Statistical Complexity of Quantum Learning
ADVANCED QUANTUM TECHNOLOGIES(2024)
摘要
Learning problems involve settings in which an algorithm has to make decisions based on data, and possibly side information such as expert knowledge. This study has two main goals. First, it reviews and generalizes different results on the data and model complexity of quantum learning, where the data and/or the algorithm can be quantum, focusing on information-theoretic techniques. Second, it introduces the notion of copy complexity, which quantifies the number of copies of a quantum state required to achieve a target accuracy level. Copy complexity arises from the destructive nature of quantum measurements, which irreversibly alter the state to be processed, limiting the information that can be extracted about quantum data. As a result, empirical risk minimization is generally inapplicable. The paper presents novel results on the copy complexity for both training and testing. To make the paper self-contained and approachable by different research communities, an extensive background material is provided on classical results from statistical learning theory, as well as on the distinguishability of quantum states. Throughout, the differences between quantum and classical learning are highlighted by addressing both supervised and unsupervised learning, and extensive pointers are provided to the literature. The statistical performance of quantum learning is investigated as a function of the number of training data N$N$, and of the number of copies available for each quantum state in the training and testing data sets, respectively S$S$ and V$V$. Indeed, the biggest difference in quantum learning comes from the destructive nature of quantum measurements, which limits the amount of information that can be extracted with few copies. image
更多查看译文
关键词
information theory,quantum hypothesis testing,quantum machine learning,statistical learning theory
AI 理解论文
溯源树
样例
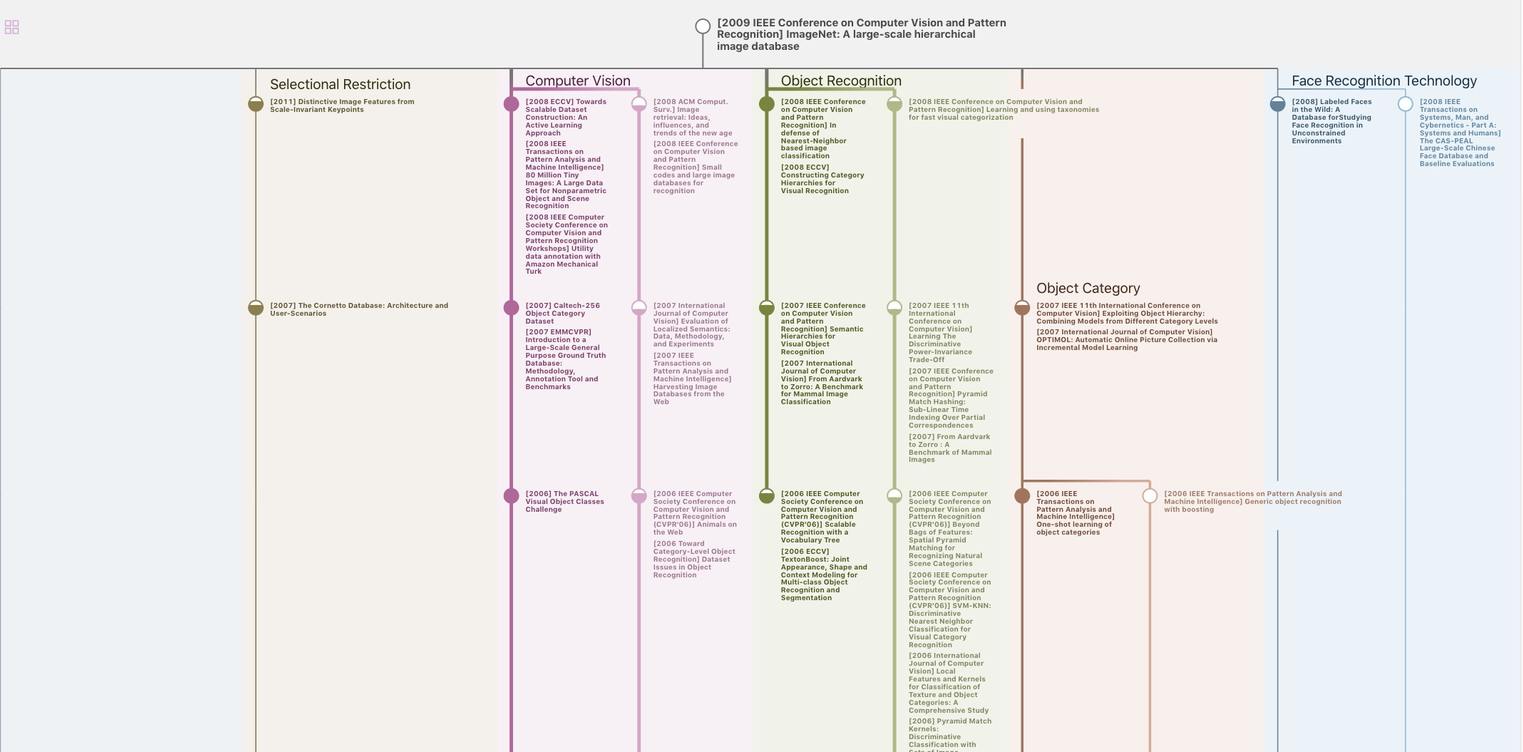
生成溯源树,研究论文发展脉络
Chat Paper
正在生成论文摘要