Training data in satellite image classification for land cover mapping: a review
EUROPEAN JOURNAL OF REMOTE SENSING(2024)
摘要
The current land cover (LC) mapping paradigm relies on automatic satellite imagery classification, predominantly through supervised methods, which depend on training data to calibrate classification algorithms. Hence, training data have a critical influence on classification accuracy. Although research on specific aspects of training data in the LC classification context exists, a study that organizes and synthetizes the multiplicity of aspects and findings of these researches is needed. In this article, we review the training data used for LC classification of satellite imagery. A protocol of identification and selection of relevant documents was followed, resulting in 114 peer-reviewed studies included. Main research topics were identified and documents were characterized according to their contribution to each topic, which allowed uncovering subtopics and categories and synthetizing the main findings regarding different aspects of the training dataset. The analysis found four research topics, namely construction of the training dataset, sample quality, sampling design and advanced learning techniques. Subtopics included sample collection method, sample cleaning procedures, sample size, sampling method, class balance and distribution, among others. A summary of the main findings and approaches provided an overview of the research in this area, which may serve as a starting point for new LC mapping initiatives.
更多查看译文
关键词
Land cover,satellite images,supervised classification,training data,sampling design,sample quality
AI 理解论文
溯源树
样例
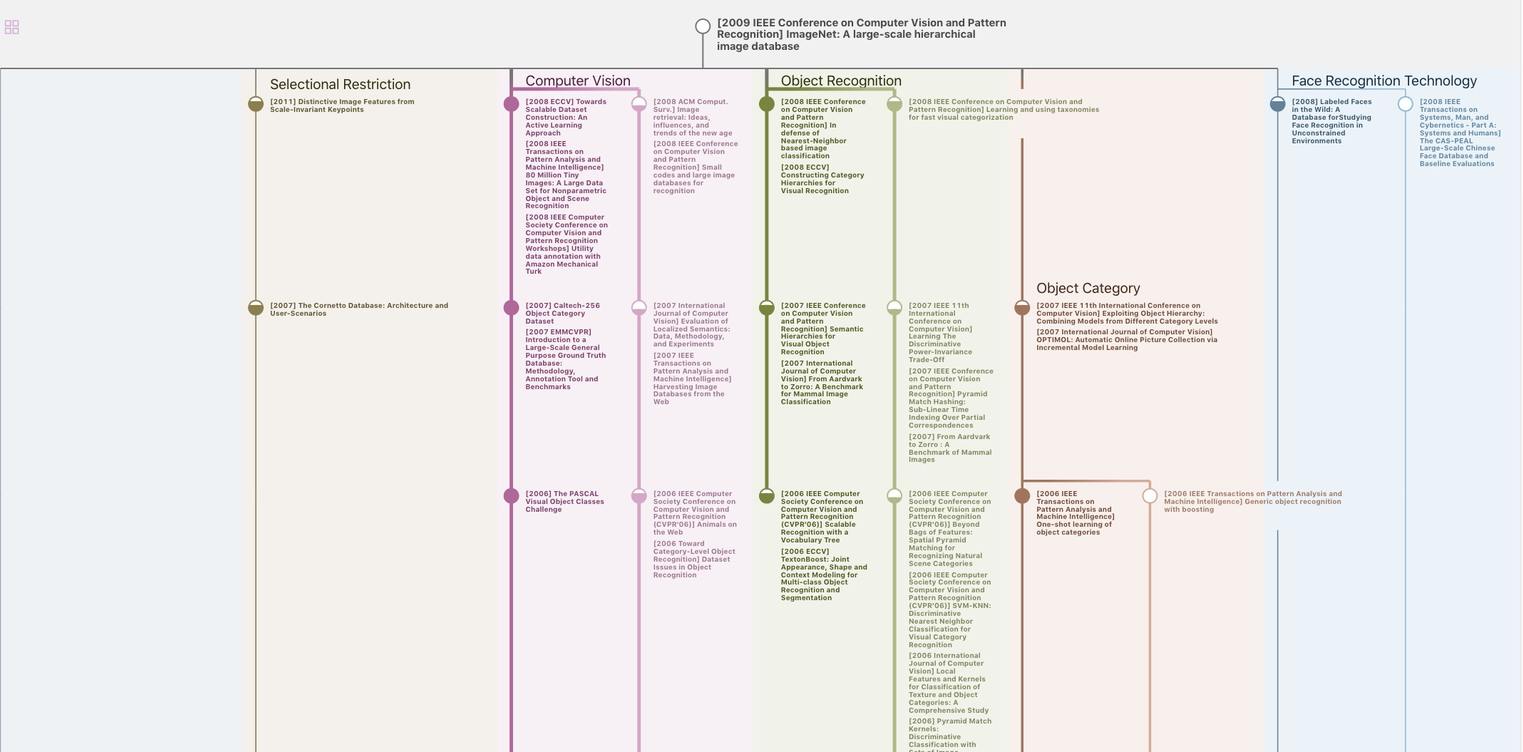
生成溯源树,研究论文发展脉络
Chat Paper
正在生成论文摘要