Eigen-Entropy based Time Series Signatures to Support Multivariate Time Series Classification
crossref(2024)
摘要
Abstract Most current algorithms for multivariate time series classification tend to overlook the correlations between time series of different variables. In this research, we propose a framework that leverages Eigen-entropy along with a cumulative moving window to derive time series signatures to support the classification task. These signatures are enumerations of correlations among different time series considering the temporal nature of the dataset. To manage dataset's dynamic nature, we employ preprocessing with dense multi scale entropy. Consequently, the proposed framework, Eigen-entropy-based Time Series Signatures, captures correlations among multivariate time series without losing its temporal and dynamic aspects. The efficacy of our algorithm is assessed using six binary datasets sourced from the University of East Anglia (UEA), in addition to a publicly available sepsis dataset and an institutional sepsis dataset from the Mayo Clinic. We employ recall as a metric for evaluation against the baseline algorithm, Dependent Dynamic Time Warping with 1 Nearest Neighbor. Our algorithm outperforms the baseline algorithm for UEA datasets in terms of recall with an average value of 10% across all datasets, and by 38% for an institutional sepsis dataset. For the public sepsis dataset, the performance of our algorithm is comparable to the baseline algorithm.
更多查看译文
AI 理解论文
溯源树
样例
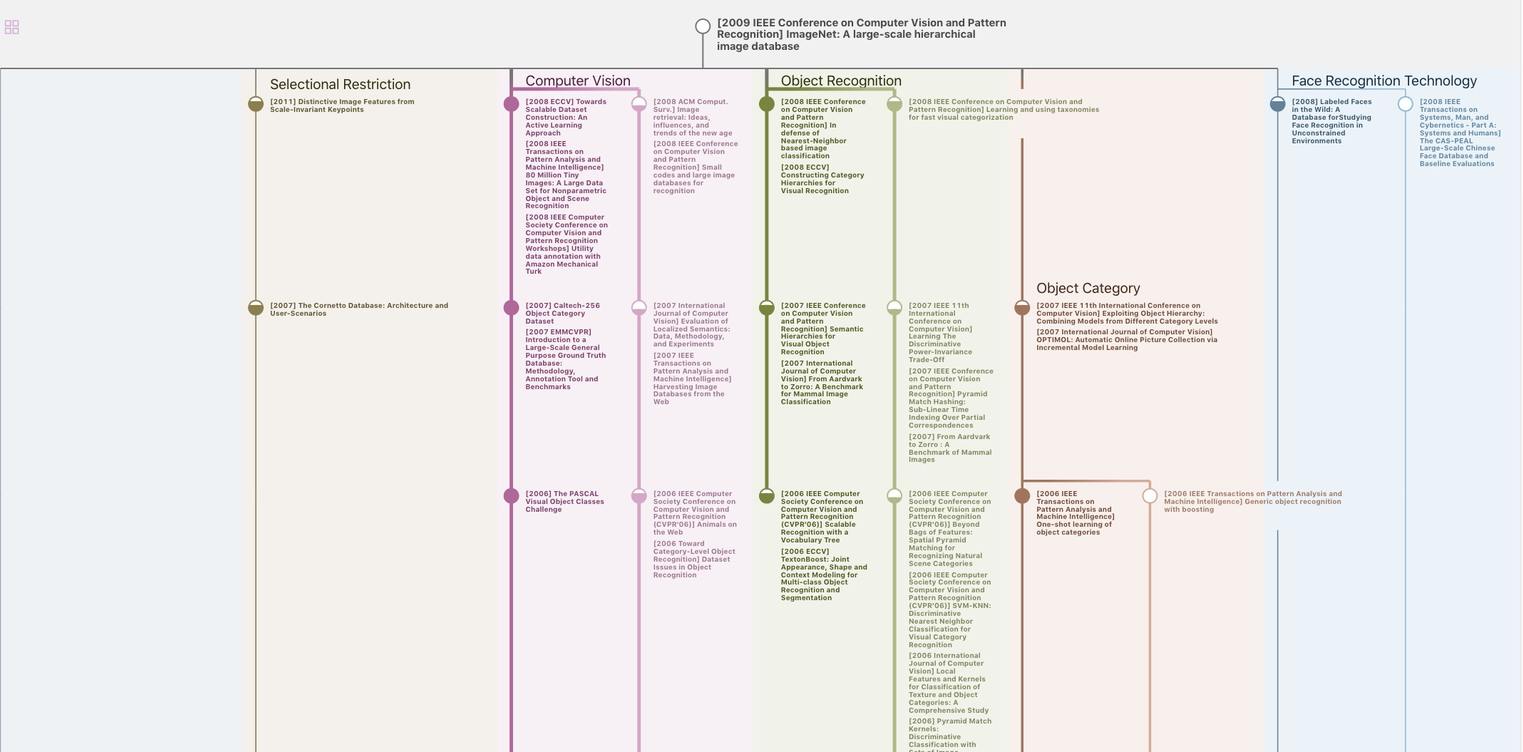
生成溯源树,研究论文发展脉络
Chat Paper
正在生成论文摘要