Evaluation of Artificial Intelligence (AI)-basedin silicotools for variant classification in clinically actionable NSCLC variants
crossref(2024)
摘要
AbstractIntroduction/BackgroundGenetic variants beyond FDA-approved drug targets are often identified in non-small cell lung cancer (NSCLC) patients. Although the performances ofin silicotools in predicting variant pathogenicity have been analyzed in previous studies, they have not been analyzed for actionable targets of FDA-approved therapies for NSCLC. The aim of this study is to compare the performance of commonly usedin silicotools in classifying the pathogenicity of actionable variants in NSCLC.Materials and MethodsWe evaluated the performance of the followingin silicotools: Polyphen-2 (HumDiv, HumVar), Align-GVGD, MutationTaster2021, CADD, CONDEL, and REVEL. A curated set of targetable NSCLC missense variants (n=236) was used. The overall accuracy, sensitivity, specificity, positive predictive value (PPV), negative predictive value (NPV), and Matthews correlation coefficient (MCC) of eachin silicotool was determined.ResultsThe most recently released MutationTaster2021 demonstrated the highest performance in terms of accuracy, specificity, PPV, and MCC, but was outperformed by CADD for both sensitivity and NPV. Although some tools demonstrated high sensitivities, all tools except MutationTaster2021 displayed markedly low overall specificities, as low as 23%.ConclusionThe collective results indicate that the evaluatedin silicotools can provide guidance in predicting the pathogenicity of NSCLC missense variants, but are not fully reliable. The tools analyzed in this study could be acceptable to rule out pathogenicity in variants given their higher sensitivities, but are limited when it comes to identifying pathogenicity in variants due to low specificities.HighlightsMutationTaster2021 demonstrated the highest overall performanceCADD demonstrated the highest sensitivity (99.19%) and NPV (96.55%).All tools but MutationTaster2021 demonstrated low specificities, as low as 23%An individual predictor outperformed 3 meta predictorsPerformance variability suggests caution when usingin silicotools in the clinic
更多查看译文
AI 理解论文
溯源树
样例
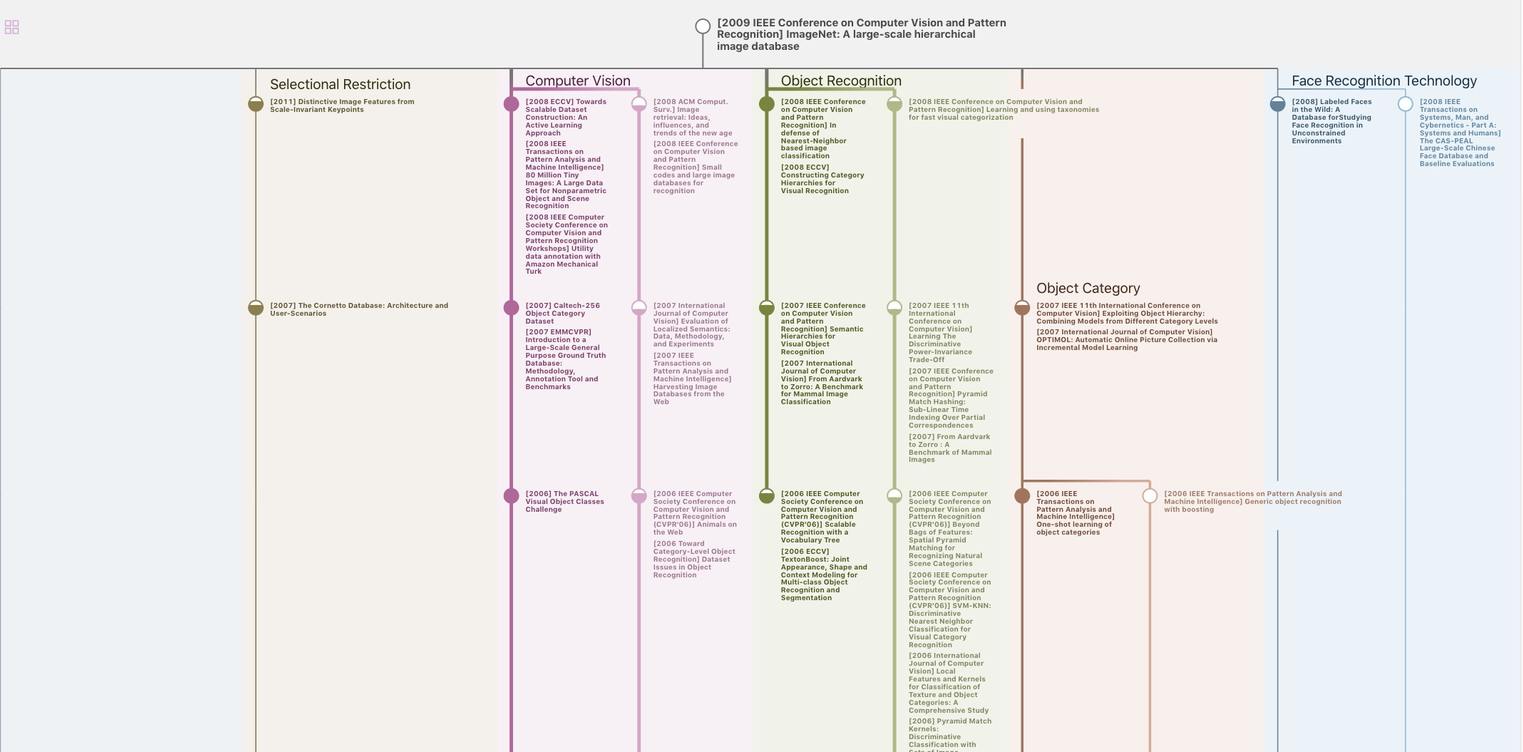
生成溯源树,研究论文发展脉络
Chat Paper
正在生成论文摘要