On the use of neutron flux gradient with ANNs for the detection of diverted spent nuclear fuel
Annals of Nuclear Energy(2024)
摘要
One of the main tasks in nuclear safeguards is regular inspections of Spent Nuclear Fuel (SNF) assemblies to detect possible diversions of special nuclear material such as 235U and 239Pu. In these inspections, characteristic signatures of SNF such as emissions of neutrons and gamma rays from the radioactive decay, are measured and their consistency with the declared assemblies is verified to ensure that no fuel pins have been removed. Research in this field is focused on both the development of detection equipment and methods for the analysis of the acquired measurement data. In this paper, the use of the neutron flux gradient, which is not considered in regular SNF verification, is investigated in combination with the scalar neutron flux as input to artificial neural network models for the quantification of fuel pins in SNF assemblies. The training and testing of these ANN models rely on a synthetic dataset that is generated from Monte Carlo simulations of a typical intact pressurized water reactor assembly and with different patterns of fuel pins replaced by dummy pins. The dataset consists of unique scenarios so that the ANN can be assessed over “unknown” cases that are not part of the learning phase. Results show that the neutron flux gradient is advantageous for a more accurate reconstruction of diversions within SNF assemblies.
更多查看译文
关键词
Neutron flux gradient,Artificial neural networks,Spent nuclear fuel,Nuclear safeguards,Monte Carlo
AI 理解论文
溯源树
样例
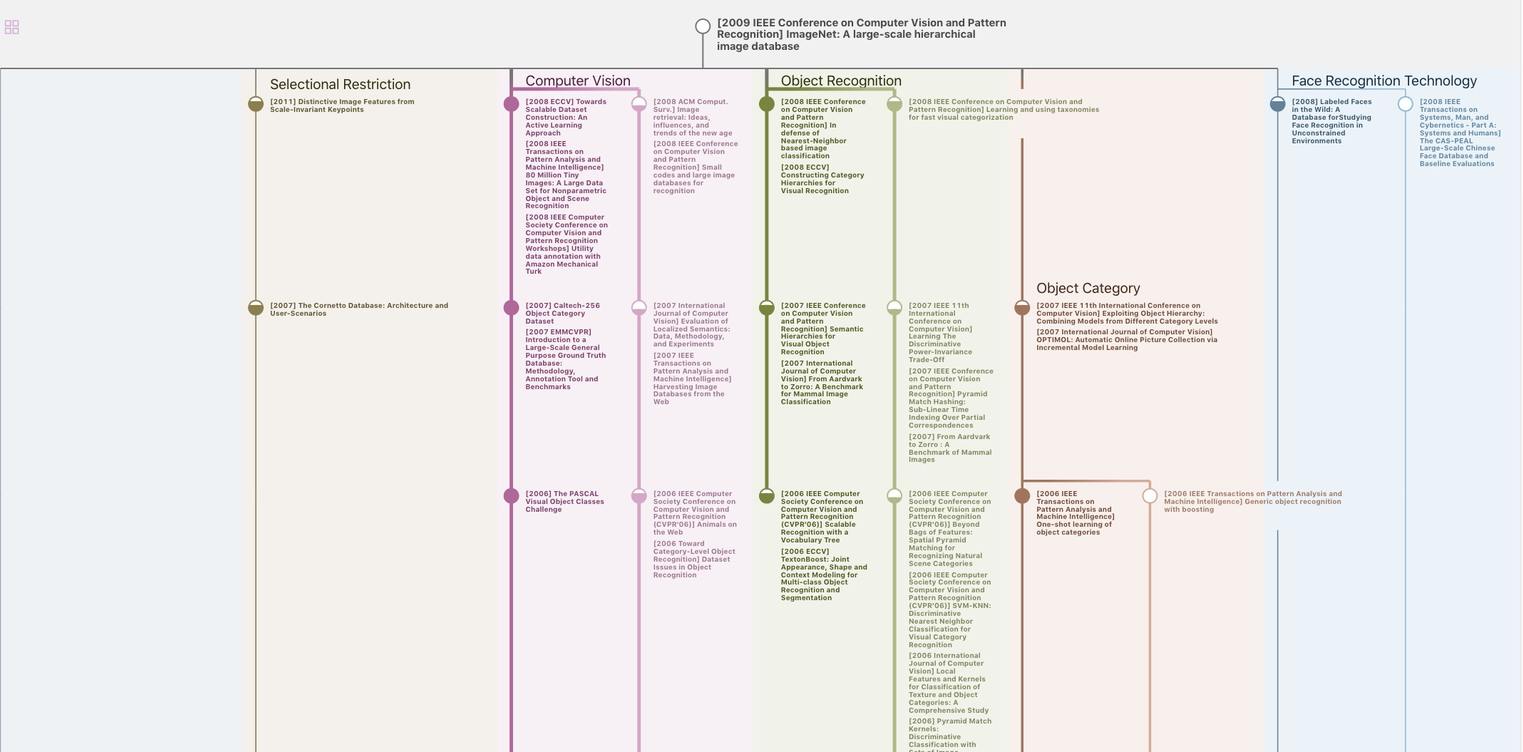
生成溯源树,研究论文发展脉络
Chat Paper
正在生成论文摘要