Transfer-learning-based representation learning for trajectory similarity search
GeoInformatica(2024)
摘要
Trajectory similarity search is one of the most fundamental tasks in spatial-temporal data analysis. Classical methods are based on predefined trajectory similarity measures, consuming high time and space costs. To accelerate similarity computation, some deep metric learning methods have recently been proposed to approximate predefined measures based on the learned representation of trajectories. However, instead of predefined measures, real applications may require personalized measures, which cannot be effectively learned by existing models due to insufficient labels. Thus, this paper proposes a transfer-learning-based model FTL-Traj, which addresses this problem by effectively transferring knowledge from several existing measures as source measures. Particularly, a ProbSparse self-attention-based GRU unit is designed to extract the spatial and structural information of each trajectory. Confronted with diverse source measures, the priority modeling assists the model for the rational ensemble. Then, sparse labels are enriched with rank knowledge and collaboration knowledge via transfer learning. Extensive experiments on two real-world datasets demonstrate the superiority of our model.
更多查看译文
关键词
Trajectory similarity,Top k similarity search,Transfer learning
AI 理解论文
溯源树
样例
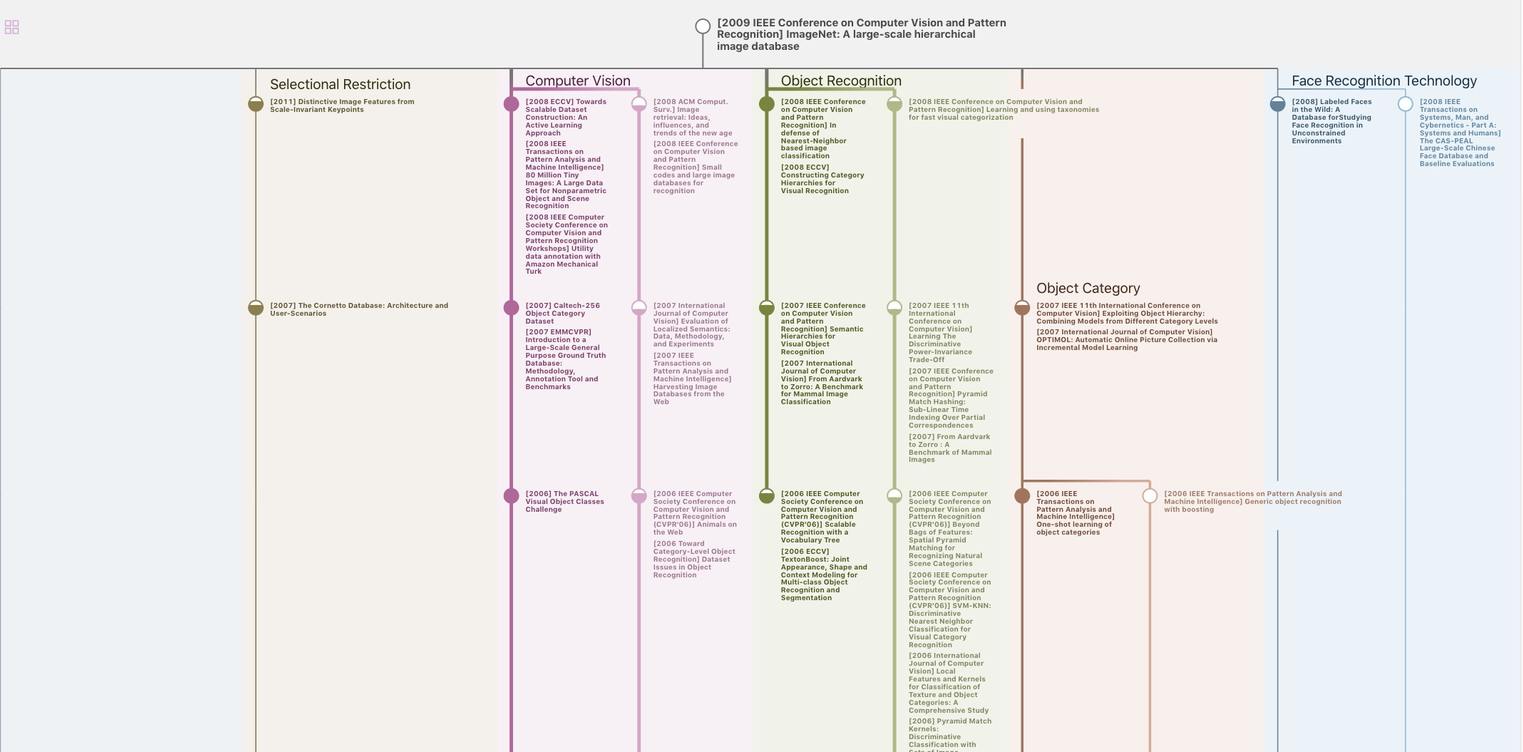
生成溯源树,研究论文发展脉络
Chat Paper
正在生成论文摘要