A deep learning pipeline for time-lapse camera monitoring of floral environments and insect populations
biorxiv(2024)
摘要
Insects play pivotal roles in various fields, prompting the integration of cameras and computer vision to develop novel monitoring approaches in agriculture, ecology, evolution and biodiversity monitoring. This paper presents a comprehensive methodology to monitor insects in the wild and to quantify floral cover as a key resource affecting the abundance and diversity of insects. We apply the methods across more than 10 million images recorded over two years using 48 insect camera traps placed in three main habitat types. The proposed deep-learning pipeline estimates flower cover and detects and classifies arthropod taxa from time-lapse recordings. Semantic and color segmentation are combined to estimate the percent cover of flowers of different colors. Arthropod including insect detection incorporates motion-informed enhanced images and object detection, followed by filtering stationary objects to minimize double counting of non-moving animals and erroneous plant detections. This filtering approach has been demonstrated to significantly decrease the incidence of false positives, particularly in the case of arthropods, occurring in only 3% of the captured images. The final step involves grouping arthropods into 19 taxonomic classes. The classification model achieved an 80% classification average precision. As expected during the beginning and end of the season, reduced flower cover correlates with a noticeable lack of insects.
The artificial intelligence pipeline utilizes DeepLabv3 for semantic segmentation, You-Only-Look-Once (YOLOv5) for object detection, and EfficientNet for classification. This proposed engineering method offers a cost-effective approach to monitoring flower cover and penology and diverse arthropod taxa in natural environments using time-lapse camera recordings.
### Competing Interest Statement
The authors have declared no competing interest.
更多查看译文
AI 理解论文
溯源树
样例
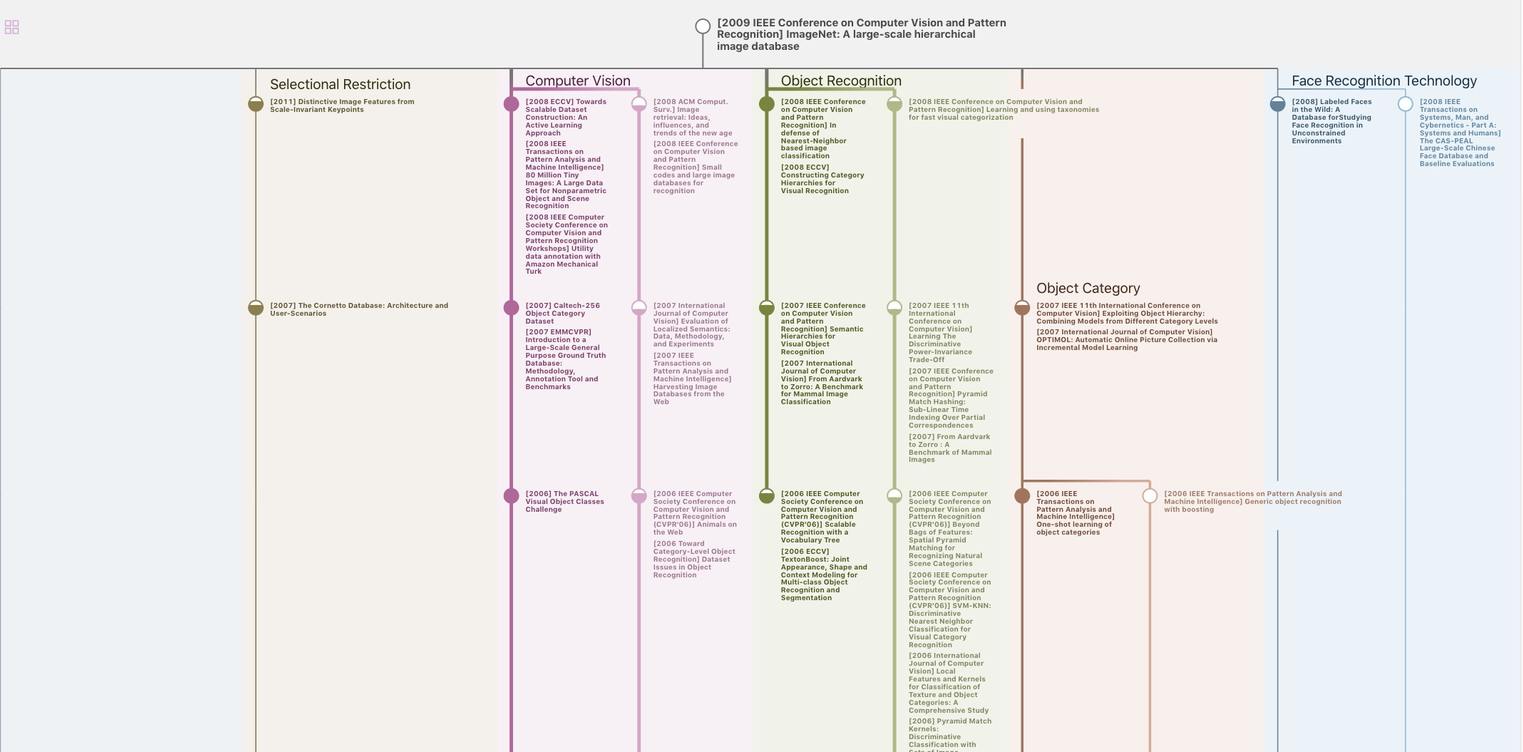
生成溯源树,研究论文发展脉络
Chat Paper
正在生成论文摘要