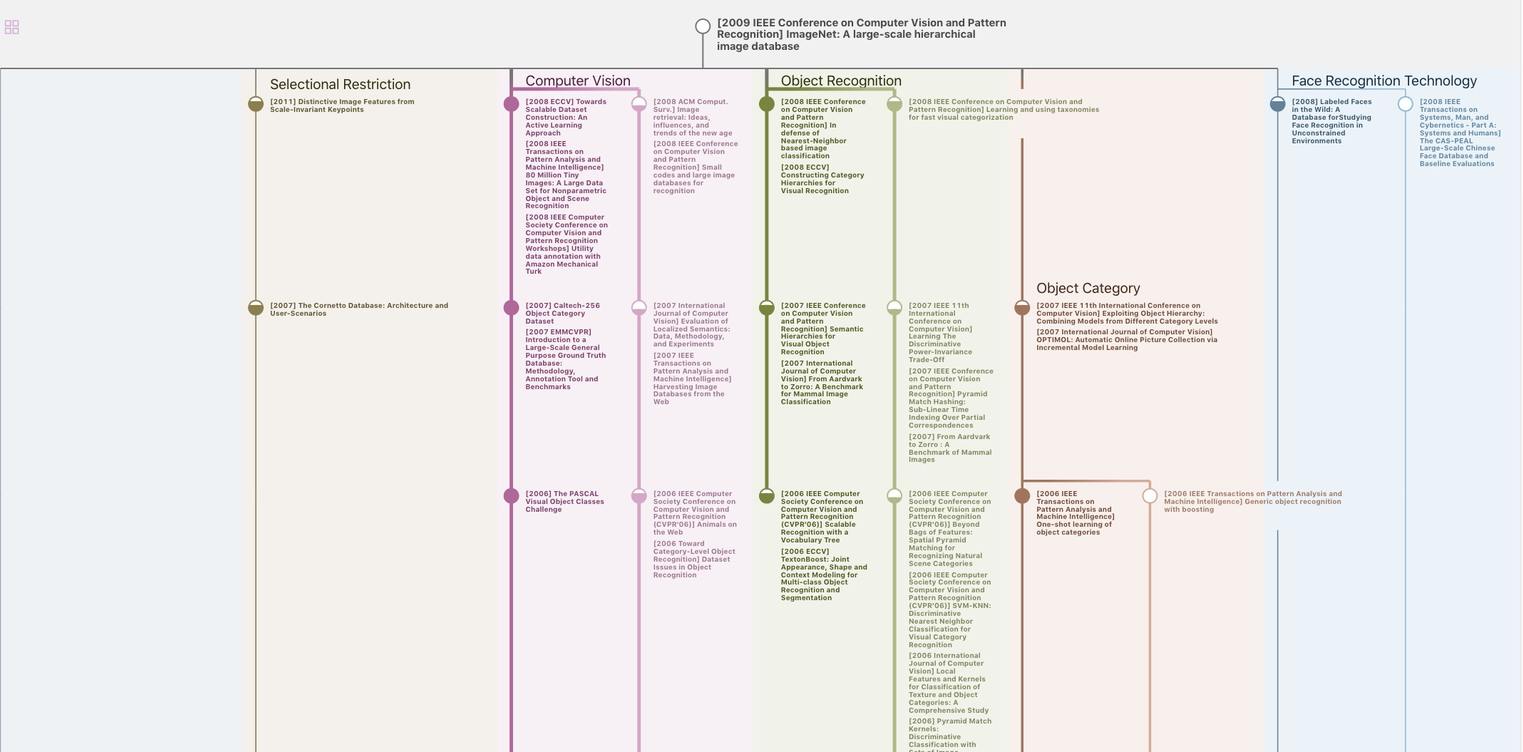
生成溯源树,研究论文发展脉络
Chat Paper
正在生成论文摘要