Learning with Noisy Correspondence
International Journal of Computer Vision(2024)
摘要
This paper studies a new learning paradigm for noisy labels, i.e., noisy correspondence (NC). Unlike the well-studied noisy labels that consider the errors in the category annotation of a sample, the NC refers to the errors in the alignment relationship of two data points. Although such false positive pairs are common especially in the data harvested from the Internet, which however are neglected by most existing works. By taking cross-modal retrieval as a showcase, we propose a method called learning with noisy correspondence (LNC). In brief, the LNC first roughly obtains the clean and noisy subsets from the original data and then rectifies the false positive pairs by using a novel adaptive prediction function. Finally, the LNC adopts a novel triplet loss with soft margins to endow cross-modal retrieval the robustness to the NC. To verify the effectiveness of the proposed LNC, we conduct experiments on six benchmark datasets in image-text and video-text retrieval tasks. Besides the effectiveness of the LNC, the experimental results show the necessity of the explicit solution to the NC faced by not only the standard model training paradigm but also the pre-training and fine-tuning paradigms.
更多查看译文
关键词
Noisy labels,Cross-modal retrieval,Multimodal learning,Misalignment
AI 理解论文
溯源树
样例
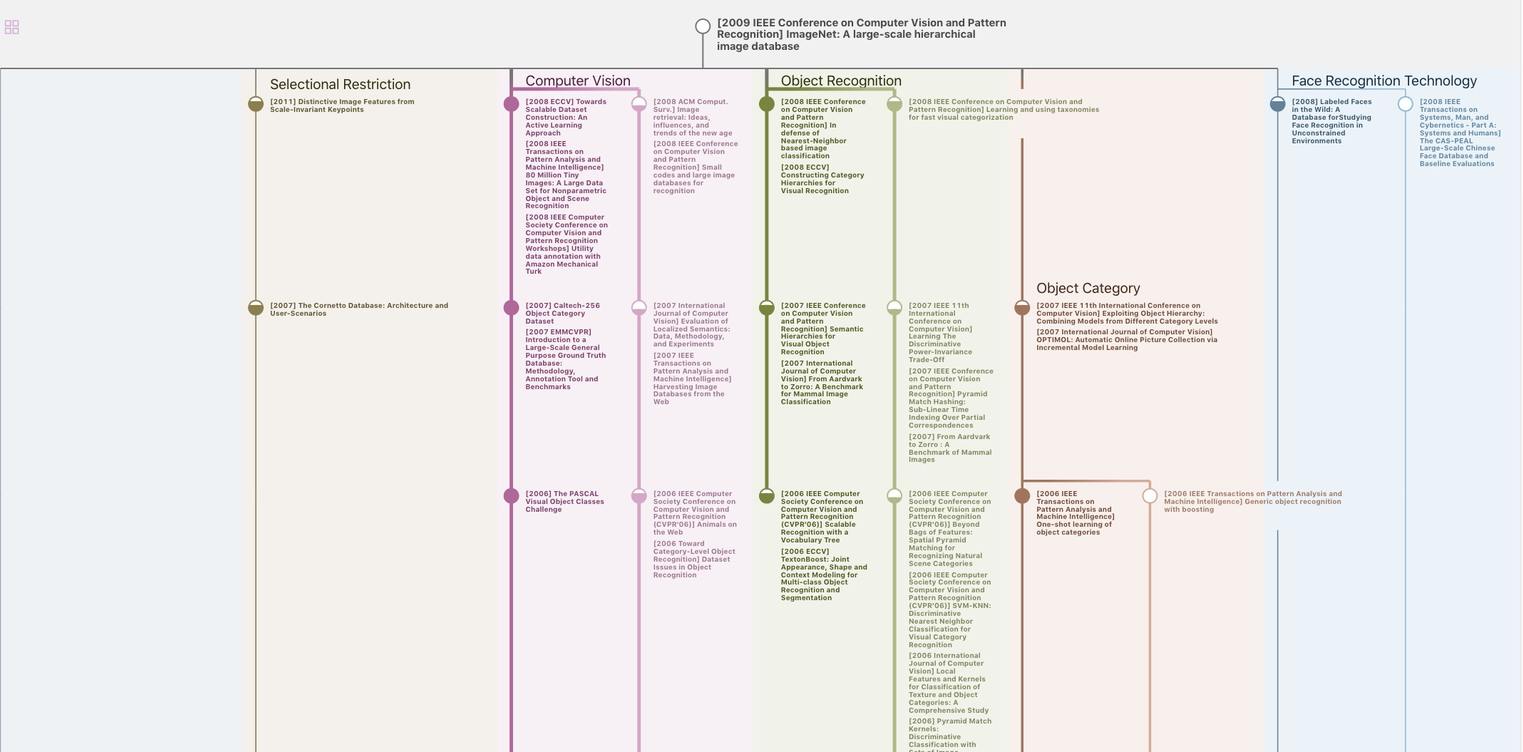
生成溯源树,研究论文发展脉络
Chat Paper
正在生成论文摘要